Fuzzy Q-Map Algorithm for Reinforcement Learning
2006 INTERNATIONAL CONFERENCE ON COMPUTATIONAL INTELLIGENCE AND SECURITY, PTS 1 AND 2, PROCEEDINGS(2007)
摘要
In reinforcement learning, it is important to get nearly right answers early. Good prediction early can reduce the prediction error afterward and accelerate learning speed. We propose Fuzzy Q-Map, function approximation algorithm based on on-line fuzzy clustering in order to accelerate learning. Fuzzy Q-Map can handle the uncertainty owing to the absence of environment model. Appling membership function to reinforcement learning can reduce the prediction error and destructive interference phenomenon caused by changes of the distribution of training data. In order to evaluate fuzzy Q-Map's performance, we experimented on the mountain car problem and compared it with CMAC. CMAC achieves the prediction rate 80% from 250 training data, Fuzzy Q-Map learns faster and keep up the prediction rate 80% from 250 training data. Fuzzy Q-Map may be applied to the field of simulation that has uncertainty and complexity.
更多查看译文
关键词
fuzzy q-map algorithm,training data,function approximation algorithm,on-line fuzzy clustering,appling membership function,destructive interference phenomenon,fuzzy q-map,reinforcement learning,good prediction,prediction rate,prediction error,fuzzy clustering,membership function,fuzzy set theory,function approximation,learning artificial intelligence
AI 理解论文
溯源树
样例
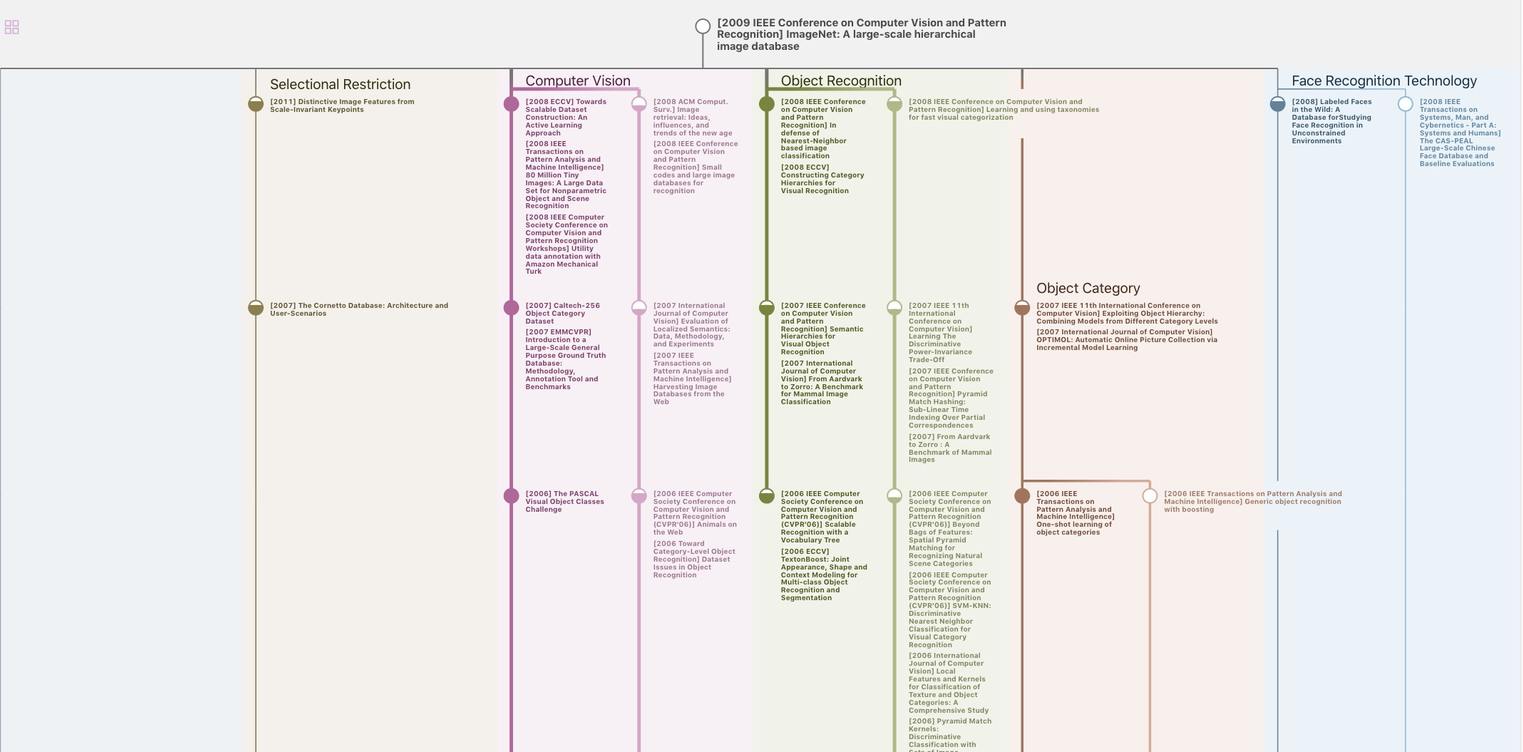
生成溯源树,研究论文发展脉络
Chat Paper
正在生成论文摘要