Bridging Domains Using World Wide Knowledge for Transfer Learning
IEEE Transactions on Knowledge and Data Engineering(2010)
摘要
A major problem of classification learning is the lack of ground-truth labeled data. It is usually expensive to label new data instances for training a model. To solve this problem, domain adaptation in transfer learning has been proposed to classify target domain data by using some other source domain data, even when the data may have different distributions. However, domain adaptation may not work well when the differences between the source and target domains are large. In this paper, we design a novel transfer learning approach, called BIG (Bridging Information Gap), to effectively extract useful knowledge in a worldwide knowledge base, which is then used to link the source and target domains for improving the classification performance. BIG works when the source and target domains share the same feature space but different underlying data distributions. Using the auxiliary source data, we can extract a “bridge” that allows cross-domain text classification problems to be solved using standard semisupervised learning algorithms. A major contribution of our work is that with BIG, a large amount of worldwide knowledge can be easily adapted and used for learning in the target domain. We conduct experiments on several real-world cross-domain text classification tasks and demonstrate that our proposed approach can outperform several existing domain adaptation approaches significantly.
更多查看译文
关键词
data mining,learning (artificial intelligence),text analysis,bridging information gap,classification learning,cross-domain text classification problems,data distributions,data mining,ground-truth labeled data,semisupervised learning algorithms,transfer learning,world wide knowledge,Data mining,Wikipedia.,cross-domain,text classification,transfer learning
AI 理解论文
溯源树
样例
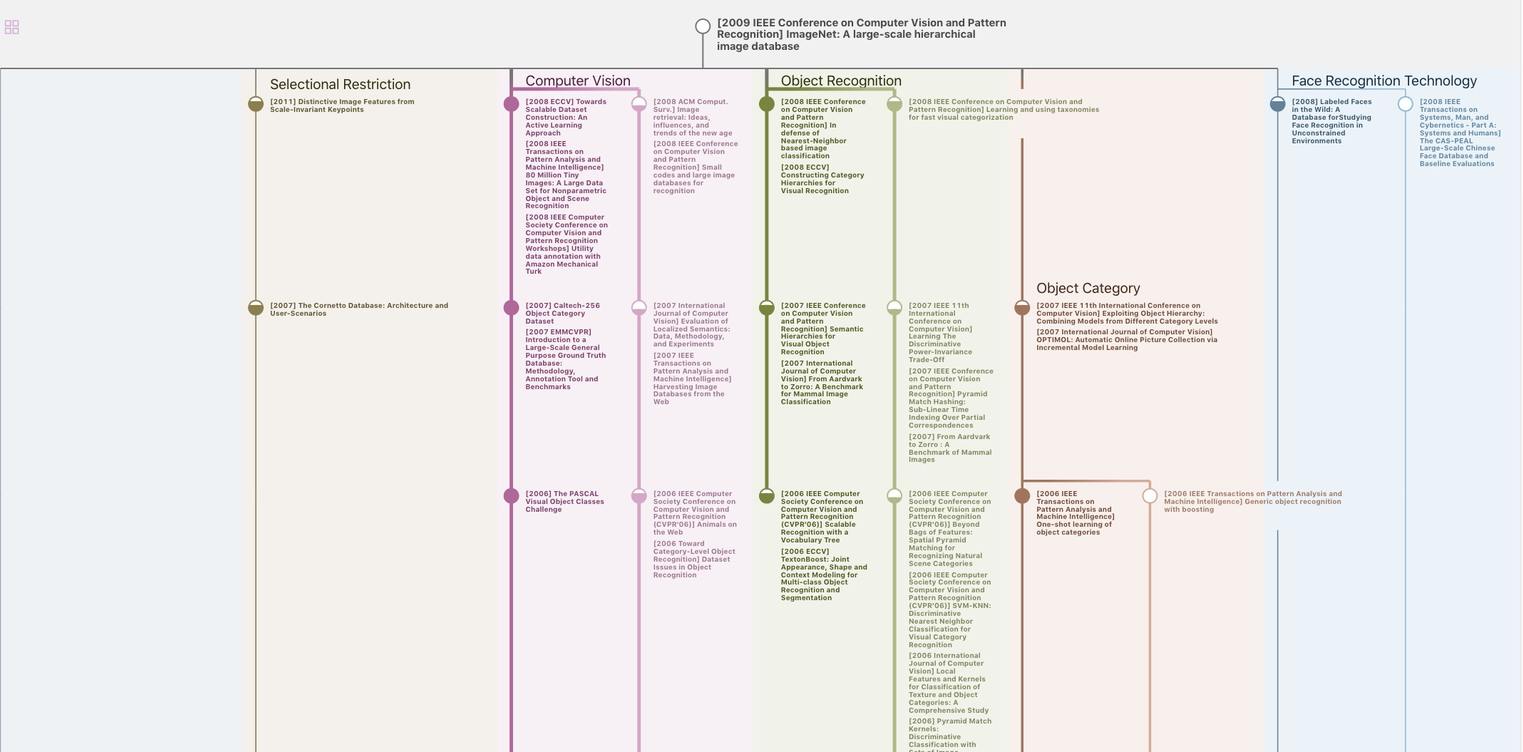
生成溯源树,研究论文发展脉络
Chat Paper
正在生成论文摘要