A minority class feature selection method
PROGRESS IN PATTERN RECOGNITION, IMAGE ANALYSIS, COMPUTER VISION, AND APPLICATIONS(2011)
摘要
In many classification problems, and in particular in medical domains, it is common to have an unbalanced class distribution. This pose problems to classifiers as they tend to perform poorly in the minority class which is often the class of interest. One commonly used strategy that to improve the classification performance is to select a subset of relevant features. Feature selection algorithms, however, have not been designed to favour the classification performance of the minority class. In this paper, we present a novel filter feature selection algorithm, called FSMC, for unbalanced data sets. FSMC selects attributes that have minority class distributions significantly different from the majority class distributions. FSMC is fast, simple, selects a small number of features and outperforms in most cases other feature selection algorithms in terms of global accuracy and in terms of performance measures for the minority class such as precision, recall, F-measure and ROC values.
更多查看译文
关键词
relevant feature,minority class,unbalanced class distribution,minority class feature selection,feature selection algorithm,novel filter feature selection,minority class distribution,performance measure,classification problem,majority class distribution,classification performance,feature selection
AI 理解论文
溯源树
样例
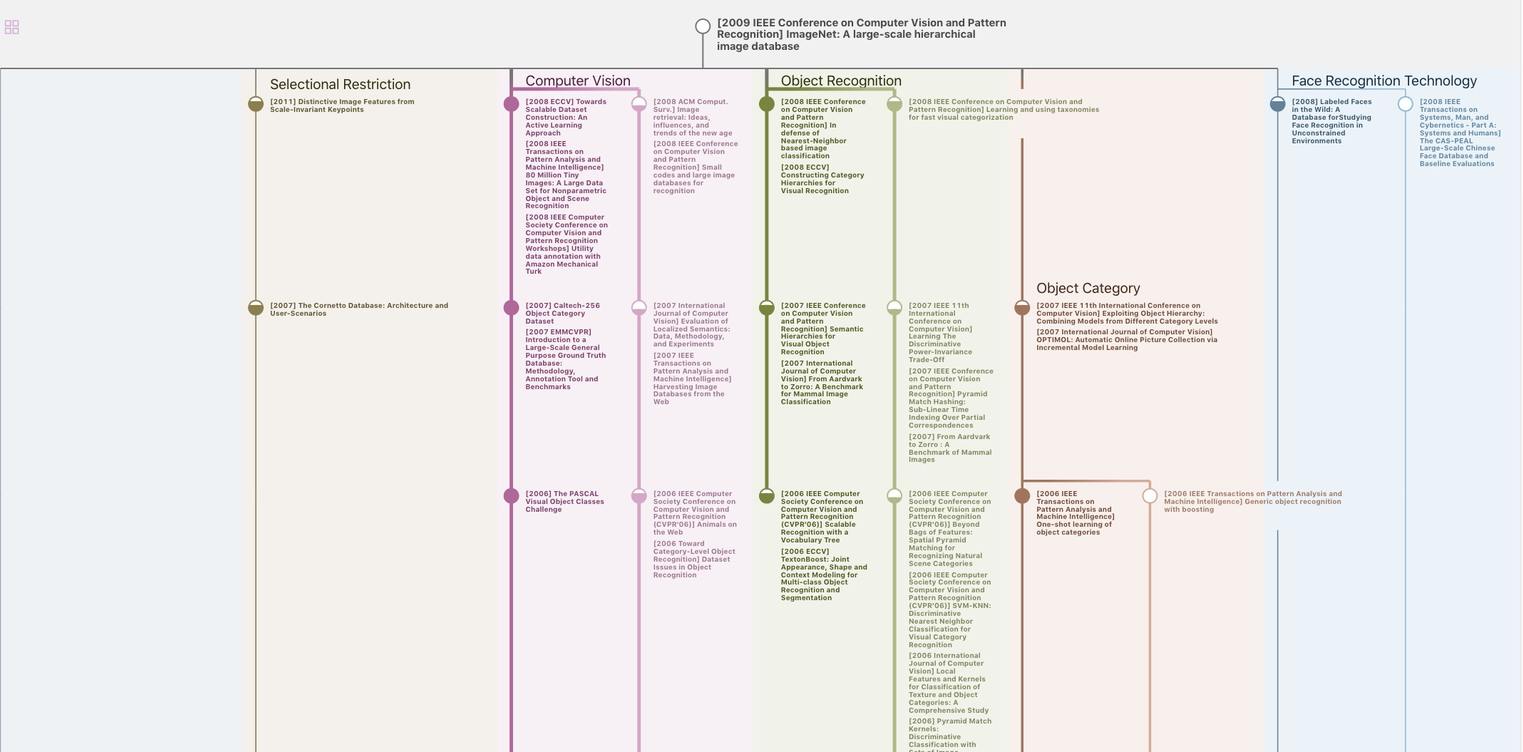
生成溯源树,研究论文发展脉络
Chat Paper
正在生成论文摘要