Clustering Based on Pairwise Distances When the Data is of Mixed Dimensions
IEEE Transactions on Information Theory(2011)
摘要
In the context of clustering, we consider a generative model in a Euclidean ambient space with clusters of different shapes, dimensions, sizes, and densities. In an asymptotic setting where the number of points becomes large, we obtain theoretical guaranties for some emblematic methods based on pairwise distances: a simple algorithm based on the extraction of connected components in a neighborhood graph; hierarchical clustering with single linkage; and the spectral clustering method of Ng, Jordan, and Weiss. The methods are shown to enjoy some near-optimal properties in terms of separation between clusters and robustness to outliers. The local scaling method of Zelnik-Manor and Perona is shown to lead to a near-optimal choice for the scale in the first and third methods. We also provide a lower bound on the spectral gap to consistently choose the correct number of clusters in the spectral method.
更多查看译文
关键词
euclidean ambient space,and phrases. clustering,spectral methods,nearest-neighbor search.,minimax rates,spectral method,mixed dimensions,random geometric graphs,hierarchical clustering,spectral gap,correct number,manifold learning,detection in point clouds,extracting connected com- ponents,spectral clustering method,local scaling method,hierarchical clustering with single linkage,neighborhood graphs,near-optimal choice,near-optimal property,emblematic method,manifolds,random geometric graph,connected component,single linkage,clustering algorithms,graph theory,lower bound,clustering,kernel,point cloud,spectral clustering,couplings,nearest neighbor search
AI 理解论文
溯源树
样例
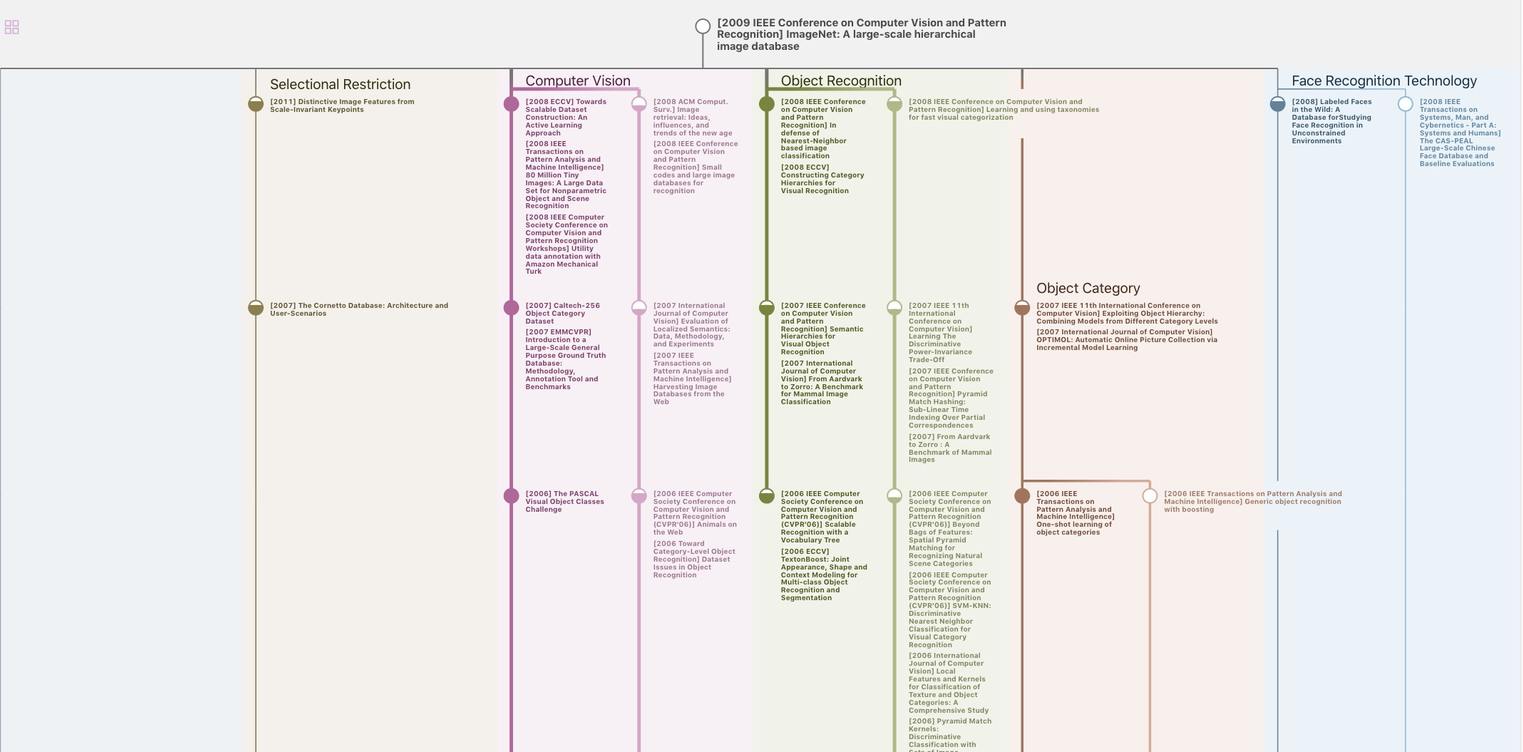
生成溯源树,研究论文发展脉络
Chat Paper
正在生成论文摘要