Applications of gibbs measure theory to loopy belief propagation algorithm
MICAI 2006: ADVANCES IN ARTIFICIAL INTELLIGENCE, PROCEEDINGS(2006)
摘要
In this paper, we pursue application of Gibbs measure theory to LBP in two ways. First, we show this theory can be applied directly to LBP for factor graphs, where one can use higher-order potentials. Consequently, we show beliefs are just marginal probabilities for a certain Gibbs measure on a computation tree. We also give a convergence criterion using this tree. Second, to see the usefulness of this approach, we apply a well-known general condition and a special one, which are developed in Gibbs measure theory, to LBP. We compare these two criteria and another criterion derived by the best present result. Consequently, we show that the special condition is better than the others and also show the general condition is better than the best present result when the influence of one-body potentials is sufficiently large. These results surely encourage the use of Gibbs measure theory in this area.
更多查看译文
关键词
general condition,higher-order potential,gibbs measure theory,belief propagation algorithm,convergence criterion,present result,factor graph,computation tree,certain gibbs measure,well-known general condition,special condition,higher order,loopy belief propagation,gibbs measure
AI 理解论文
溯源树
样例
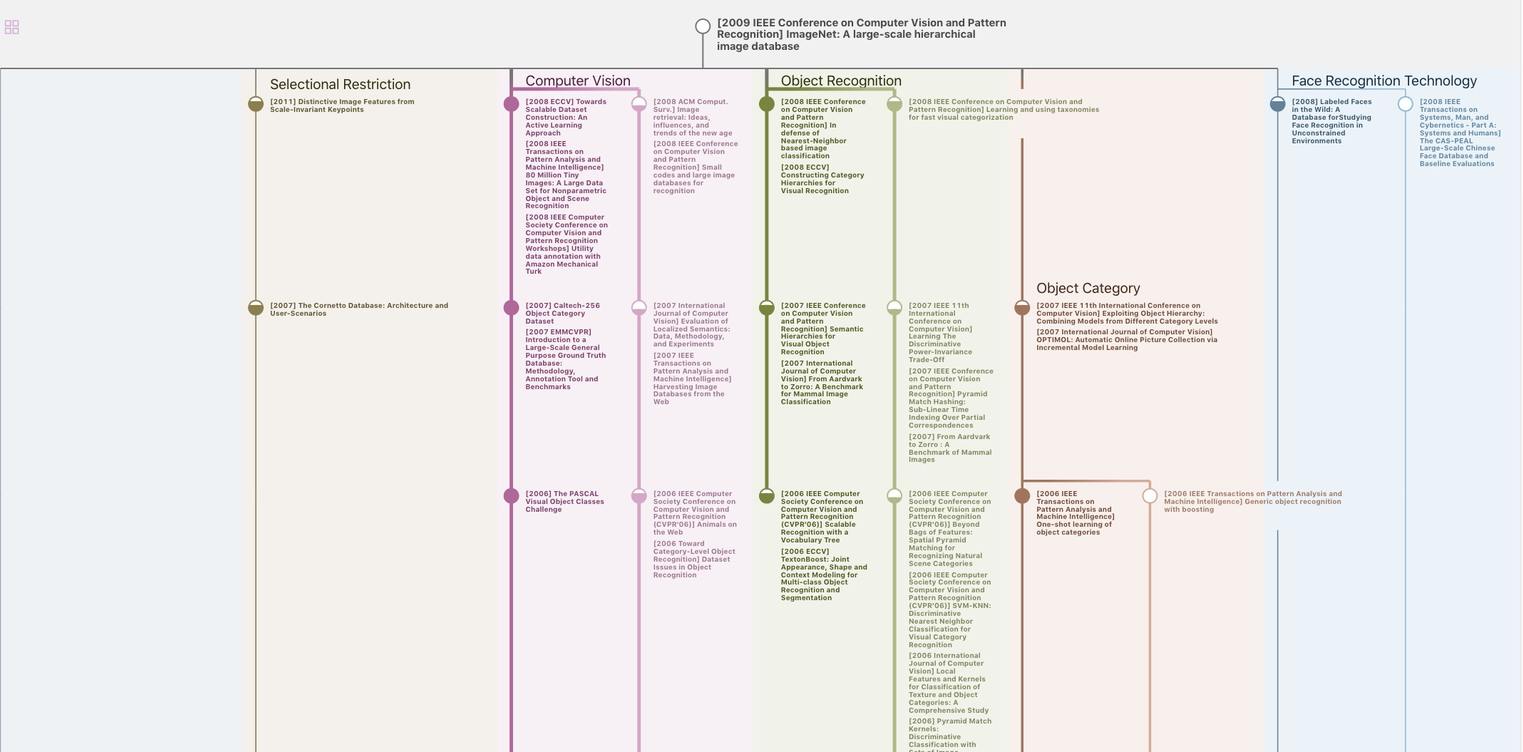
生成溯源树,研究论文发展脉络
Chat Paper
正在生成论文摘要