Automated quality control for mobile data collection
ACM DEV '12: Proceedings of the 2nd ACM Symposium on Computing for Development(2012)
摘要
Systematic interviewer error is a potential issue in any health survey, and it can be especially pernicious in low- and middle-income countries, where survey teams may face problems of limited supervision, chaotic environments, language barriers, and low literacy. Survey teams in such environments could benefit from software that leverages mobile data collection tools to provide solutions for automated data quality control. As a first step in the creation of such software, we investigate and test several algorithms that find anomalous patterns in data. We validate the algorithms using one labeled data set and two unlabeled data sets from two community outreach programs in East Africa. In the labeled set, some of the data is known to be fabricated and some is believed to be relatively accurate. The unlabeled sets are from actual field operations. We demonstrate the feasibility of tools for automated data quality control by showing that the algorithms detect the fake data in the labeled set with a high sensitivity and specificity, and that they detect compelling anomalies in the unlabeled sets.
更多查看译文
关键词
automated data quality control,fake data,actual field operation,survey team,low literacy,automated quality control,east africa,unlabeled data set,mobile data collection tool,health survey,unlabeled set,quality control,data collection
AI 理解论文
溯源树
样例
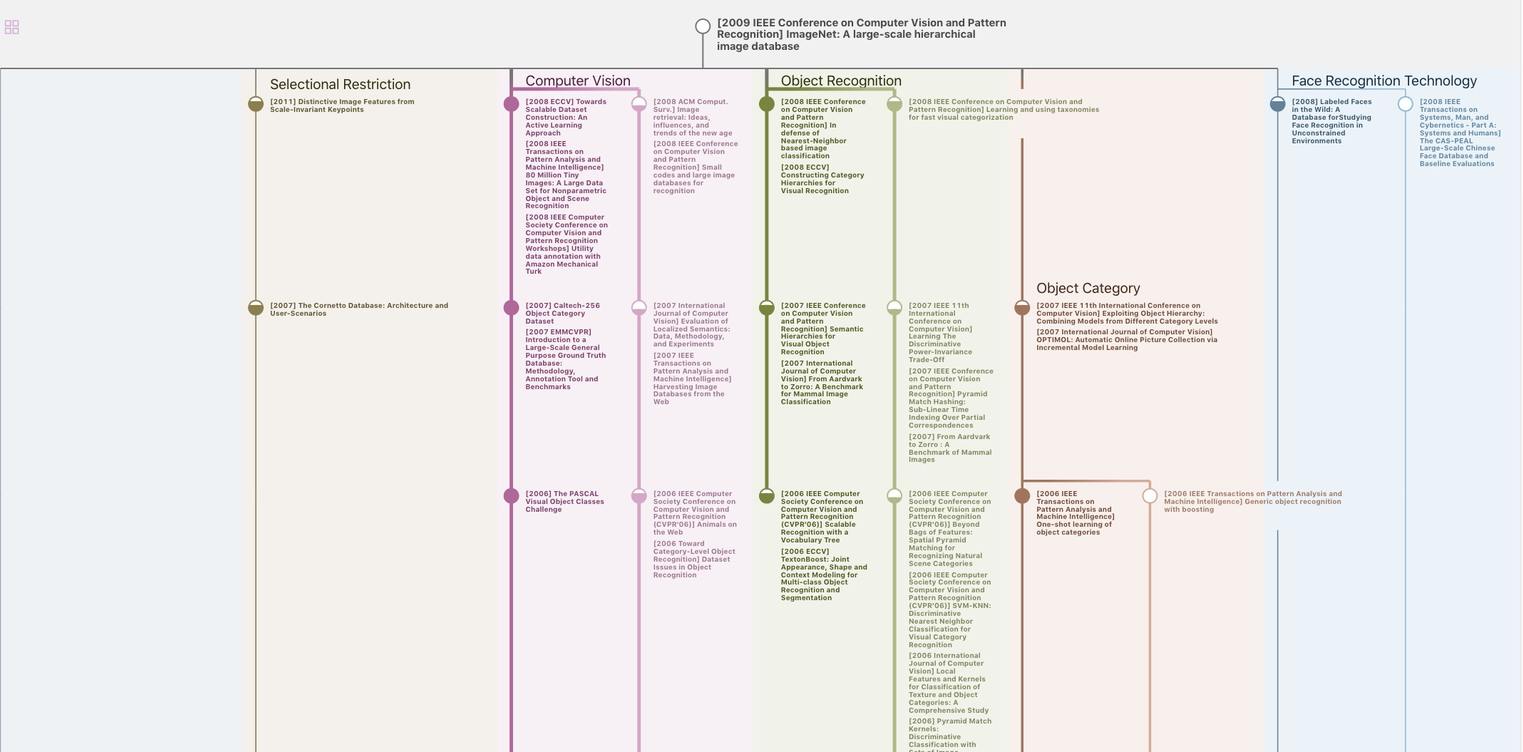
生成溯源树,研究论文发展脉络
Chat Paper
正在生成论文摘要