Feature Selection for Vibration Sensor Data Transformed by a Streaming Wavelet Packet Decomposition
Tools with Artificial Intelligence(2011)
摘要
Vibration signals play a valuable role in the remote monitoring of high-assurance machinery such as ocean turbines. Because they are waveforms, vibration data must be transformed prior to being incorporated into a machine condition monitoring/prognostic health monitoring (MCM/PHM) solution to detect which frequencies of oscillation are most prevalent. One downside of these transformations, especially the streaming version of the wavelet packet decomposition (denoted SWPD), is that they can produce a large number of features, hindering the model building and evaluation process. In this paper we demonstrate how feature selection techniques may be applied to the output of the SWPD transformation, vastly reducing the total number of features used to build models. The resulting data can be used to build more accurate models for use in MCM/PHM while minimizing computation time.
更多查看译文
关键词
denoted swpd,vibration data,accurate model,total number,swpd transformation,prognostic health monitoring,vibration signal,feature selection,vibration sensor data,machine condition monitoring,remote monitoring,large number,streaming wavelet packet decomposition,vectors,vibrations,oscillations,data mining,data transformation,wavelet transforms,discrete wavelet transform,wavelet packets,wavelet packet decomposition,model building,classification,time series,time series analysis
AI 理解论文
溯源树
样例
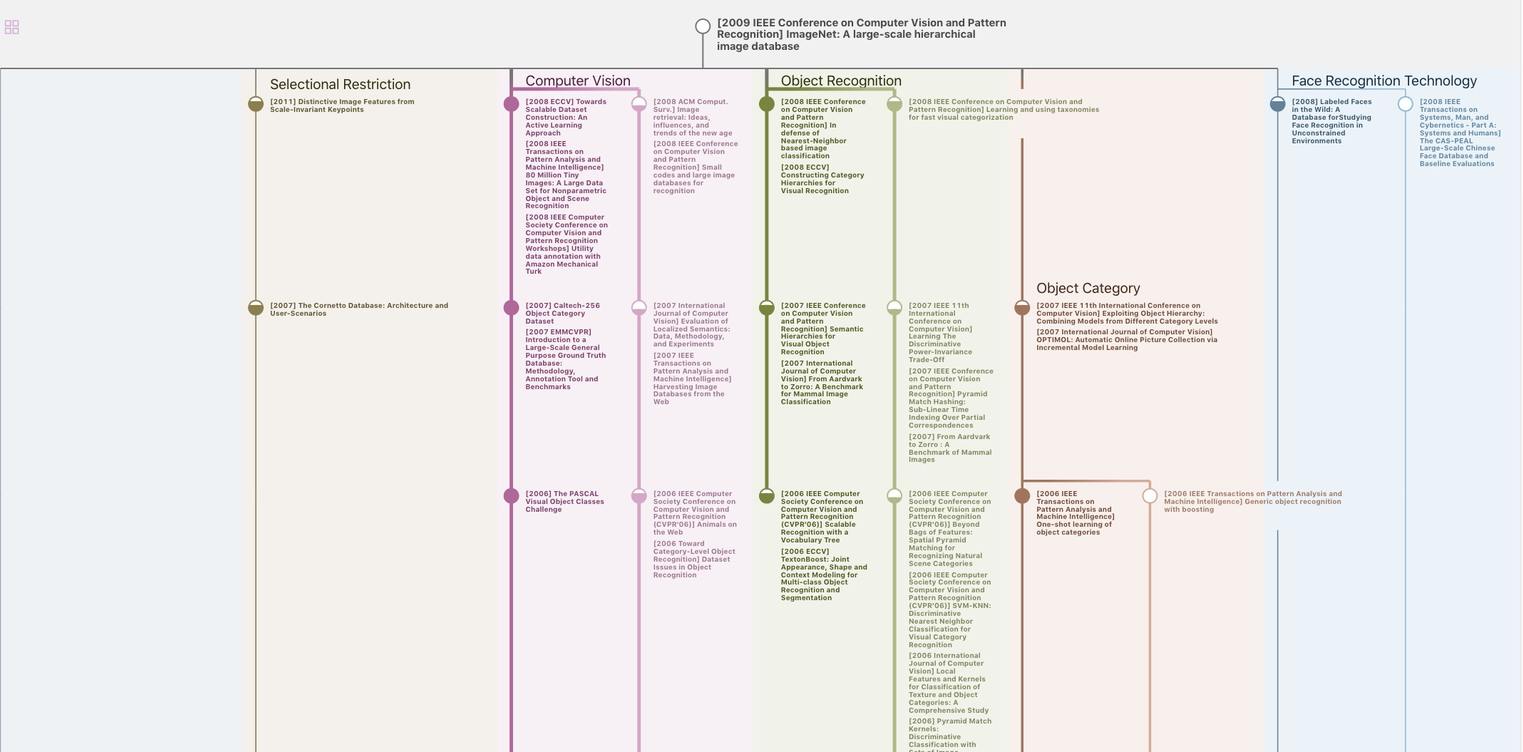
生成溯源树,研究论文发展脉络
Chat Paper
正在生成论文摘要