Large scale manifold transduction
Proceedings of the 25th international conference on Machine learning(2008)
摘要
We show how the regularizer of Transduc- tive Support Vector Machines (TSVM) can be trained by stochastic gradient descent for linear models and multi-layer architectures. The resulting methods can be trained on- line, have vastly superior training and test- ing speed to existing TSVM algorithms, can encode prior knowledge in the network archi- tecture, and obtain competitive error rates. We then go on to propose a natural gen- eralization of the TSVM loss function that takes into account neighborhood and mani- fold information directly, unifying the two- stage Low Density Separation method into a single criterion, and leading to state-of-the- art results.
更多查看译文
关键词
transductive support,tsvm loss function,competitive error rate,account neighborhood,large scale manifold transduction,manifold information,tsvm algorithm,natural generalization,vector machines,linear model,multi-layer architecture,loss function,stochastic gradient descent,error rate,layered architecture,support vector machine
AI 理解论文
溯源树
样例
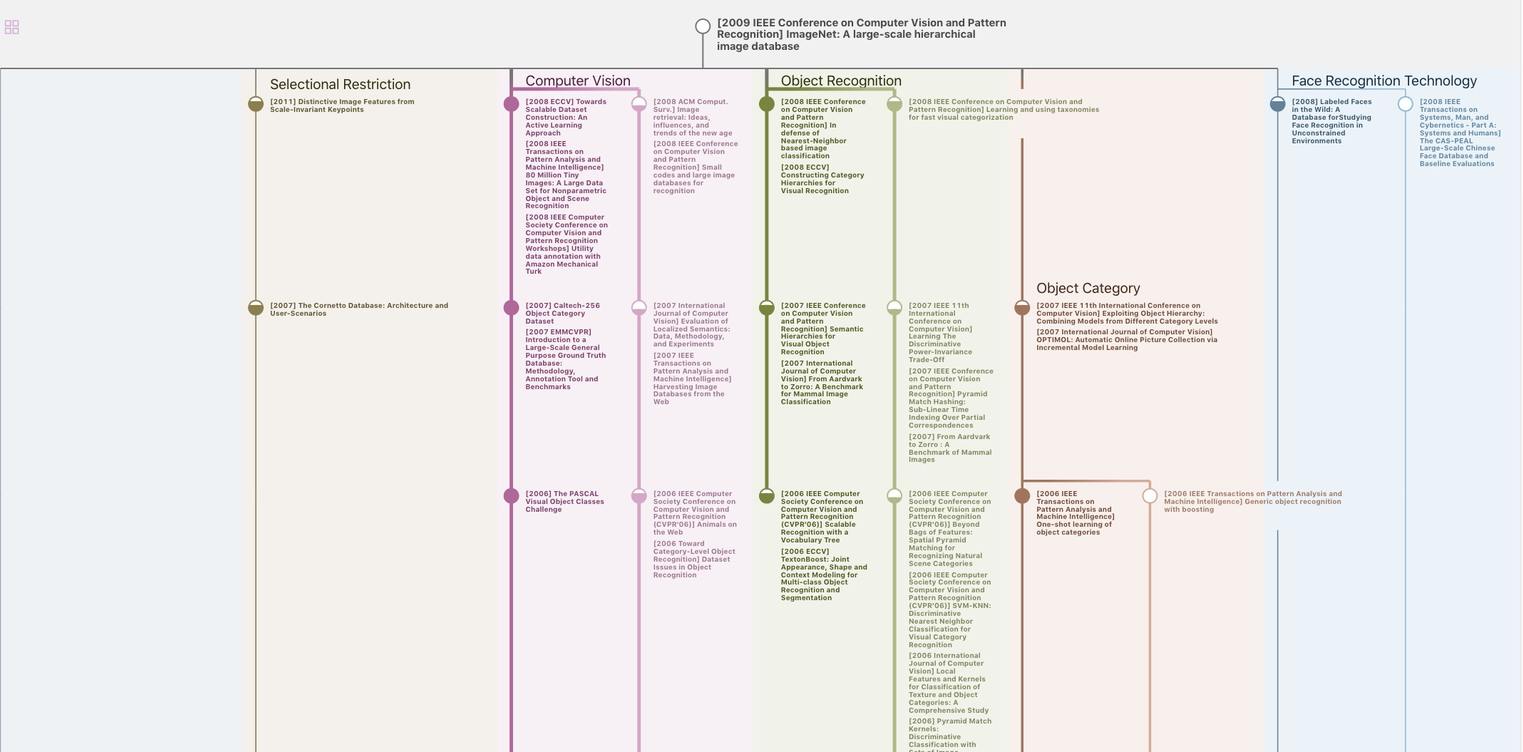
生成溯源树,研究论文发展脉络
Chat Paper
正在生成论文摘要