Exploring an Improved Decision Tree Based Weights.
ICNC (1)(2009)
摘要
Although Decision Tree learning has achieved great success in building classifier, most existing methods don't pay attention to unequal weights between different instances from training and testing data sets. However, many real world data sets are imbalanced in nature. In this paper, we introduce a new improved decision tree based weights, which considers imbalanced weights between different instances, to address the class imbalanced problems. The proposed decision tree algorithm is simple and more effective in implementation than previous decision trees. Also, the new proposed algorithm will be compared with C4.5 (a novel decision tree algorithm) experimentally and the experiment results testify that our proposed algorithm outperforms C4.5 significantly, in terms of the improvement of the classification accuracy in UCI data sets.
更多查看译文
关键词
decision making,learning (artificial intelligence),C4.5 algorithm,decision tree learning method,testing data set,training data set,weights decision tree,cost-sensitive learning,imbalanced data,weight gain ratio,weight information entropy,
AI 理解论文
溯源树
样例
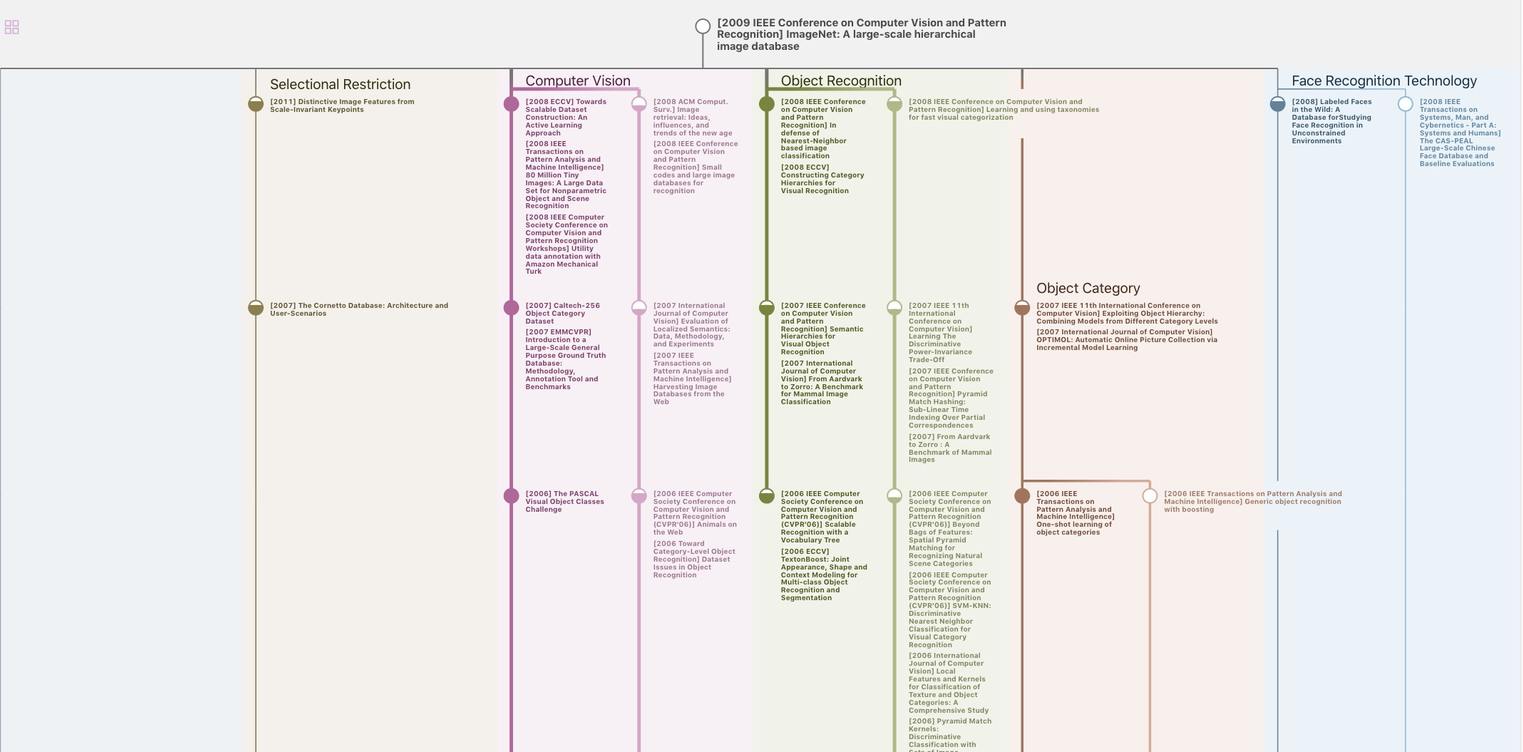
生成溯源树,研究论文发展脉络
Chat Paper
正在生成论文摘要