A Re-evaluation of Pedestrian Detection on Riemannian Manifolds
Pattern Recognition(2010)
摘要
Boosting covariance data on Riemannian manifolds has proven to be a convenient strategy in a pedestrian detection context. In this paper we show that the detection performances of the state-of-the-art approach of Tuzel et al. [7] can be greatly improved, from both a computational and a qualitative point of view, by considering practical and theoretical issues, and allowing also the estimation of occlusions in a fine way. The resulting detection system reaches the best performance on the INRIA dataset, setting novel state-of-the art results.
更多查看译文
关键词
riemannian manifold,inria dataset,novel state-of-the art result,pedestrian detection,detection system,riemannian manifolds,convenient strategy,qualitative point,covariance data,best performance,pedestrian detection context,detection performance,boosting,polynomials,manifolds,estimation theory,symmetric matrices
AI 理解论文
溯源树
样例
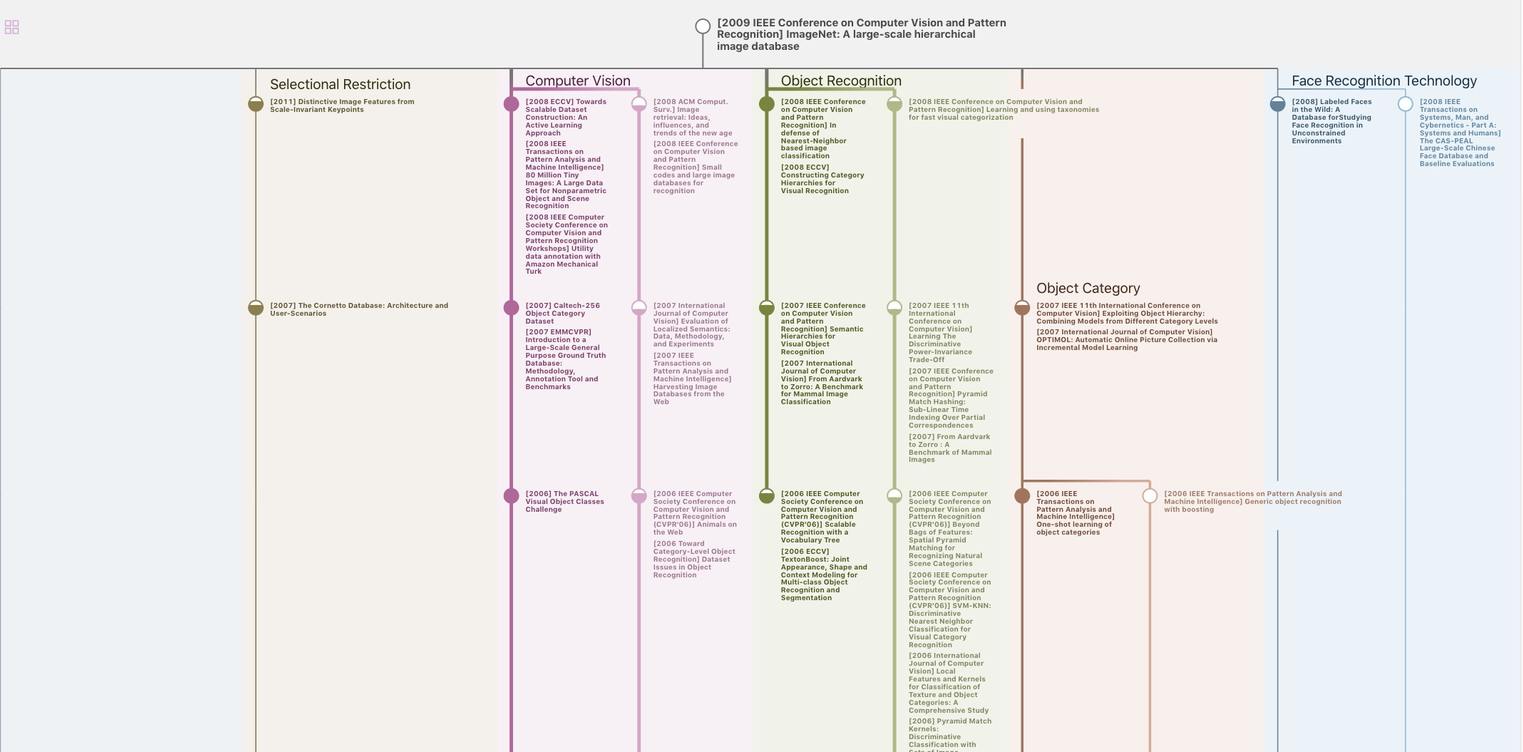
生成溯源树,研究论文发展脉络
Chat Paper
正在生成论文摘要