A supervised fuzzy adaptive resonance theory with distributed weight update
ADVANCES IN NEURAL NETWORKS - ISNN 2010, PT 1, PROCEEDINGS(2010)
摘要
The Fuzzy Adaptive Resonance Theory is an unsupervised clustering algorithm that solves stability plasticity dilemma The existing winner-take-all approach to updating weights in Fuzzy ART has two flaws: (i) it only updates one cluster while an input might belong to more than one cluster and (ii) the winner-take-all approach is costly in training time since it compares one weight to the input at a time We propose an algorithm that compares all weights to the input simultaneously and allows updating multiple matching clusters that pass the vigilance test To mitigate the effects of possibly updating clusters belonging to the wrong class we introduced weight scaling depending on the “closeness” of the weight to the input In addition, we introduced supervision to penalize the weight update for weights that have the wrong class The results show that our algorithm outperformed original Fuzzy ART in both classification accuracy and time consumption.
更多查看译文
关键词
unsupervised clustering algorithm,original fuzzy art,training time,time consumption,weight update,weight scaling,fuzzy adaptive resonance theory,fuzzy art,supervised fuzzy adaptive resonance,existing winner-take-all approach,wrong class,supervised learning,winner take all,adaptive resonance theory,clustering
AI 理解论文
溯源树
样例
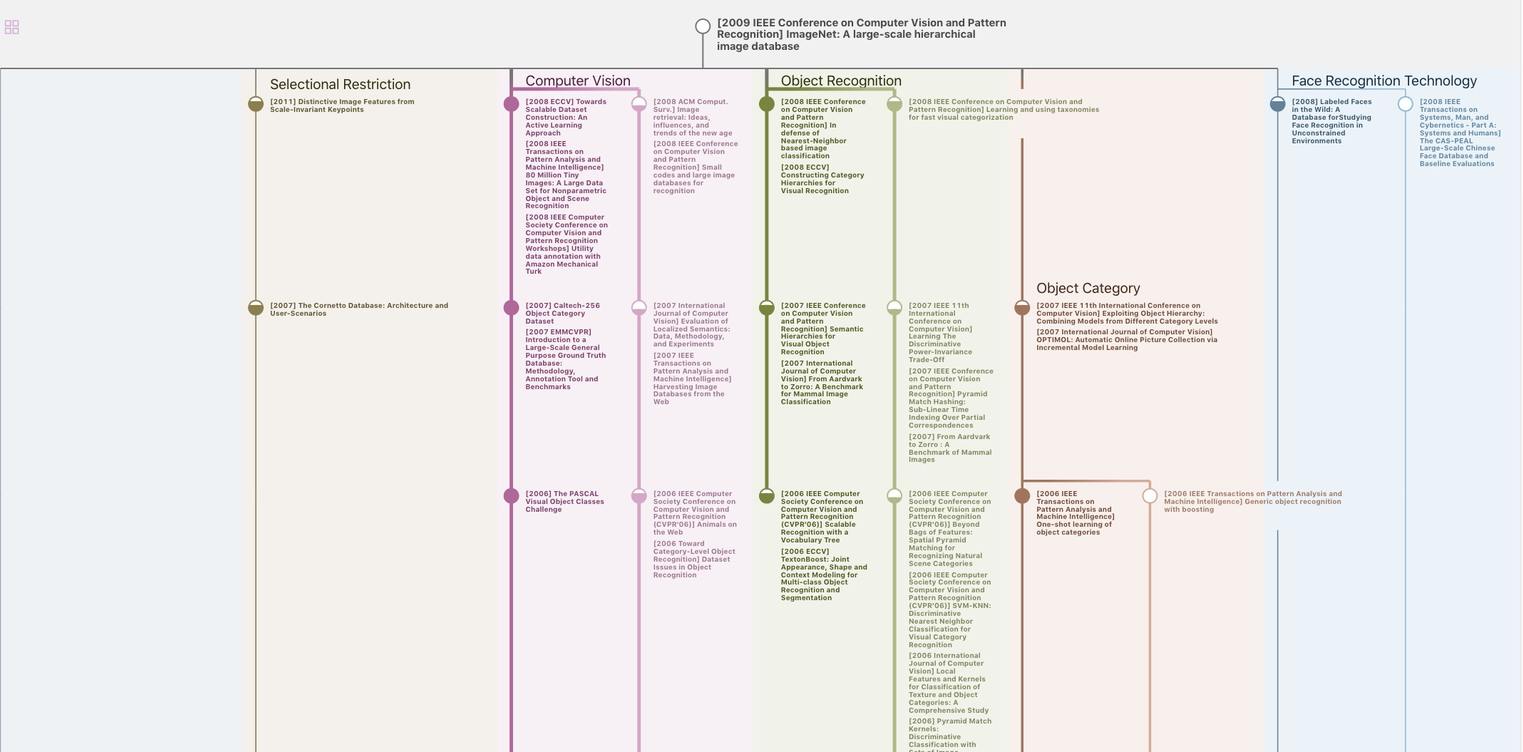
生成溯源树,研究论文发展脉络
Chat Paper
正在生成论文摘要