Orthonormal Expansion $\ell_{1}$-Minimization Algorithms for Compressed Sensing
IEEE Transactions on Signal Processing(2011)
摘要
Compressed sensing aims at reconstructing sparse signals from significantly reduced number of samples, and a popular reconstruction approach is $\ell_{1}$-norm minimization. In this correspondence, a method called orthonormal expansion is presented to reformulate the basis pursuit problem for noiseless compressed sensing. Two algorithms are proposed based on convex optimization: one exactly solves the problem and the other is a relaxed version of the first one. The latter can be considered as a modified iterative soft thresholding algorithm and is easy to implement. Numerical simulation shows that, in dealing with noise-free measurements of sparse signals, the relaxed version is accurate, fast and competitive to the recent state-of-the-art algorithms. Its practical application is demonstrated in a more general case where signals of interest are approximately sparse and measurements are contaminated with noise.
更多查看译文
关键词
convex optimization,algorithm design,noise measurement,phase transition,convex programming,basis pursuit,compressed sensing,minimisation,signal reconstruction,iterative methods,indexing terms,lagrange multiplier,numerical simulation,data compression
AI 理解论文
溯源树
样例
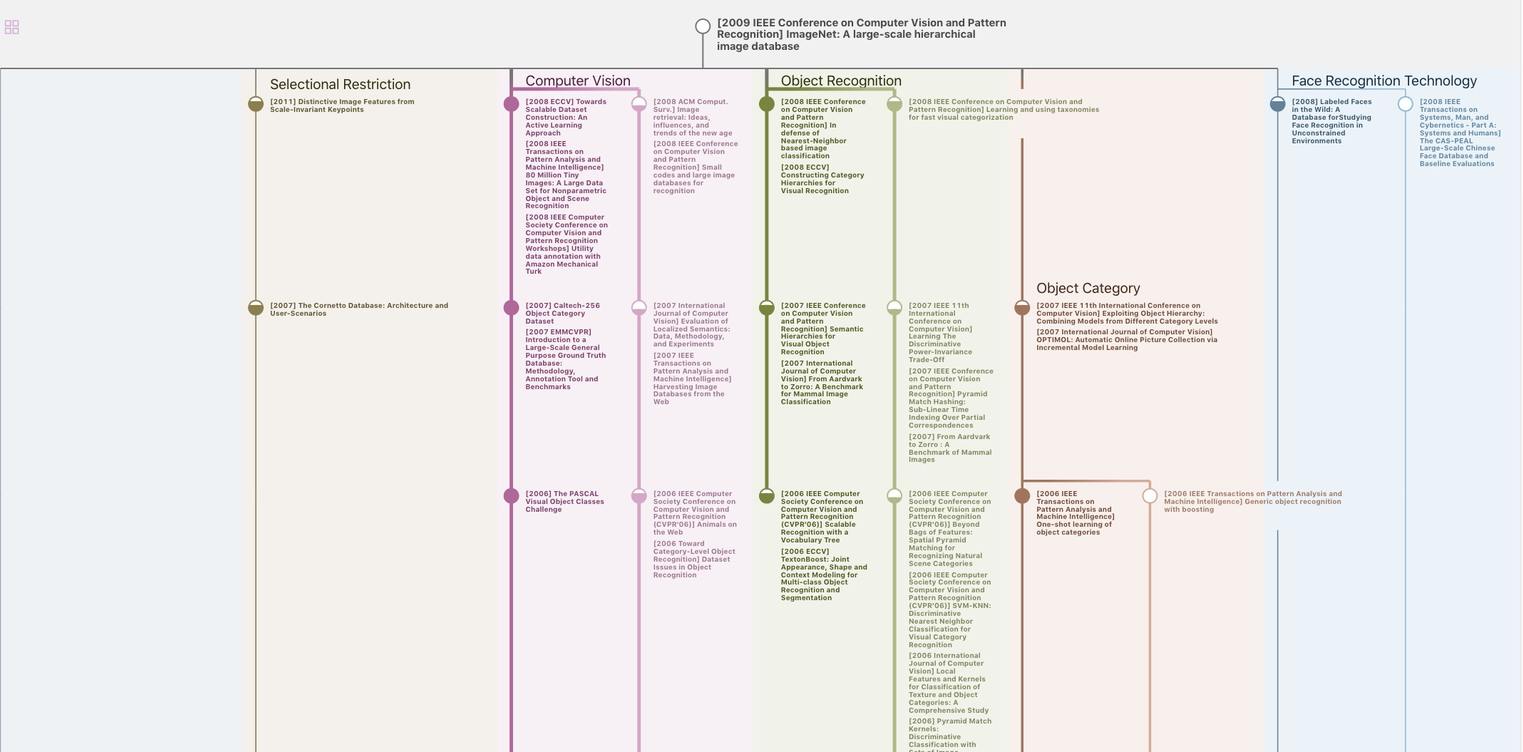
生成溯源树,研究论文发展脉络
Chat Paper
正在生成论文摘要