Unsupervised Learning on an Approximate Corpus.
NAACL HLT '12 Proceedings of the 2012 Conference of the North American Chapter of the Association for Computational Linguistics: Human Language Technologies(2012)
摘要
Unsupervised learning techniques can take advantage of large amounts of unannotated text, but the largest text corpus (the Web) is not easy to use in its full form. Instead, we have statistics about this corpus in the form of n -gram counts (Brants and Franz, 2006). While n -gram counts do not directly provide sentences, a distribution over sentences can be estimated from them in the same way that n -gram language models are estimated. We treat this distribution over sentences as an approximate corpus and show how unsupervised learning can be performed on such a corpus using variational inference. We compare hidden Markov model (HMM) training on exact and approximate corpora of various sizes, measuring speed and accuracy on unsupervised part-of-speech tagging.
更多查看译文
关键词
approximate corpus,n-gram count,largest text corpus,n-gram language model,Unsupervised learning technique,full form,unannotated text,unsupervised learning,unsupervised part-of-speech tagging,Markov model
AI 理解论文
溯源树
样例
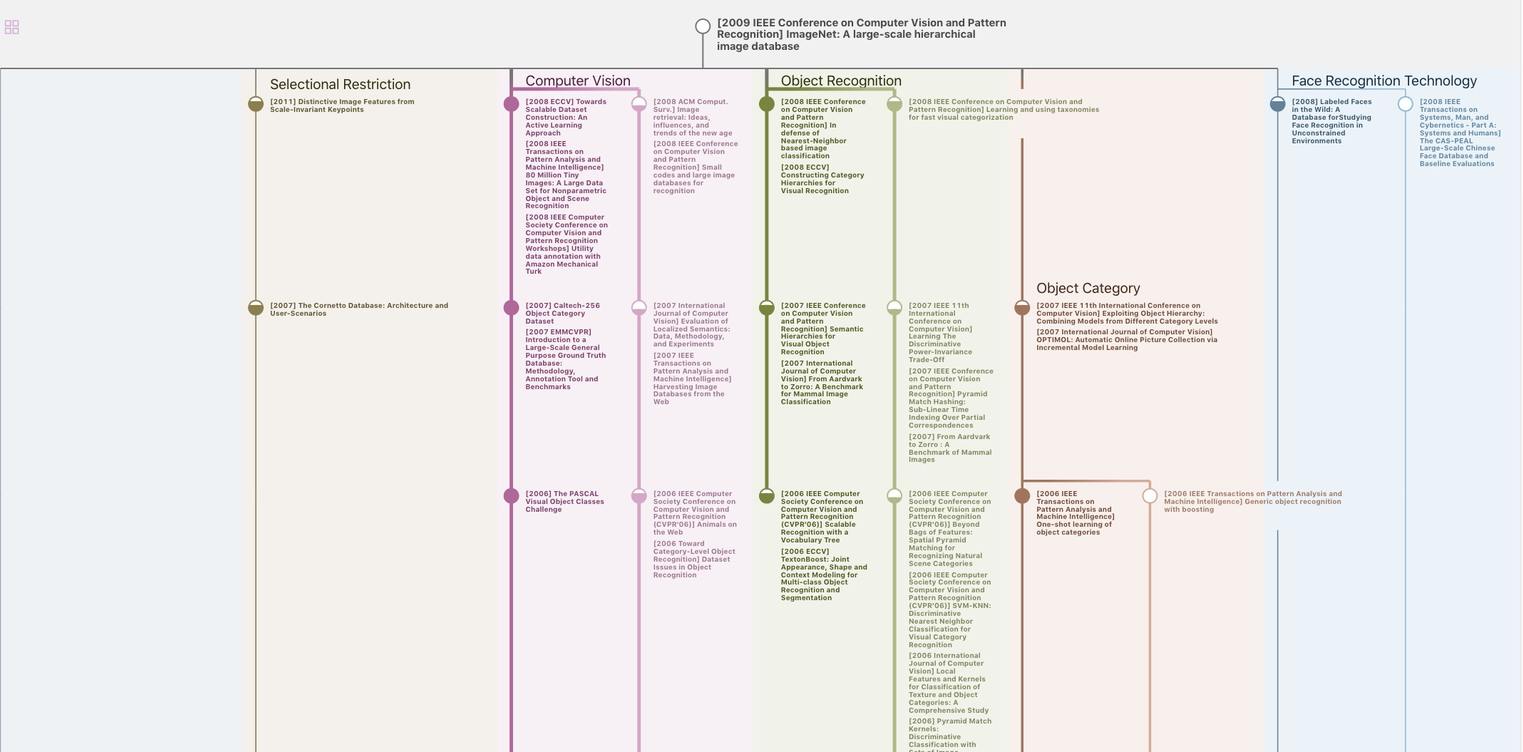
生成溯源树,研究论文发展脉络
Chat Paper
正在生成论文摘要