Computing Steerable Principal Components of a Large Set of Images and Their Rotations
IEEE Transactions on Image Processing(2011)
摘要
We present here an efficient algorithm to compute the Principal Component Analysis (PCA) of a large image set consisting of images and, for each image, the set of its uniform rotations in the plane. We do this by pointing out the block circulant structure of the covariance matrix and utilizing that structure to compute its eigenvectors. We also demonstrate the advantages of this algorithm over similar ones with numerical experiments. Although it is useful in many settings, we illustrate the specific application of the algorithm to the problem of cryo-electron microscopy.
更多查看译文
关键词
covariance matrices,eigenvalues and eigenfunctions,image processing,principal component analysis,block circulant structure,covariance matrix,cryo-electron microscopy,eigenvectors,large image set,steerable principal component analysis,EDICS Category: TEC-PRC image and video processing techniques
AI 理解论文
溯源树
样例
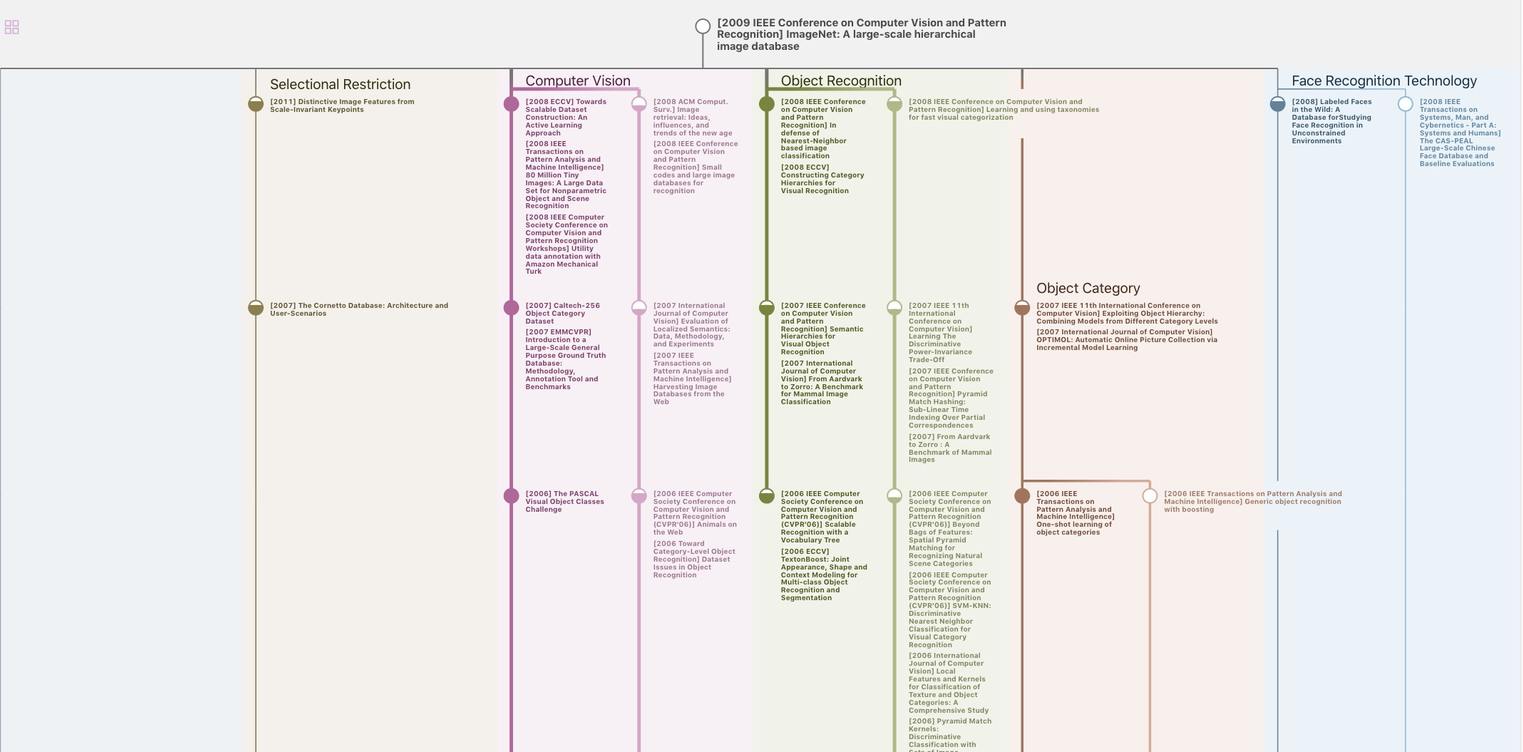
生成溯源树,研究论文发展脉络
Chat Paper
正在生成论文摘要