An empirical evaluation of supervised learning in high dimensions
Proceedings of the 25th international conference on Machine learning(2008)
摘要
In this paper we perform an empirical evaluation of supervised learning on high-dimensional data. We evaluate performance on three metrics: accuracy, AUC, and squared loss and study the effect of increasing dimensionality on the performance of the learning algorithms. Our findings are consistent with previous studies for problems of relatively low dimension, but suggest that as dimensionality increases the relative performance of the learning algorithms changes. To our surprise, the method that performs consistently well across all dimensions is random forests, followed by neural nets, boosted trees, and SVMs.
更多查看译文
关键词
high-dimensional data,previous study,relative performance,low dimension,algorithms change,high dimension,supervised learning,neural net,empirical evaluation,random forest,dimensionality increase,high dimensional data
AI 理解论文
溯源树
样例
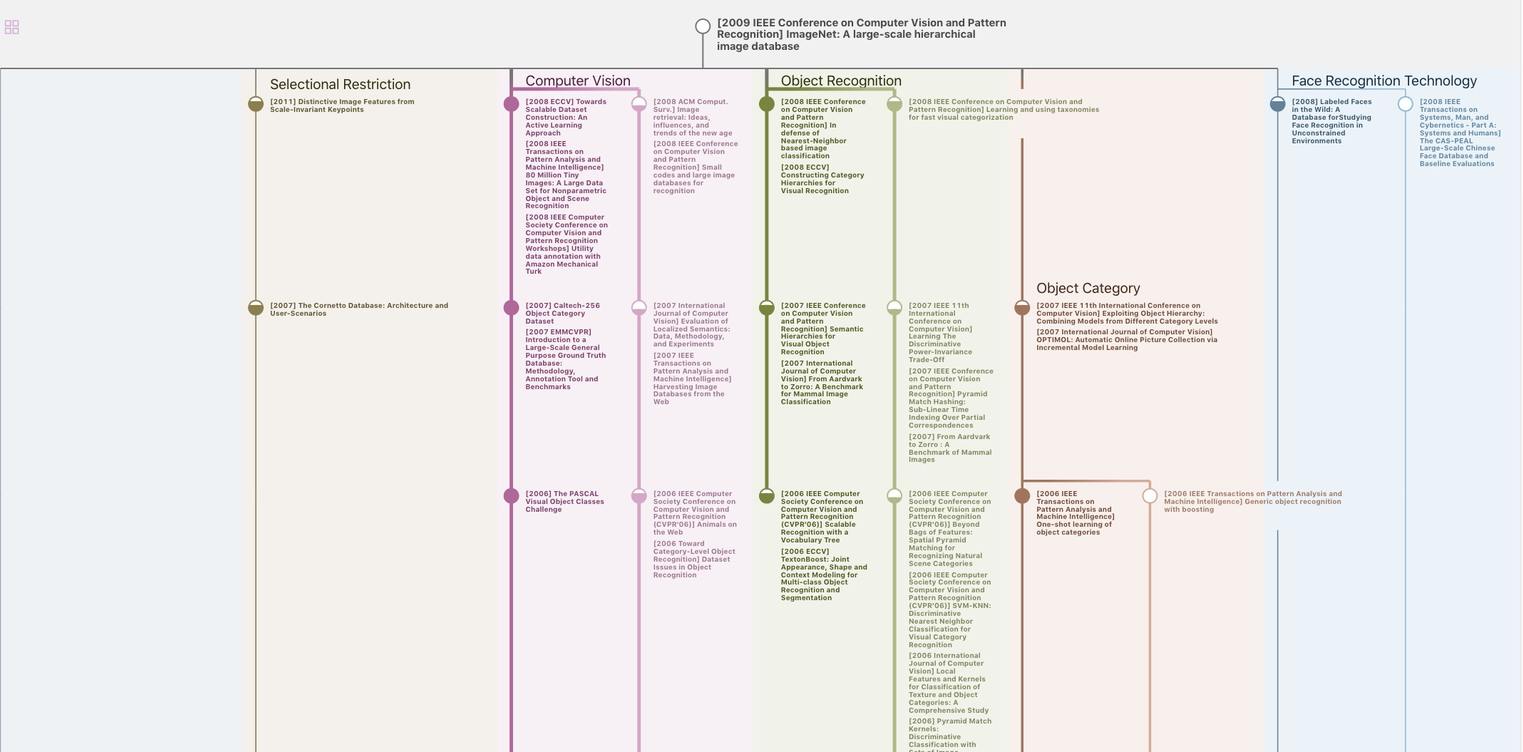
生成溯源树,研究论文发展脉络
Chat Paper
正在生成论文摘要