Identifying the Critical Features That Affect the Job Performance of Survey Interviewers
Technologies and Applications of Artificial Intelligence(2011)
Abstract
In an attempt to build a good predictor of the performance of survey interviewers, we propose a feature selection method that derives the features' strength (i.e., degree of usefulness) from various feature subsets drawn from a pool of all the features. The method also builds a predictor by using support vector regression (SVR) as the learning machine and the selected features as variables. Applying the method to a collection of 278 instances obtained from 67 interviewers par-ticipating in eight survey projects, we identified three critical features, experience and two attributional style variables, out of fifteen features. Compared with results of four existing methods, the proposed predictor produced the smallest predictive error. Furthermore, the three features utilized by our method were also identified as the most important features by the four compared methods.
MoreTranslated text
Key words
fifteen feature,adaptive multiple feature subset (amfes),survey interviewer,good predictor,proposed predictor,surveying,critical feature,feature selection method,learning (artificial intelligence),support vector regression,features identification,fea-ture selection,learning machine,feature subsets,survey project,existing method,job performance,critical features,selected feature,feature selection,survey interviewers,important feature,support vector machines,feature ranking,learning artificial intelligence,prediction error,comparative method
AI Read Science
Must-Reading Tree
Example
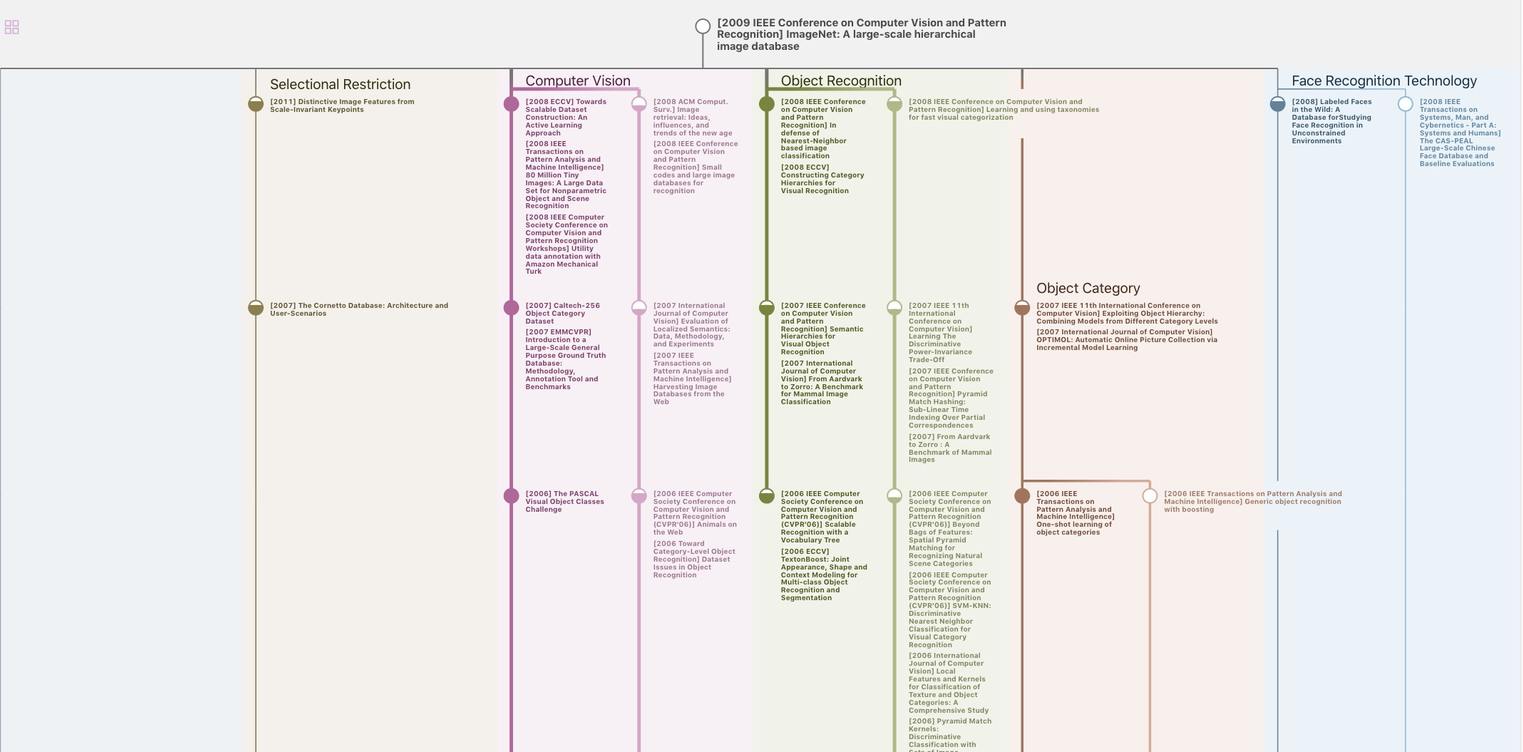
Generate MRT to find the research sequence of this paper
Chat Paper
Summary is being generated by the instructions you defined