Improving Patient Opinion Mining through Multi-step Classification
TEXT, SPEECH AND DIALOGUE, PROCEEDINGS(2009)
摘要
Automatically tracking attitudes, feelings and reactions in on-line forums, blogs and news is a desirable instrument to support statistical analyses by companies, the government, and even individuals. In this paper, we present a novel approach to polarity classification of short text snippets, which takes into account the way data are naturally distributed into several topics in order to obtain better classification models for polarity. Our approach is multi-step, where in the initial step a standard topic classifier is learned from the data and the topic labels, and in the ensuing step several polarity classifiers, one per topic, are learned from the data and the polarity labels. We empirically show that our approach improves classification accuracy over a real-world dataset by over 10%, when compared against a standard single-step approach using the same feature sets. The approach is applicable whenever training material is available for building both topic and polarity learning models.
更多查看译文
关键词
improving patient opinion mining,novel approach,initial step,standard topic classifier,polarity classifier,multi-step classification,classification accuracy,topic label,automatically tracking attitude,polarity label,better classification model,standard single-step approach,opinion mining
AI 理解论文
溯源树
样例
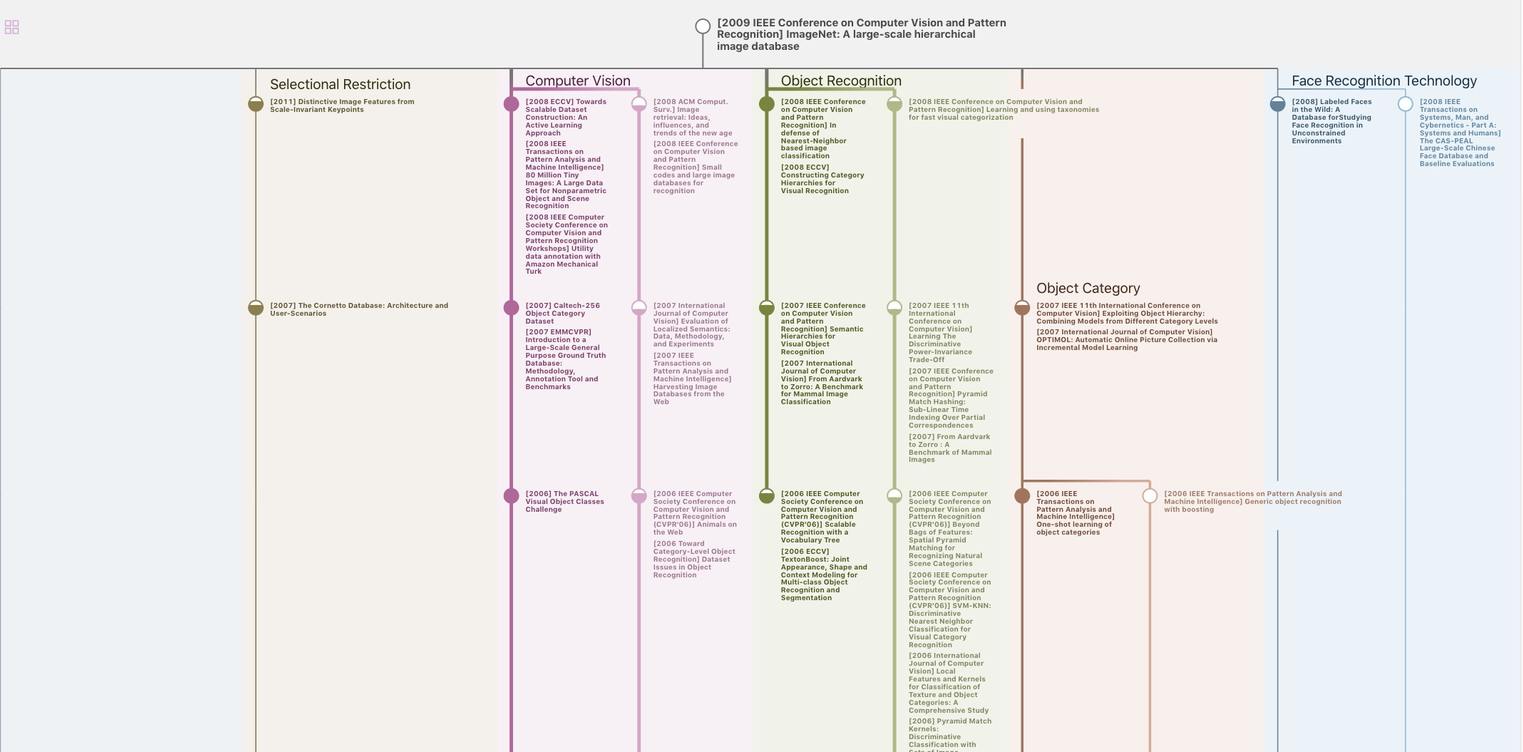
生成溯源树,研究论文发展脉络
Chat Paper
正在生成论文摘要