k-Anonymity via Clustering Domain Knowledge for Privacy Preservation
Fuzzy Systems and Knowledge Discovery, 2008. FSKD '08. Fifth International Conference(2008)
Abstract
Preservation of privacy in micro-data release is a challenging task in data mining. The k-anonymity method has attracted much attention of researchers. Quasi-identifier is a key concept in k-anonymity. The tuples whose quasi-identifiers have near effect on the sensitive attributes should be grouped to reduce information loss. The previous investigations ignored this point. This paper studies k-anonymity via clustering domain knowledge. The contributions include: (a) Constructing a weighted matrix based on domain knowledge and proposing measure methods. It carefully considers the effect between the quasi-identifiers and the sensitive attributes. (b) Developing a heuristic algorithm to achieve k-anonymity via clustering domain knowledge based on the measure methods. (c) Implementing the algorithm for privacy preservation, and (d) Experiments on real data demonstrate that the proposed k-anonymous methods decrease 30% information loss compared with basic k-anonymity.
MoreTranslated text
Key words
privacy preservation,sensitive attribute,quasi-identifiers,pattern clustering,basic k-anonymity,near effect,data privacy,clustering domain knowledge,heuristic algorithm,k-anonymity method,microdata release,data mining,paper studies k-anonymity,domain knowledge,weighted matrix,information loss,artificial neural networks,micro data,privacy,clustering algorithms
AI Read Science
Must-Reading Tree
Example
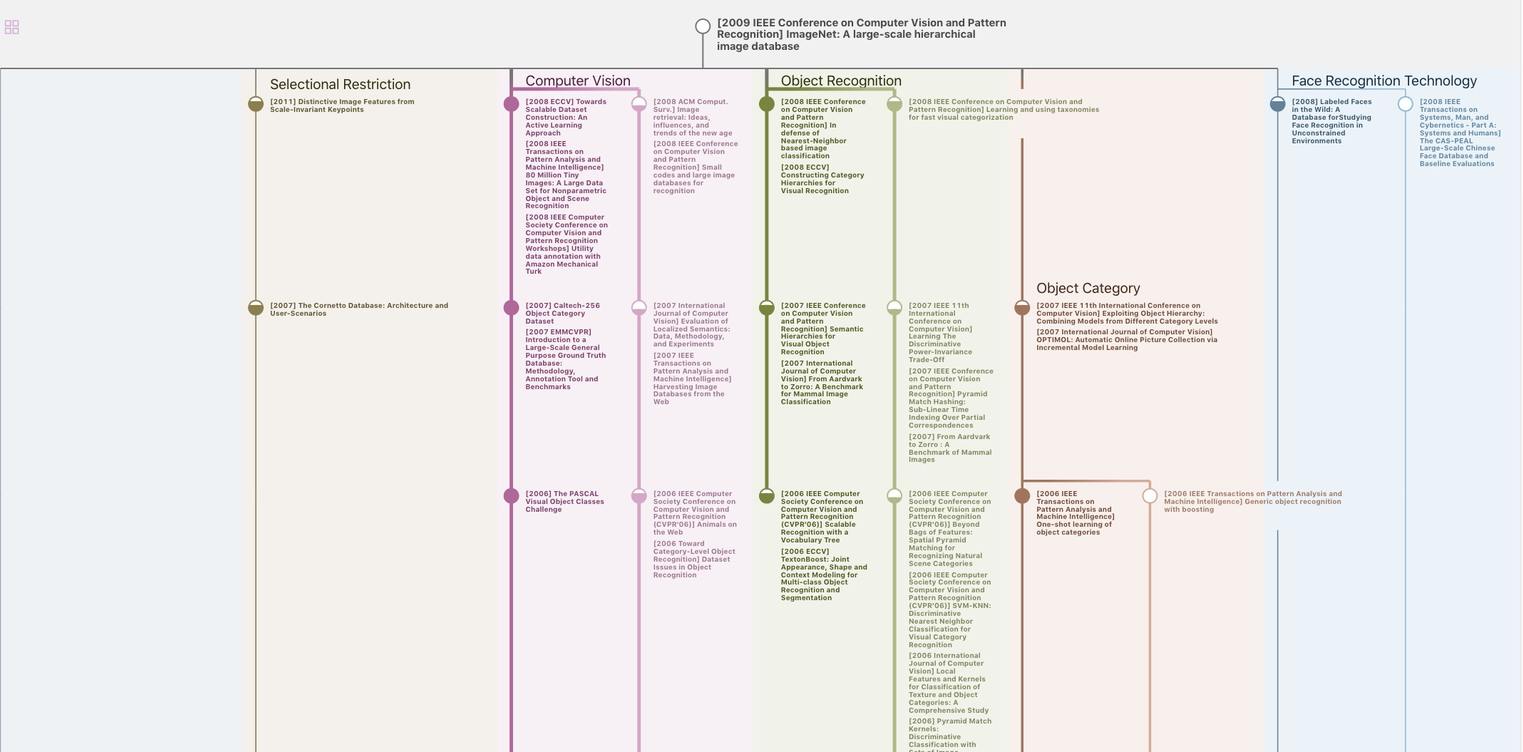
Generate MRT to find the research sequence of this paper
Chat Paper
Summary is being generated by the instructions you defined