Semisupervised Hyperspectral Image Classification with SVM and PSO
ICMTMA), 2010 International Conference(2010)
摘要
This paper proposes a novel semi supervised approach to classify hyperspectral image. This method can overcome the limited training samples problem. It combines support vector machine (SVM) and particle swarm optimization(PSO). The new approach exploits the wealth of unlabeled samples for improving the classification accuracy. The method can inflate the original training samples by estimating the labels of the unlabeled samples. The label estimation process is performed by the designed PSO. The effectiveness of the proposed system is carried on a real hyperspectral data set. The experimental results indicate that the classification performance generated by the proposed algorithm is generally competitive.
更多查看译文
关键词
hyperspectral data set,novel semi supervised approach,learning (artificial intelligence),pso,particle swarm optimisation,semisupervised hyperspectral image classification,original training sample,svm,limited training samples problem,image classification,support vector machine,proposed algorithm,proposed system,data inflation,classification accuracy,semisupervised,classification performance,new approach,hyperspectral image,support vector machines,unlabeled sample,particle swarm optimization,remote sensing,learning artificial intelligence,hyperspectral imaging,automation,accuracy,kernel,hyperspectral sensors,mechatronics
AI 理解论文
溯源树
样例
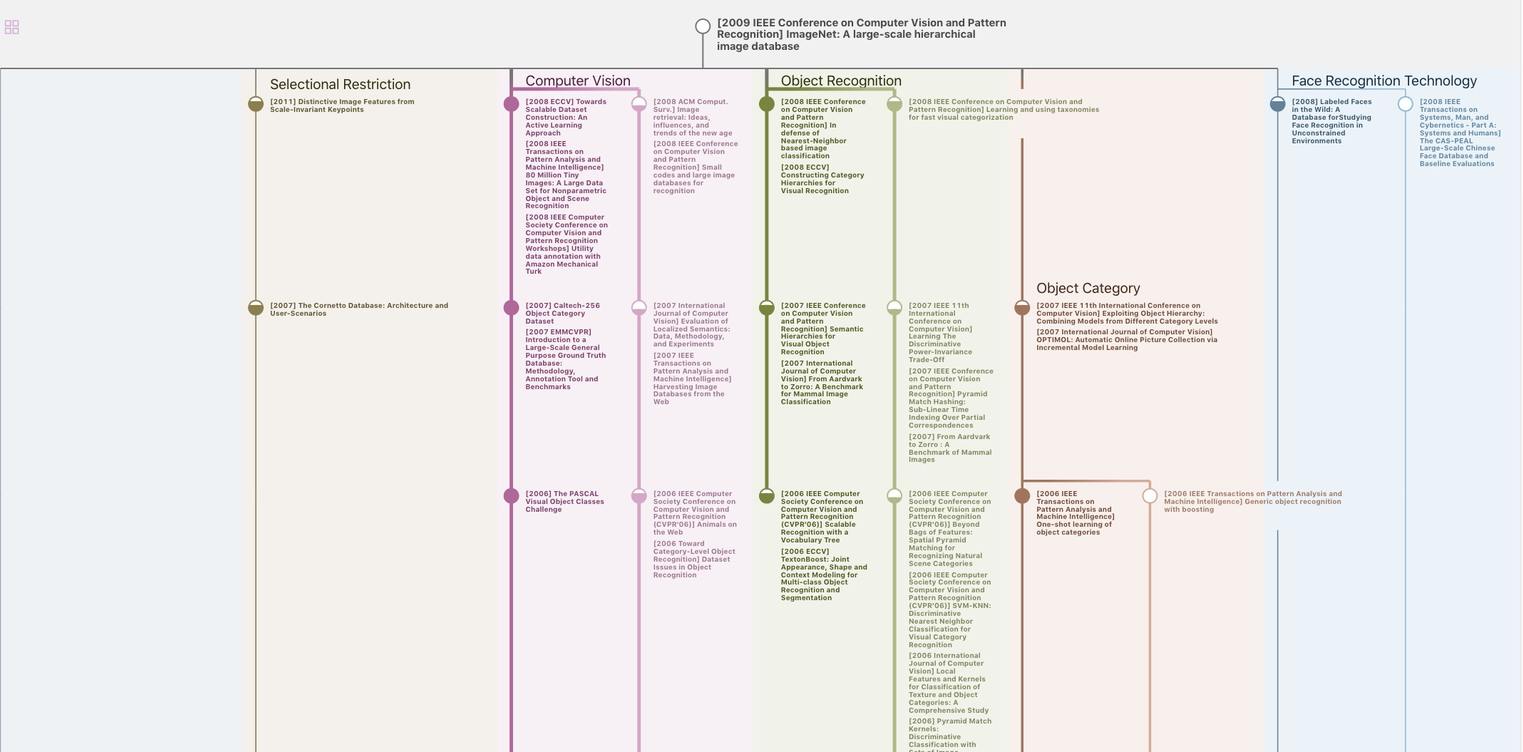
生成溯源树,研究论文发展脉络
Chat Paper
正在生成论文摘要