Cross-domain activity recognition via transfer learning
Pervasive and Mobile Computing(2011)
摘要
In activity recognition, one major challenge is how to reduce the labeling effort one needs to make when recognizing a new set of activities. In this paper, we analyze the possibility of transferring knowledge from the available labeled data on a set of existing activities in one domain to help recognize the activities in another different but related domain. We found that such a knowledge transfer process is possible, provided that the recognized activities from the two domains are related in some way. We develop a bridge between the activities in two domains by learning a similarity function via Web search, under the condition that the sensor readings are from the same feature space. Based on the learned similarity measure, our algorithm interprets the data from the source domain as ''pseudo training data'' in the target domain with different confidence levels, which are in turn fed into supervised learning algorithms for training the classifier. We show that after using this transfer learning approach, the performance of activity recognition in the new domain is increased several fold as compared to when no knowledge transfer is done. Our algorithm is evaluated on several real-world datasets to demonstrate its effectiveness. In the experiments, our algorithm could achieve a 60% accuracy most of the time with no or very few training data in the target domain, which easily outperforms the supervised learning methods.
更多查看译文
关键词
new domain,related domain,activity recognition,supervised learning algorithm,pseudo training data,target domain,training data,transfer learning,knowledge transfer,supervised learning method,source domain,cross-domain activity recognition,web mining,supervised learning,confidence level,feature space
AI 理解论文
溯源树
样例
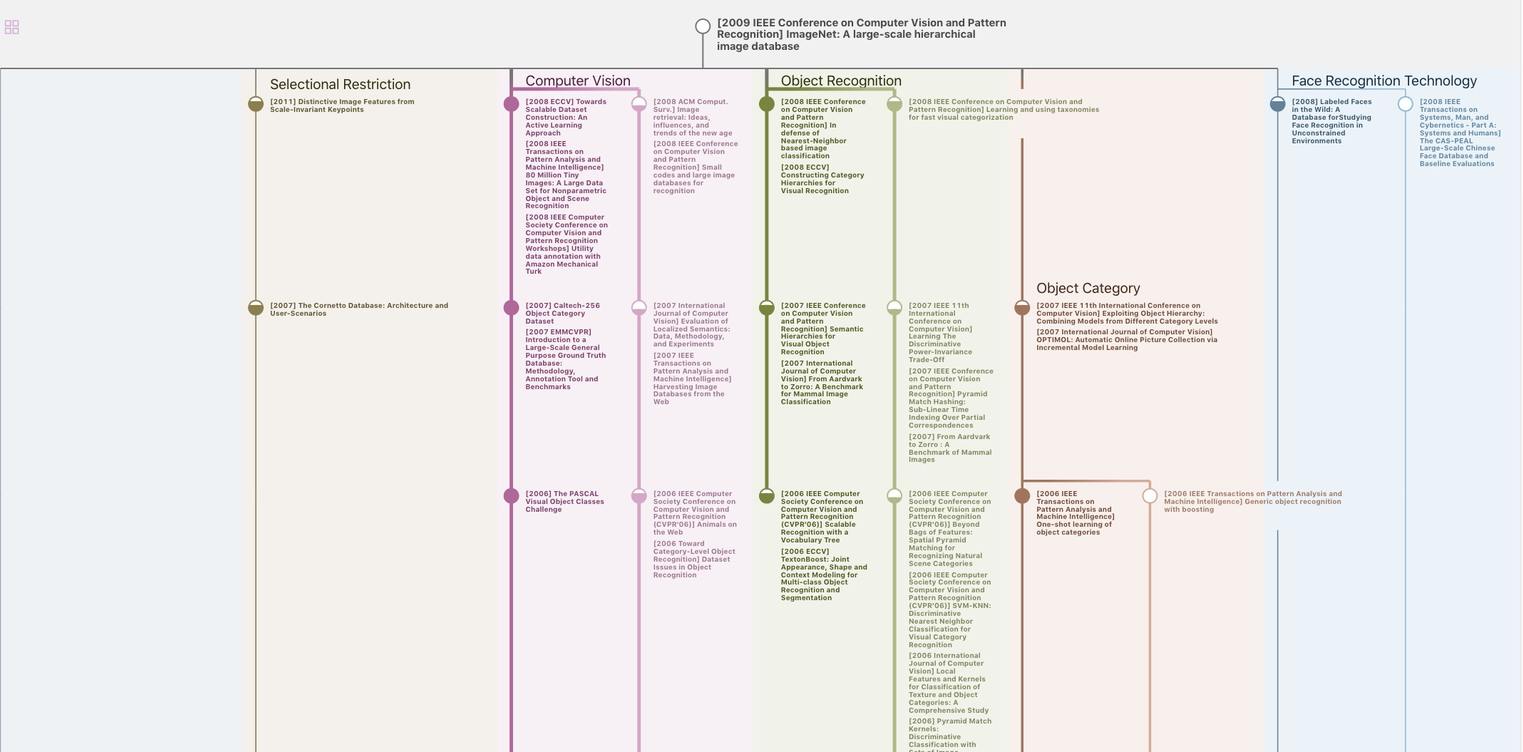
生成溯源树,研究论文发展脉络
Chat Paper
正在生成论文摘要