Time-Series Classification Based on Individualised Error Prediction
Computational Science and Engineering(2010)
摘要
Time-series classification is an active research topic in machine learning, as it finds applications in numerous domains. The k-NN classifier, based on the discrete time warping (DTW) distance, had been shown to be competitive to many state-of-the art time-series classification methods. Nevertheless, due to the complexity of time-series data sets, our investigation demonstrates that a single, global choice for k (= 1) can become sub optimal, because each individual region of a data set may require a different k value. In this paper, we proposed a novel individualized error prediction (IEP) mechanism that considers a range of k-NN classifiers (for different k values) and uses secondary regression models that predict the error of each such classifier. This permits to perform k-NN time-series classification in a more fine grained fashion that adapts to the varying characteristics among different regions by avoiding the restriction of a single value of k. Our experimental evaluation, using a large collection of real time-series data, indicates that the proposed method is more robust and compares favorably against two examined baselines by resulting in significant reduction in the classification error.
更多查看译文
关键词
real time-series data,k-nn classifier,different region,k-nn time-series classification,classification error,state-of-the art time-series classification,time-series classification,time-series data set,different k value,individualised error prediction,time series data,set theory,predictive models,time series,accuracy,regression analysis,regression model,learning artificial intelligence,discrete time,data set,data mining,data models,training data,machine learning,classification,time series analysis
AI 理解论文
溯源树
样例
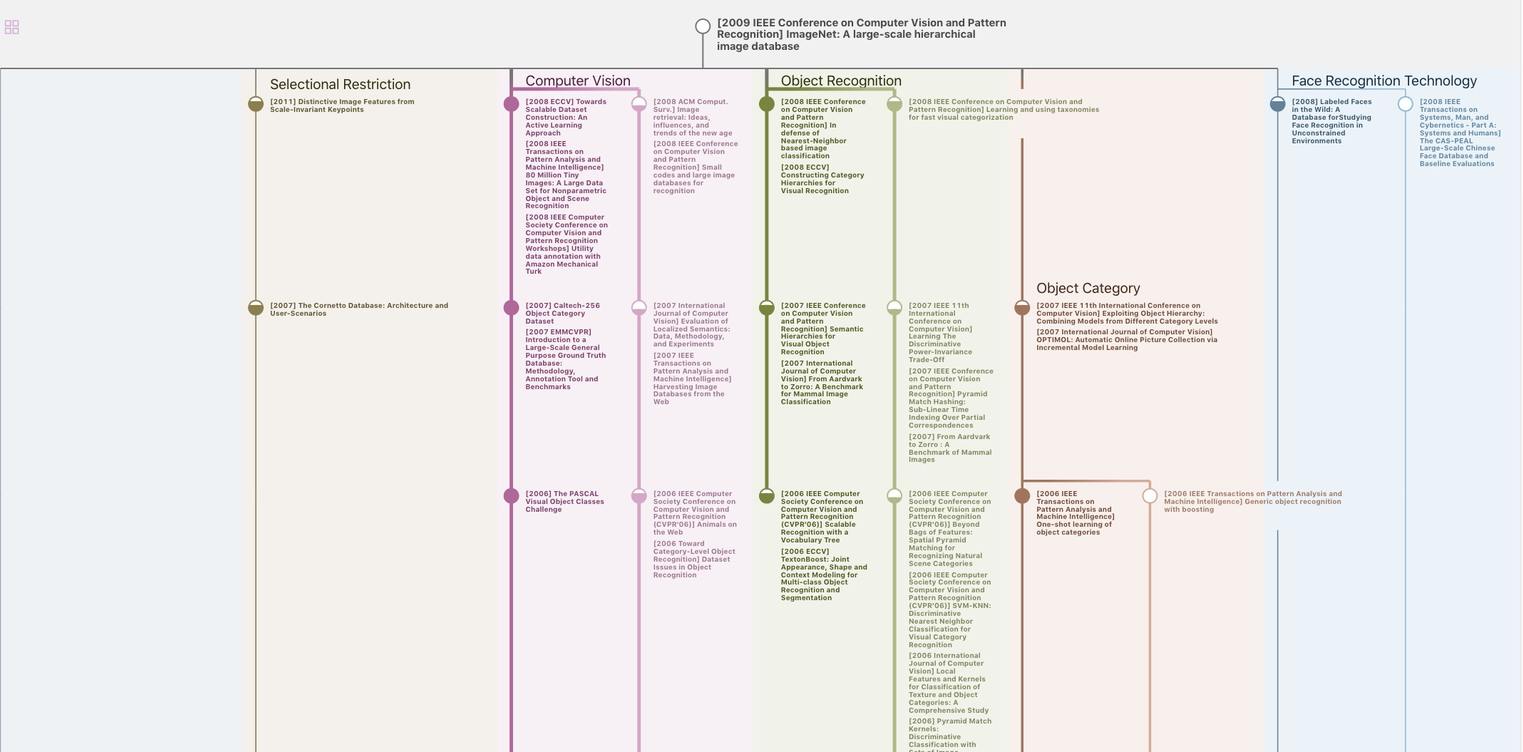
生成溯源树,研究论文发展脉络
Chat Paper
正在生成论文摘要