Text clustering using frequent itemsets
Knowledge-Based Systems(2010)
Abstract
Frequent itemset originates from association rule mining. Recently, it has been applied in text mining such as document categorization, clustering, etc. In this paper, we conduct a study on text clustering using frequent itemsets. The main contribution of this paper is three manifolds. First, we present a review on existing methods of document clustering using frequent patterns. Second, a new method called Maximum Capturing is proposed for document clustering. Maximum Capturing includes two procedures: constructing document clusters and assigning cluster topics. We develop three versions of Maximum Capturing based on three similarity measures. We propose a normalization process based on frequency sensitive competitive learning for Maximum Capturing to merge cluster candidates into predefined number of clusters. Third, experiments are carried out to evaluate the proposed method in comparison with CFWS, CMS, FTC and FIHC methods. Experiment results show that in clustering, Maximum Capturing has better performances than other methods mentioned above. Particularly, Maximum Capturing with representation using individual words and similarity measure using asymmetrical binary similarity achieves the best performance. Moreover, topics produced by Maximum Capturing distinguished clusters from each other and can be used as labels of document clusters.
MoreTranslated text
Key words
document clustering,asymmetrical binary similarity,similarity measure,document categorization,competitive learning,frequent itemset,frequent itemsets,document cluster,fihc method,frequent pattern,maximum capturing,text clustering,association rule mining,text mining
AI Read Science
Must-Reading Tree
Example
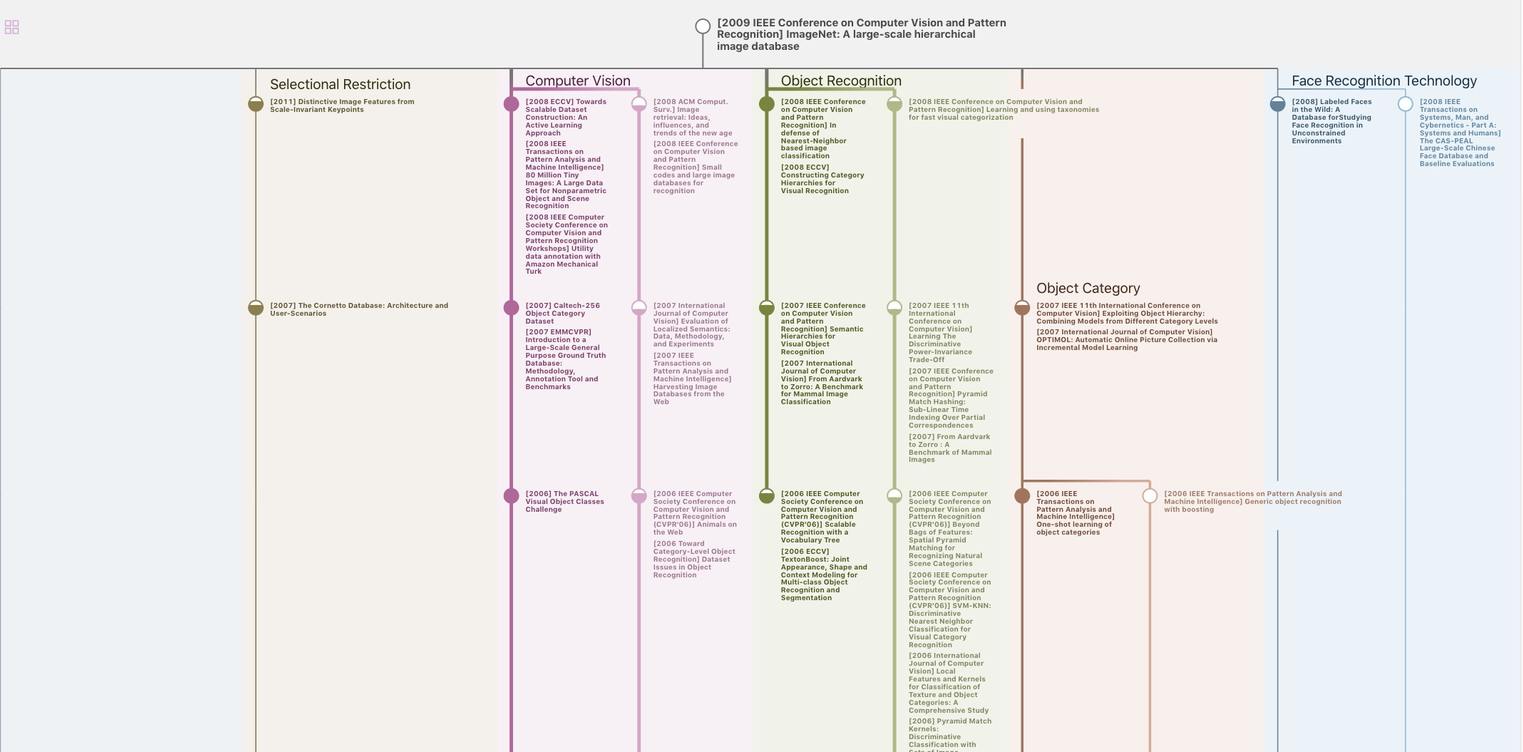
Generate MRT to find the research sequence of this paper
Chat Paper
Summary is being generated by the instructions you defined