Combining Multi-Distributed Mixture Models and Bayesian Networks for Semi-Supervised Learning
ICMLA '07 Proceedings of the Sixth International Conference on Machine Learning and Applications(2007)
摘要
In many real world scenarios, mixture models have successfully been used for analyzing features in data ([11, 13, 21]). Usually, multivariate Gaussian distributions for continuous data ([2, 8, 4]) or Bayesian networks for nominal data ([15, 16]) are applied. In this paper, we combine both approaches in a family of Bayesian models for continuous data that are able to handle univariate as well as multivariate nodes, different types of distributions, e.g. Gaussian as well as Poisson distributed nodes, and dependencies between nodes. The models we introduce can be used for unsupervised, semi-supervised as well as for fully supervised learning tasks. We evaluate our models empirically on generated synthetic data and on public datasets thereby showing that they outperform classifiers such as SVMs and logistic regression on mixture data.
更多查看译文
关键词
gaussian distribution,gaussian processes,mixture model,bayesian networks,logistic regression,learning artificial intelligence,bayesian model,bayesian network,poisson distribution,synthetic data,semi supervised learning,supervised learning
AI 理解论文
溯源树
样例
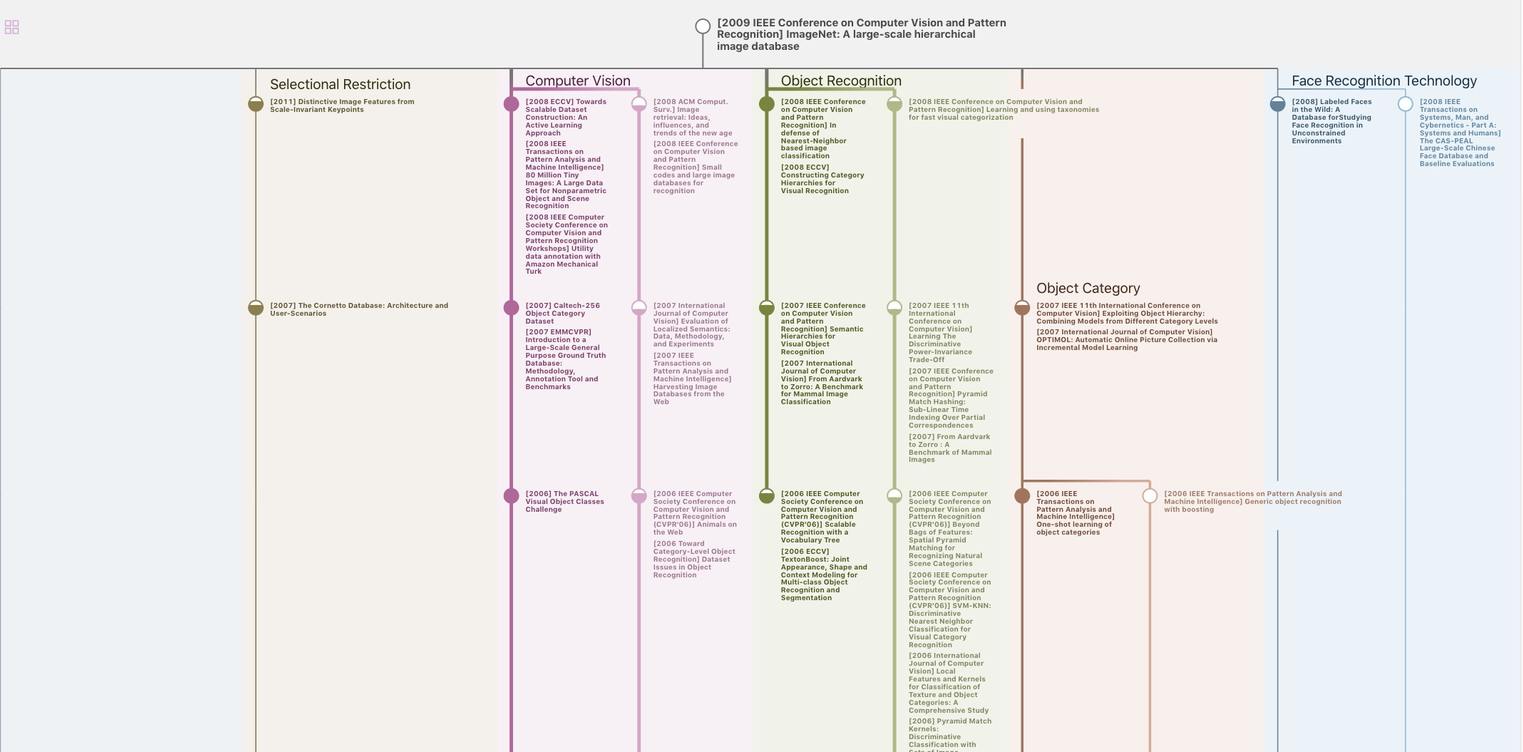
生成溯源树,研究论文发展脉络
Chat Paper
正在生成论文摘要