Feature seeding for action recognition
Computer Vision(2011)
摘要
Progress in action recognition has been in large part due to advances in the features that drive learning-based methods. However, the relative sparsity of training data and the risk of overfitting have made it difficult to directly search for good features. In this paper we suggest using synthetic data to search for robust features that can more easily take advantage of limited data, rather than using the synthetic data directly as a substitute for real data. We demonstrate that the features discovered by our selection method, which we call seeding, improve performance on an action classification task on real data, even though the synthetic data from which the features are seeded differs significantly from the real data, both in terms of appearance and the set of action classes.
更多查看译文
关键词
good feature,training data,learning-based method,large part,action class,action recognition,limited data,synthetic data,action classification task,histograms,image classification,trajectory,support vector machines,learning artificial intelligence,feature extraction,support vector machine
AI 理解论文
溯源树
样例
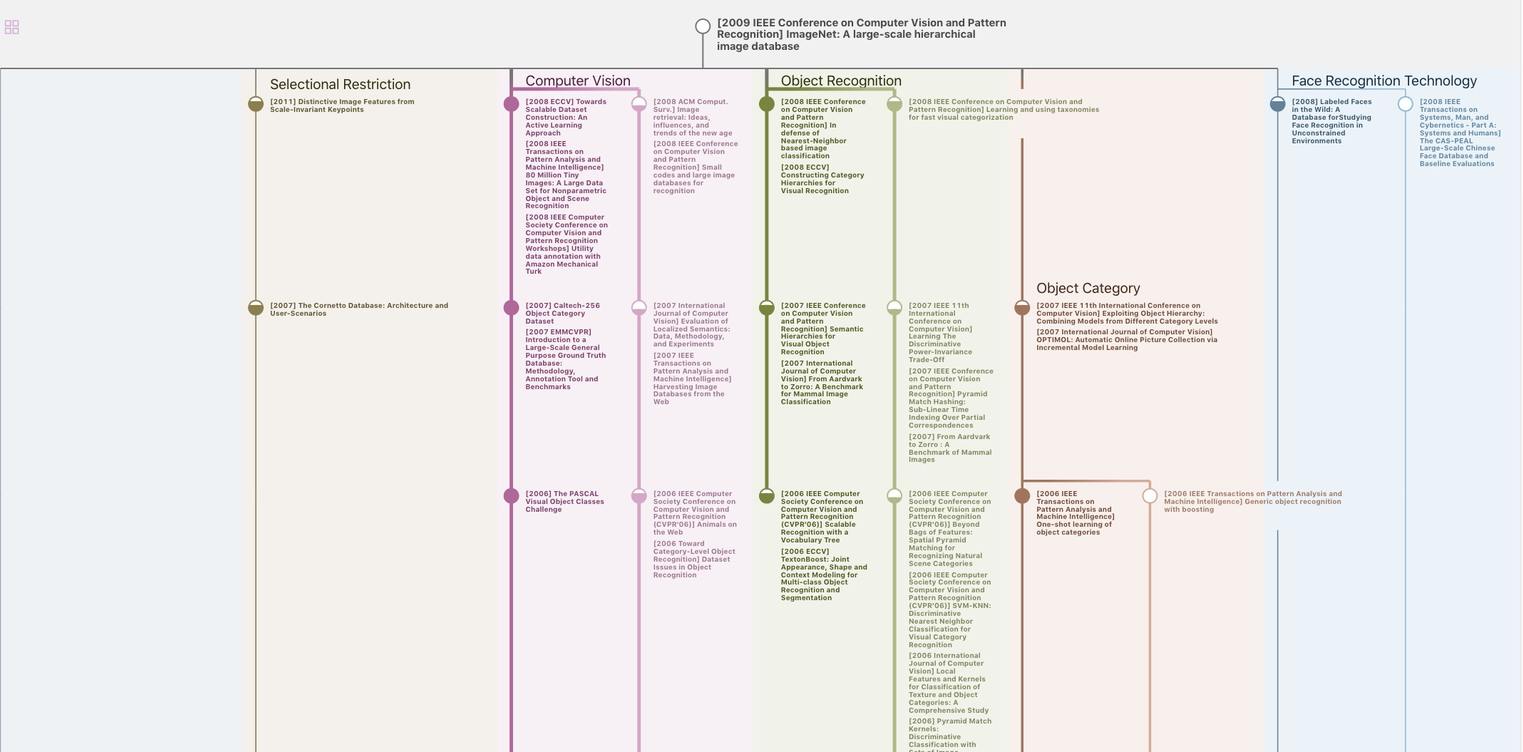
生成溯源树,研究论文发展脉络
Chat Paper
正在生成论文摘要