A Cluster-Validity Index Combining An Overlap Measure And A Separation Measure Based On Fuzzy-Aggregation Operators
IEEE TRANSACTIONS ON FUZZY SYSTEMS(2011)
Abstract
Since a clustering algorithm can produce as many partitions as desired, one needs to assess their quality in order to select the partition that most represents the structure in the data, if there is any. This is the rationale for the cluster-validity (CV) problem and indices. This paper presents a CV index that helps to find the optimal number of clusters of data from partitions generated by a fuzzy-clustering algorithm, such as the fuzzy c-means (FCM) or its derivatives. Given a fuzzy partition, this new index uses a measure of multiple cluster overlap and a separation measure for each data point, both based on an aggregation operation of membership degrees. Experimental results on artificial and benchmark datasets are given to demonstrate the performance of the proposed index, as compared with traditional and recent indices.
MoreTranslated text
Key words
Aggregation operators (AOs),cluster validity (CV),fuzzy-cluster analysis,triangular norms (t-norms)
AI Read Science
Must-Reading Tree
Example
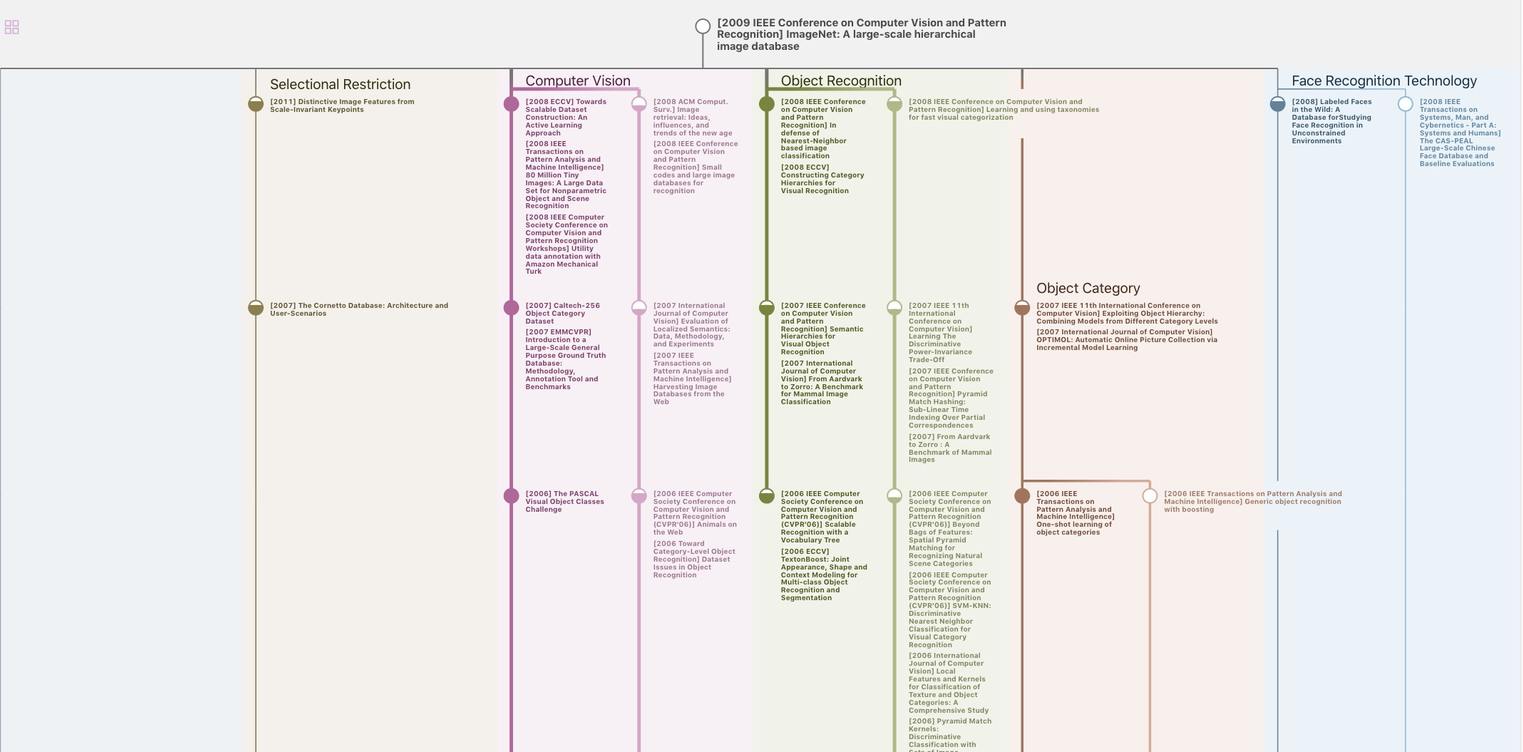
Generate MRT to find the research sequence of this paper
Chat Paper
Summary is being generated by the instructions you defined