Sequential estimation of mixtures of structured autoregressive models
Computational Statistics & Data Analysis(2013)
摘要
A class of mixtures of structured autoregressive (AR) models and methods for sequential estimation within this class of models are considered. Such models and methods are motivated by the analysis of electroencephalogram (EEG) signals recorded during a cognitive fatigue experiment. Specifically, an electroencephalogram recorded from a subject who performed continuous mental arithmetic for 180 min is studied. The EEG signal is modeled via mixtures of autoregressive processes with structured prior distributions on the reciprocal roots of the characteristic AR polynomials. The use of structured prior distributions on the AR mixture components allows researchers to include scientifically meaningful information related to various states of mental alertness. On-line posterior estimation of the model parameters and related quantities is achieved using a sequential Monte Carlo algorithm. The performance of such algorithm is illustrated by applying it to simulated data and EEG data. The EEG analyses show that the mixtures of structured AR models successfully identify EEG features that may be associated with states of mental fatigue. Furthermore, one of the key features of the proposed methods is that they can be implemented in real time, allowing for automatic characterization of mental fatigue from EEG recordings.
更多查看译文
关键词
ar mixture component,eeg feature,structured priors,on-line estimation,structured prior distribution,eeg recording,sequential estimation,eeg analyses show,continuous mental arithmetic,mixture of autoregressions,eeg data,eeg signal,sequential monte carlo,characteristic ar polynomial,mental fatigue,structured autoregressive model
AI 理解论文
溯源树
样例
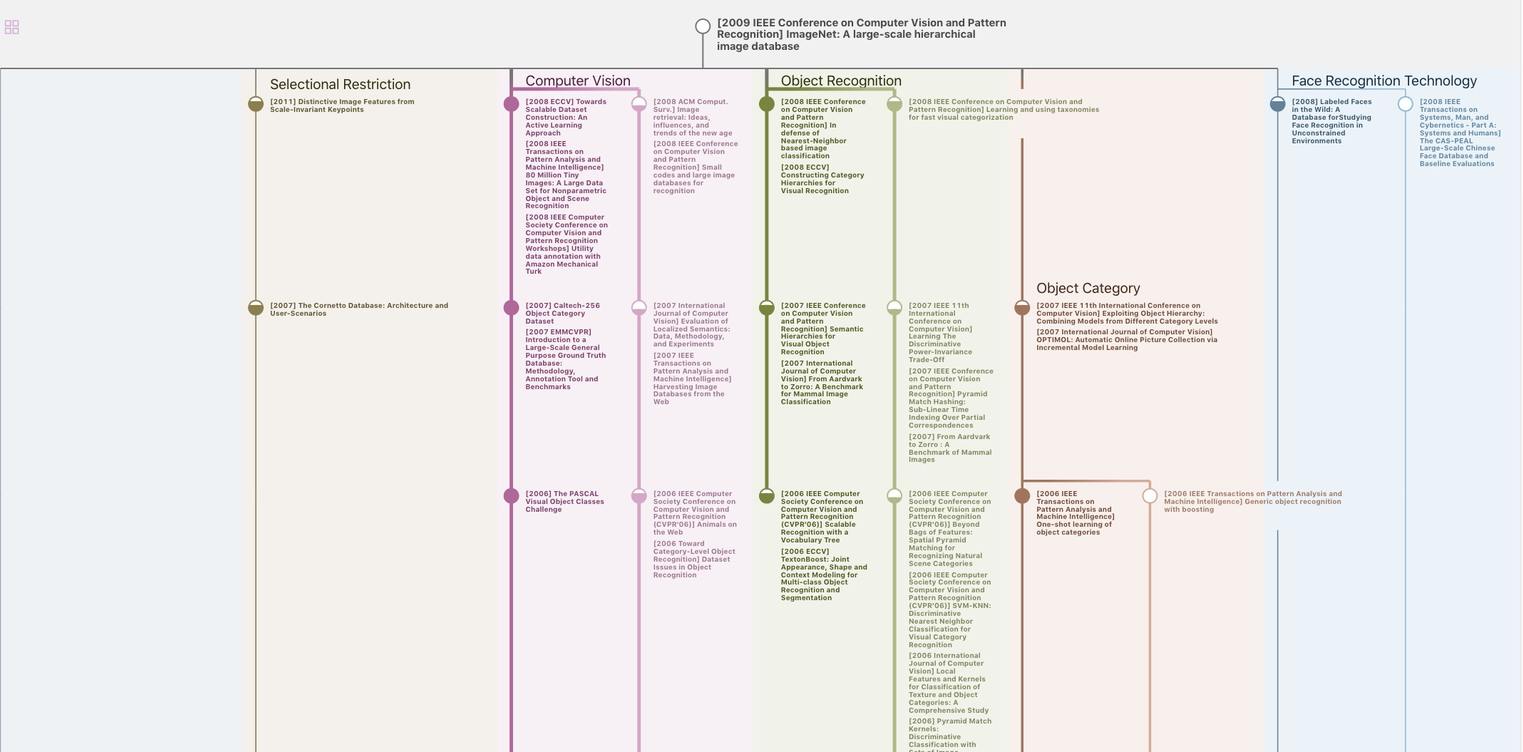
生成溯源树,研究论文发展脉络
Chat Paper
正在生成论文摘要