Approximate posterior distributions for convolutional two-level hidden Markov models
Computational Statistics & Data Analysis(2013)
Abstract
A convolutional two-level hidden Markov model is defined and evaluated. The bottom level contains an unobserved categorical Markov chain, and given the variables in this level the middle level contains unobserved conditionally independent Gaussian variables. The top level contains observable variables that are a convolution of the variables in the middle level plus additive Gaussian errors. The objective is to assess the categorical variables in the bottom level given the convolved observations in the top level. The inversion is cast in a Bayesian setting with a Markov chain prior model and convolved Gaussian likelihood model. The associated posterior model cannot be assessed since the normalizing constant is too computer demanding to calculate for realistic problems. Three approximate posterior models based on approximations of the likelihood model on generalized factorial form are defined. These approximations can be exactly assessed by the forward-backward algorithm. Both a synthetic case and a real seismic inversion case are used in an empirical evaluation. It is concluded that reliable and computationally efficient approximate posterior models for convolutional two-level hidden Markov models can be defined.
MoreTranslated text
Key words
likelihood model,markov model,associated posterior model,approximate posterior distribution,convolved gaussian likelihood model,convolutional two-level,approximate posterior model,prior model,top level,bottom level,middle level,convolution,seismic inversion,approximation,hidden markov model,forward backward algorithm
AI Read Science
Must-Reading Tree
Example
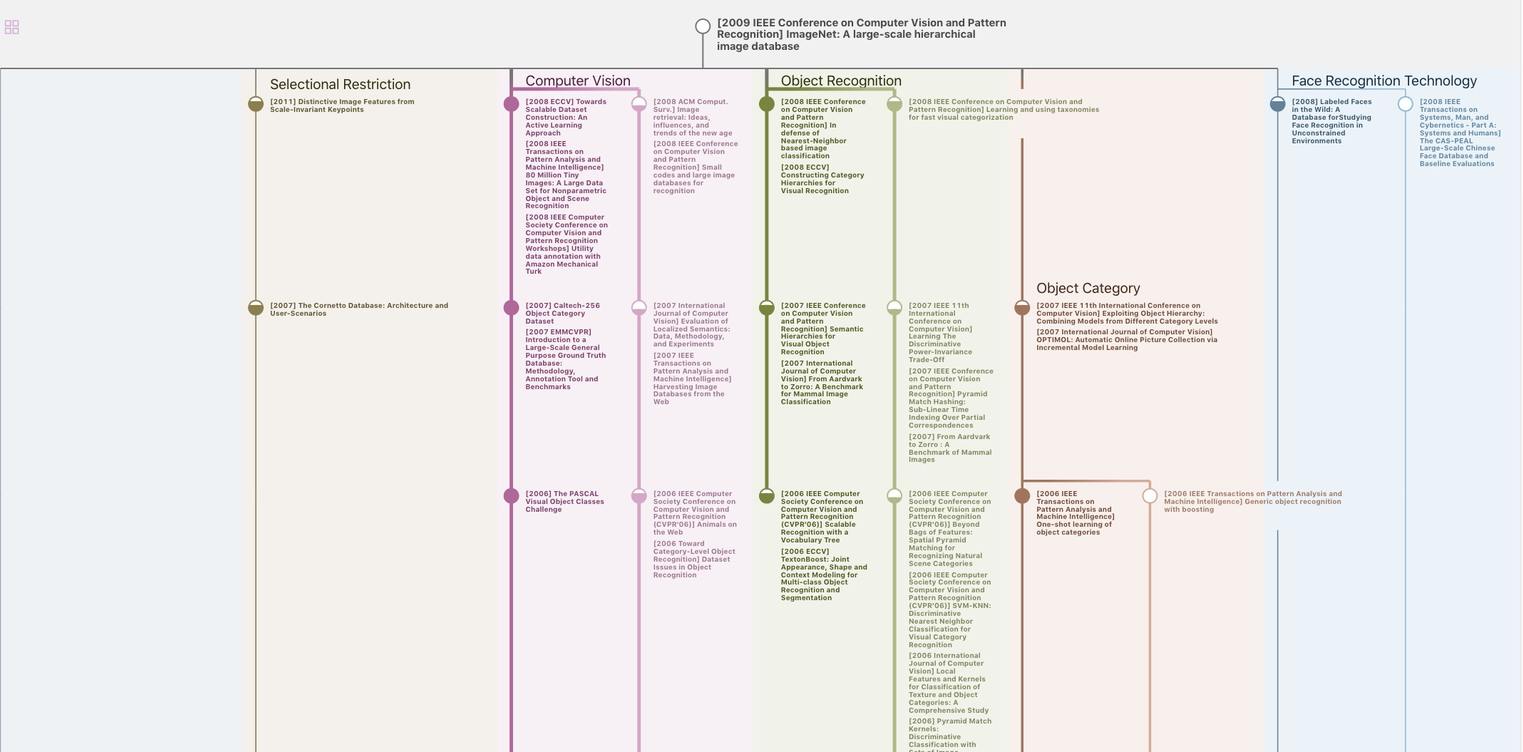
Generate MRT to find the research sequence of this paper
Chat Paper
Summary is being generated by the instructions you defined