Selecting Features Using the SFS in Conjunction with Vector Quantization
Frontiers in Handwriting Recognition(2010)
摘要
When discrete Hidden-Markov-Models (HMMs)-based recognition is performed, vector quantization (VQ) is used to transform continuous observations to sequences of discrete symbols. After VQ, the quantization error is not spread equally among the features. This impairs the feature significance, which is important when features are selected, e. g. by applying the Sequential Forward Selection (SFS). In this paper, we introduce a novel vector quantization (VQ) scheme for distributing the quantization error equally among the quantized dimensions of a feature vector. Afterwards, the proposed VQ scheme is used to apply the SFS on the features in on-line handwritten whiteboard note recognition based on discrete HMMs. In an experimental section, we show that the novel VQ scheme derives feature sets of almost half the size of the feature sets gained when standard VQ is used for quantization, while the performance stays the same.
更多查看译文
关键词
error analysis,handwriting recognition,hidden Markov models,vector quantisation,SFS,discrete hidden Markov model,discrete symbol,feature selection,online handwritten whiteboard note recognition,quantization error,sequential forward selection,vector quantization
AI 理解论文
溯源树
样例
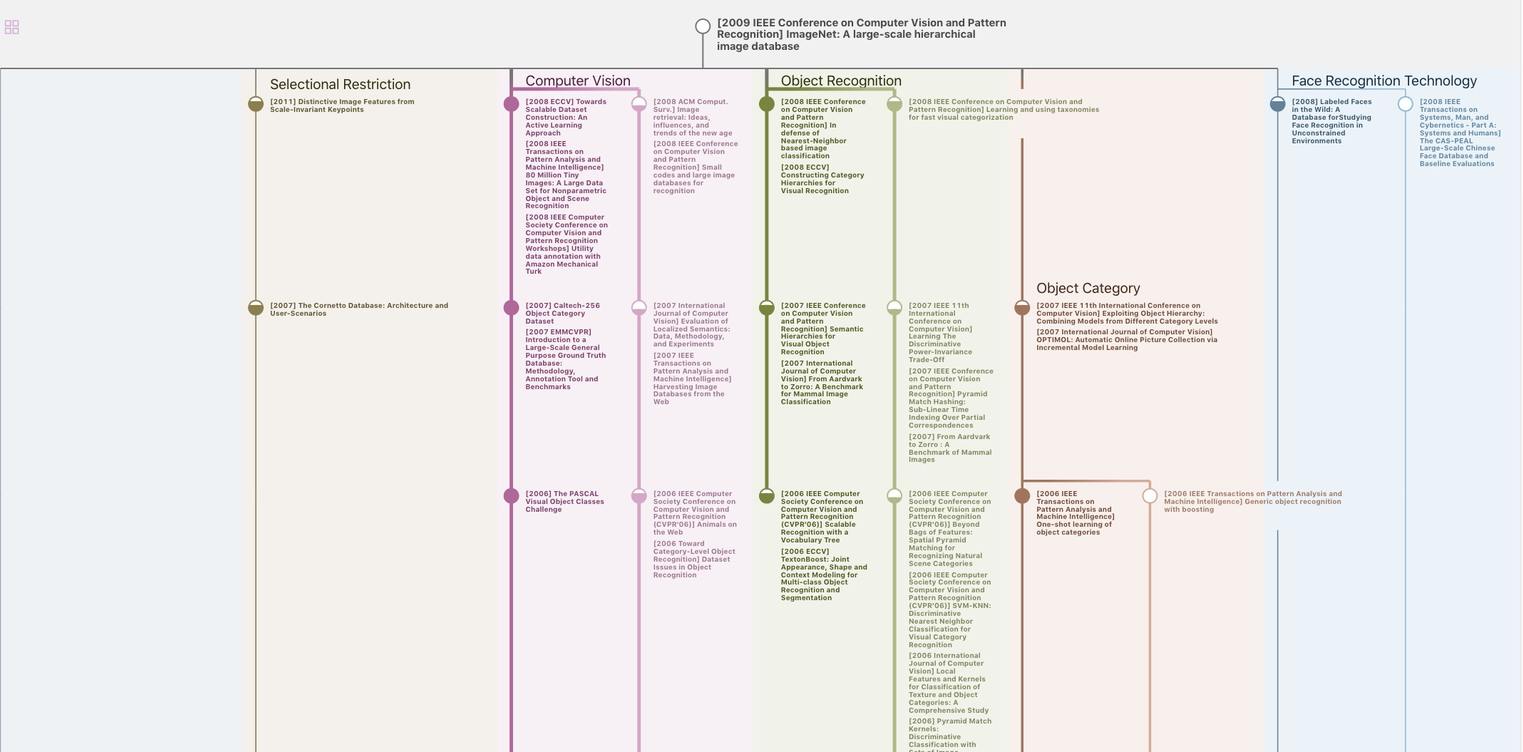
生成溯源树,研究论文发展脉络
Chat Paper
正在生成论文摘要