Association Rule Mining in Multiple, Multidimensional Time Series Medical Data
Journal of healthcare informatics research(2017)
摘要
Time series pattern mining (TSPM) finds correlations or dependencies in same series or in multiple time series. When numerous instances of multiple time series data are associated with different quantitative attributes, they form a multiple multidimensional framework. In this paper, we consider real-life time series data of muscular activities of human participants obtained from multiple electromyogram (EMG) sensors and discover patterns in these EMG time series data. Each EMG time series data is associated with quantitative attributes such as energy of the signal and onset time, which are required to be mined along with EMG time series patterns. We propose a two-stage approach for this purpose: in the first stage, our emphasis is on discovering frequent patterns in multiple time series by doing sequential mining across time slices. And in the next stage, we focus on the quantitative attributes of only those time series that are present in the patterns discovered in the first stage. Our evaluation with large sets of time series data from multiple EMG sensors demonstrate that our two-stage approach speeds up the process of finding association rules in such multidimensional environment as compared to other methods and scales up linearly in terms of number of time series involved. Our approach is generic in finding association rules in other medical sensor databases containing multiple time series associated with quantitative attributes, which can be used in extending research areas like rehabilitation programs or designing better prosthetic devices.
更多查看译文
关键词
Association rules,Multi-attribute,Pattern mining,Clustering,Electromyograms
AI 理解论文
溯源树
样例
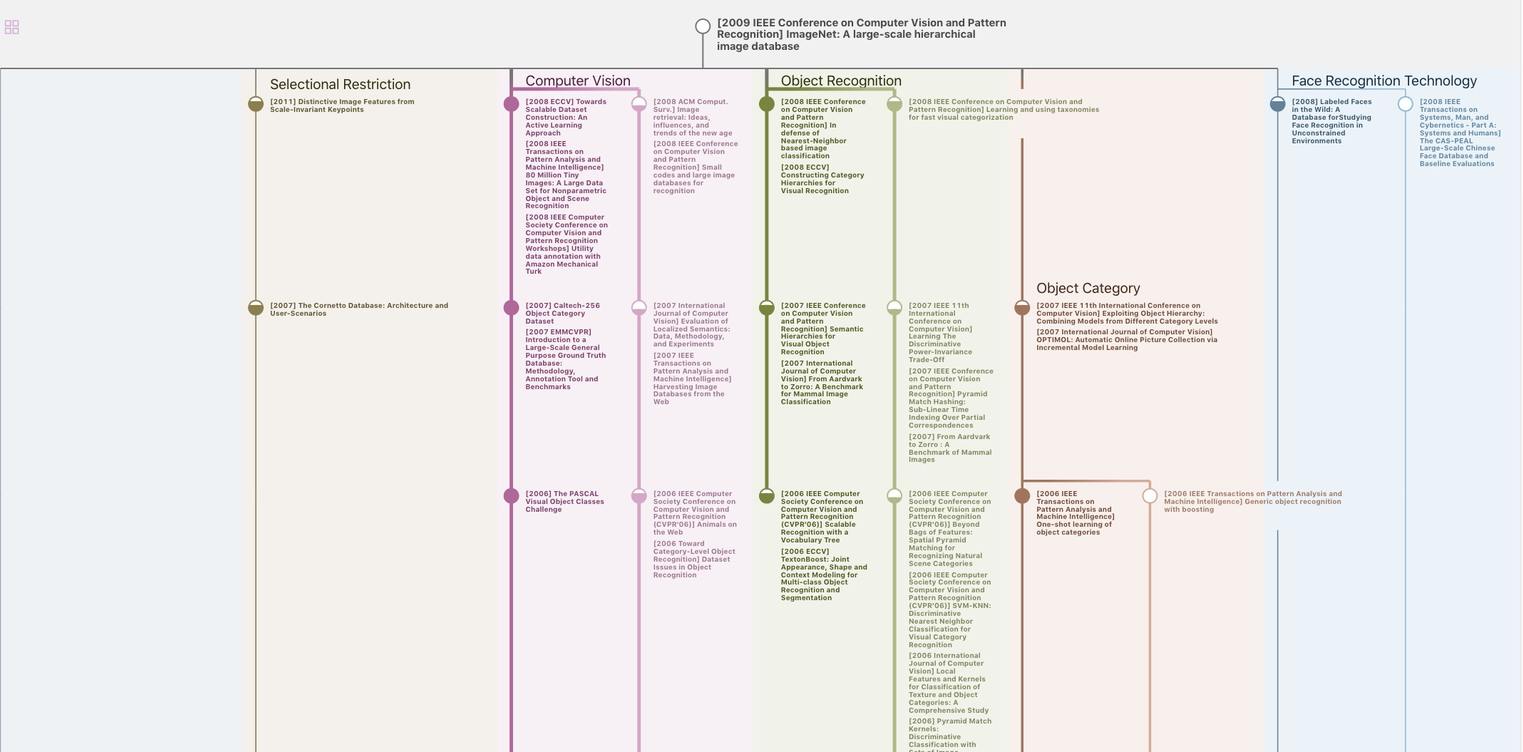
生成溯源树,研究论文发展脉络
Chat Paper
正在生成论文摘要