Multidimensional Similarity In-network Query for Large-Scale Sensor Networks
Taipei(2009)
摘要
The multidimensional similarity query, an essential query for information processing in sensor networks, has not received sufficient attention in the research community of sensor networks. In this paper, we study the multidimensional similarity query for large-scale sensor networks and propose a new algorithm called DIC (dimension reduction by Chebyshev polynomials). In DIC algorithm, the normalized Chebyshv coefficients are adopted as indexing and theoretic storage location of multidimensional data, and the multidimensional data are stored in the sensor nodes close to the theoretic location. A query bounding is estimated by using DIC algorithm, and query is executed inside a small zone. Inside the small zone, a new method of the itinerary-based query propagation and data aggregation is presented. The DIC algorithm does not require to preserve any index structure in sensor nodes, and also do not reply on any infrastructure structures distributed among the sensor nodes. We provide extensive experiments to evaluate the performance of the algorithm. The experimental results demonstrate that DIC can indeed enable efficient similarity queries.
更多查看译文
关键词
efficient similarity query,query bounding,dic algorithm,sensor network,essential query,small zone,multidimensional data,large-scale sensor network,large-scale sensor networks,multidimensional similarity in-network query,indexing,chebyshev approximation,multidimensional similarity query,itinerary-based query propagation,information processing,wireless sensor networks,theoretic storage location,normalized chebyshv coefficients,sensor node,dimension reduction by chebyshev polynomials,data aggregation,query processing,polynomials,routing,indexes,chebyshev polynomial,dimension reduction,multidimensional systems,accuracy,data mining
AI 理解论文
溯源树
样例
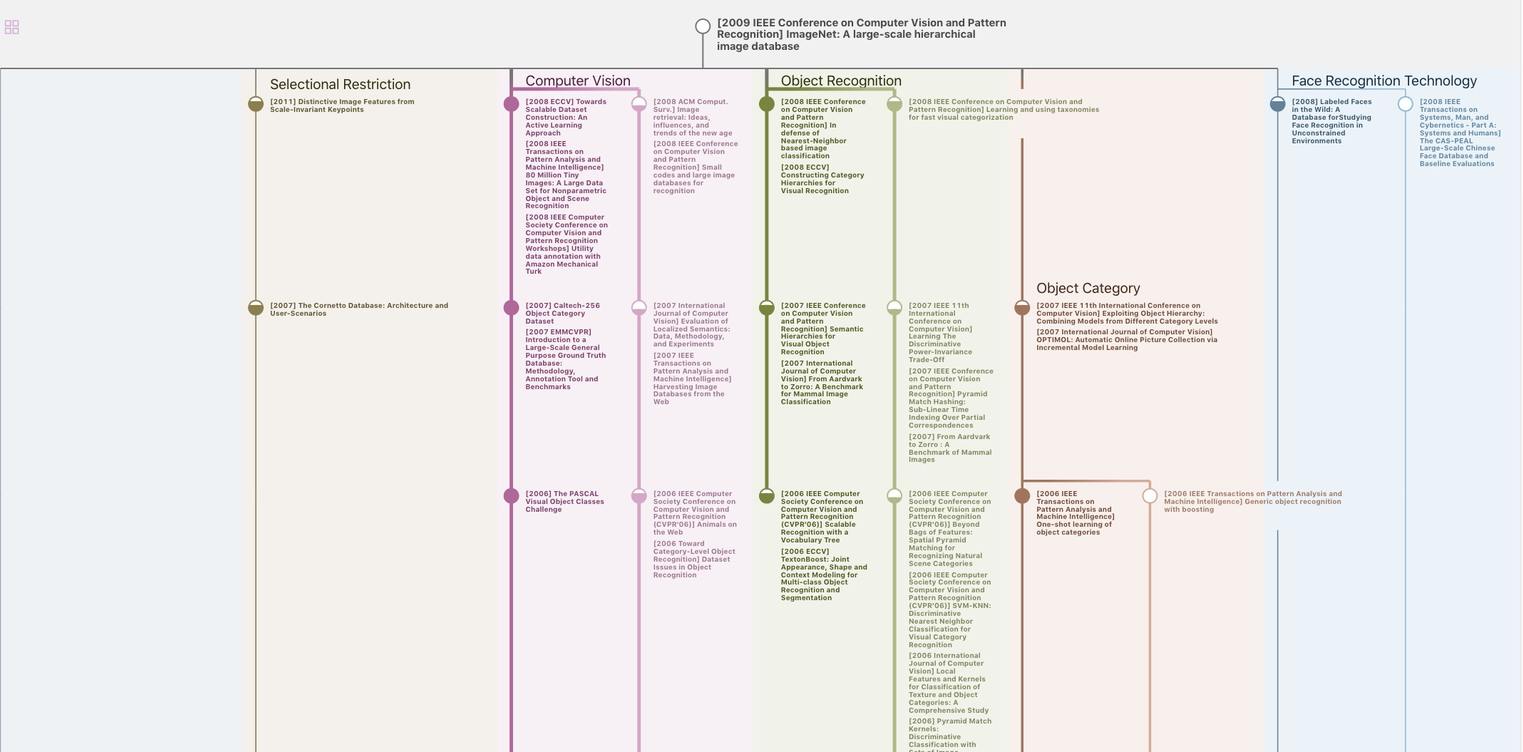
生成溯源树,研究论文发展脉络
Chat Paper
正在生成论文摘要