Leave-One-Out cross-validation based model selection for manifold regularization
ADVANCES IN NEURAL NETWORKS - ISNN 2010, PT 1, PROCEEDINGS(2010)
摘要
Classified labels are expensive by virtue of the utilization of field knowledge while the unlabeled data contains significant information, which can not be explored by supervised learning The Manifold Regularization (MR) based semi-supervised learning (SSL) could explores information from both labeled and unlabeled data Moreover, the model selection of MR seriously affects its predictive performance due to the inherent additional geometry regularizer of SSL In this paper, a leave-one-out cross-validation based PRESS criterion is first presented for model selection of MR to choose appropriate regularization coefficients and kernel parameters The Manifold regularization and model selection algorithm are employed to a real-life benchmark dataset The proposed approach, leveraged by effectively exploiting the embedded intrinsic geometric manifolds, outperforms the original MR and supervised learning approaches.
更多查看译文
关键词
classified label,manifold regularization,model selection algorithm,leave-one-out cross-validation,appropriate regularization coefficient,significant information,original mr,semi-supervised learning,press criterion,unlabeled data,model selection,leave one out cross validation,supervised learning,semi supervised learning
AI 理解论文
溯源树
样例
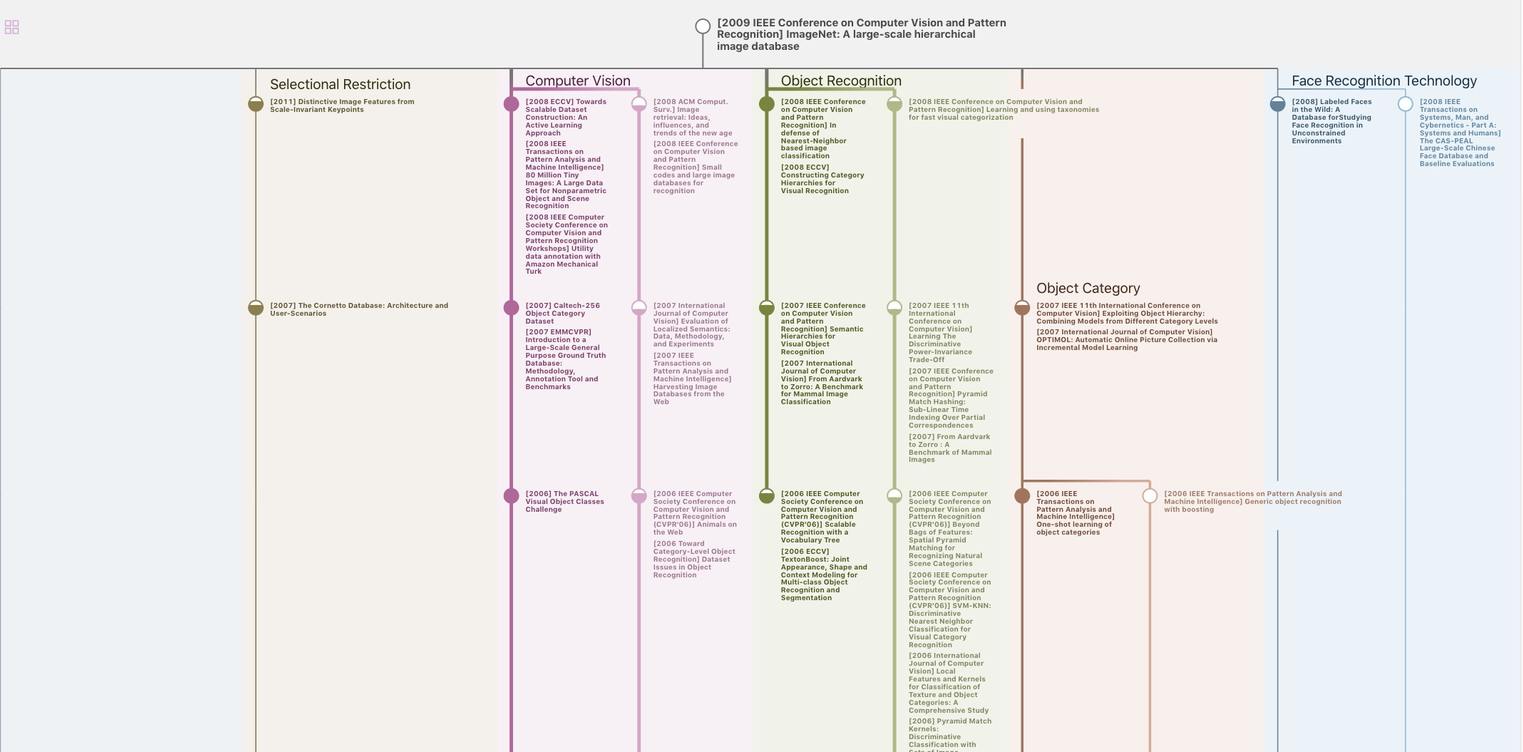
生成溯源树,研究论文发展脉络
Chat Paper
正在生成论文摘要