Edge Weight Regularization over Multiple Graphs for Similarity Learning
Data Mining(2010)
摘要
The growth of the web has directly influenced the increase in the availability of relational data. One of the key problems in mining such data is computing the similarity between objects with heterogeneous feature types. For example, publications have many heterogeneous features like text, citations, authorship information, venue information, etc. In most approaches, similarity is estimated using each feature type in isolation and then combined in a linear fashion. However, this approach does not take advantage of the dependencies between the different feature spaces. In this paper, we propose a novel approach to combine the different sources of similarity using a regularization framework over edges in multiple graphs. We show that the objective function induced by the framework is convex. We also propose an efficient algorithm using coordinate descent [1] to solve the optimization problem. We extrinsically evaluate the performance of the proposed unified similarity measure on two different tasks, clustering and classification. The proposed similarity measure outperforms three baselines and a state-of-the-art classification algorithm on a variety of standard, large data sets.
更多查看译文
关键词
convex programming,data mining,graph theory,pattern classification,pattern clustering,convex function,data classification,data clustering,data mining,edge weight regularization,multiple graph,optimization,regularization framework,relational data,similarity learning,Classification,Clustering,Heterogeneous Features,Machine Learning,Similarity Learning
AI 理解论文
溯源树
样例
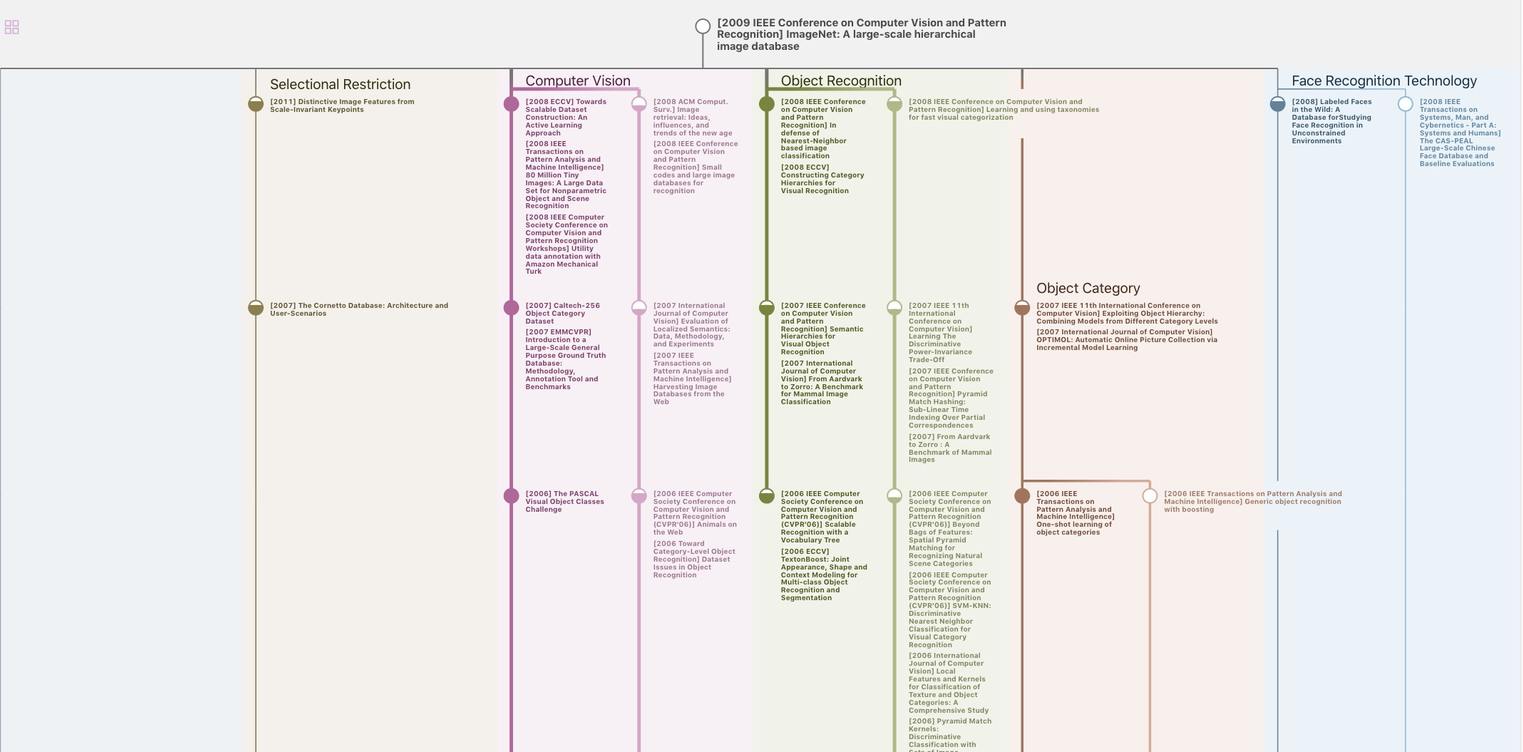
生成溯源树,研究论文发展脉络
Chat Paper
正在生成论文摘要