Learning Multiple Tasks with Boosted Decision Trees.
ECML/PKDD (1)(2012)
摘要
We address the problem of multi-task learning with no label correspondence among tasks. Learning multiple related tasks simultaneously, by exploiting their shared knowledge can improve the predictive performance on every task. We develop the multi-task Adaboost environment with Multi-Task Decision Trees as weak classifiers. We first adapt the well known decision tree learning to the multi-task setting. We revise the information gain rule for learning decision trees in the multi-task setting. We use this feature to develop a novel criterion for learning Multi-Task Decision Trees. The criterion guides the tree construction by learning the decision rules from data of different tasks, and representing different degrees of task relatedness. We then modify MT-Adaboost to combine Multi-task Decision Trees as weak learners. We experimentally validate the advantage of the new technique; we report results of experiments conducted on several multi-task datasets, including the Enron email set and Spam Filtering collection.
更多查看译文
关键词
Multi-Task Decision Trees,multi-task setting,decision tree,multi-task learning,multi-task Adaboost environment,multi-task datasets,decision rule,criterion guide,different degree,different task,multiple task
AI 理解论文
溯源树
样例
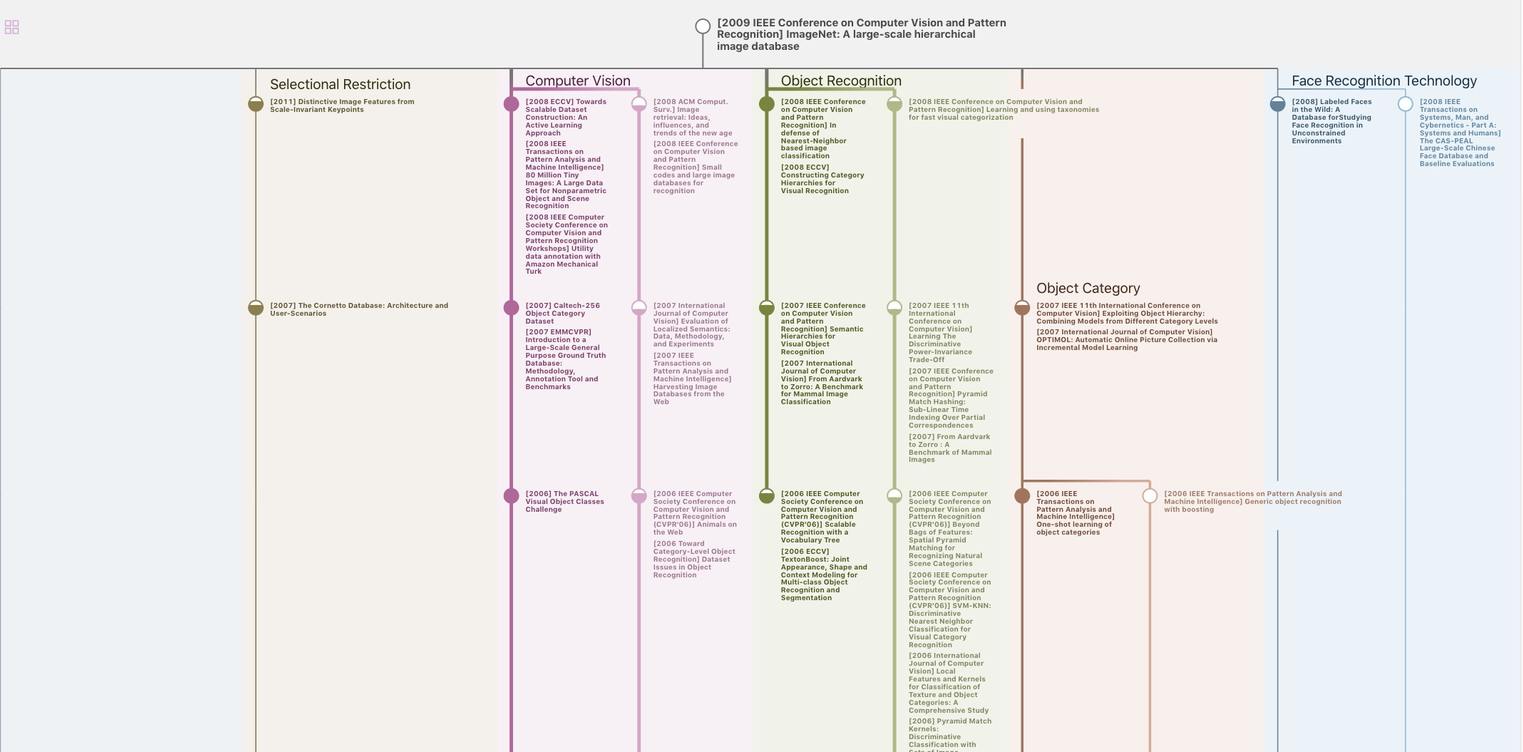
生成溯源树,研究论文发展脉络
Chat Paper
正在生成论文摘要