Mining Infrequent Causal Associations in Electronic Health Databases
2011 IEEE 11th International Conference on Data Mining Workshops(2011)
Key words
potential causal association,exclusive causal-leverage,causal relationship,infrequent causal relationship,data mining algorithm,innovative data mining framework,potential ADR,real patient data,associated adverse drug reaction,electronic patient datasets,Electronic Health Databases,Mining Infrequent Causal Associations
AI Read Science
Must-Reading Tree
Example
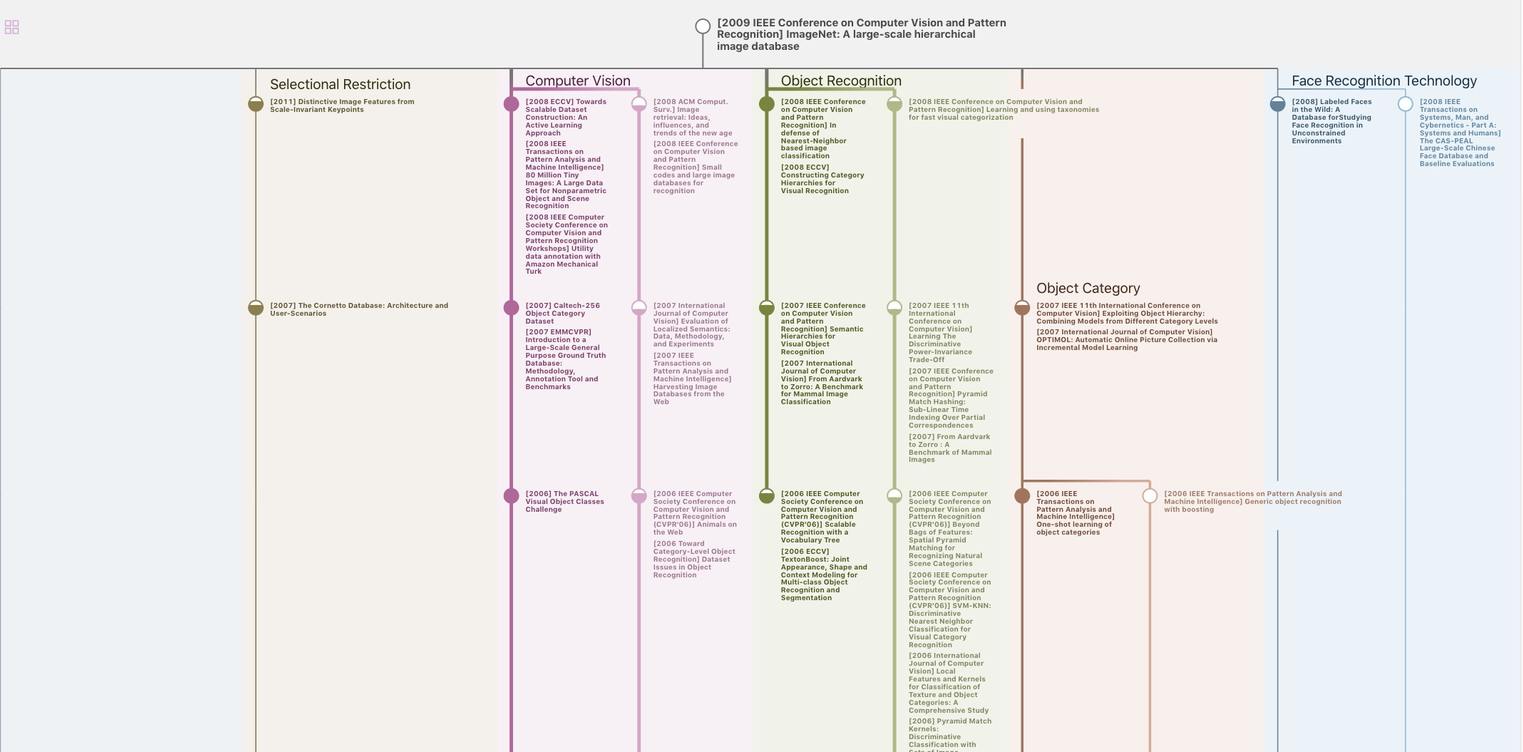
Generate MRT to find the research sequence of this paper
Chat Paper
Summary is being generated by the instructions you defined