Improving Selection Methods For Evolutionary Algorithms By Clustering
ICNC 2007: THIRD INTERNATIONAL CONFERENCE ON NATURAL COMPUTATION, VOL 3, PROCEEDINGS(2007)
摘要
This study applies clustering in population selection to improve the efficiency of evolutionary algorithms. The main contributions include: (a) Proposes a novel selection framework that uses the number of clusters for a population as the measurement the population diversity. (b) Proposes clustering-ranking selection, an instance Of this framework, and discusses its mathematical principle by PD-SP equation. (c) Gives experiments over CLPSO (Comprehensive Learning Particle Swarm Optimization). Experiment result shows that the proposed selection method outperforms canonical exponential ranking on all the sixteen-benchmark functions for both 10-D and 30-D problem except a function for 30-D problem.
更多查看译文
关键词
30-D problem,novel selection framework,population selection,proposed selection method,population diversity,Comprehensive Learning Particle Swarm,PD-SP equation,canonical exponential ranking,evolutionary algorithm,experiment result,Evolutionary Algorithms,Improving Selection Methods
AI 理解论文
溯源树
样例
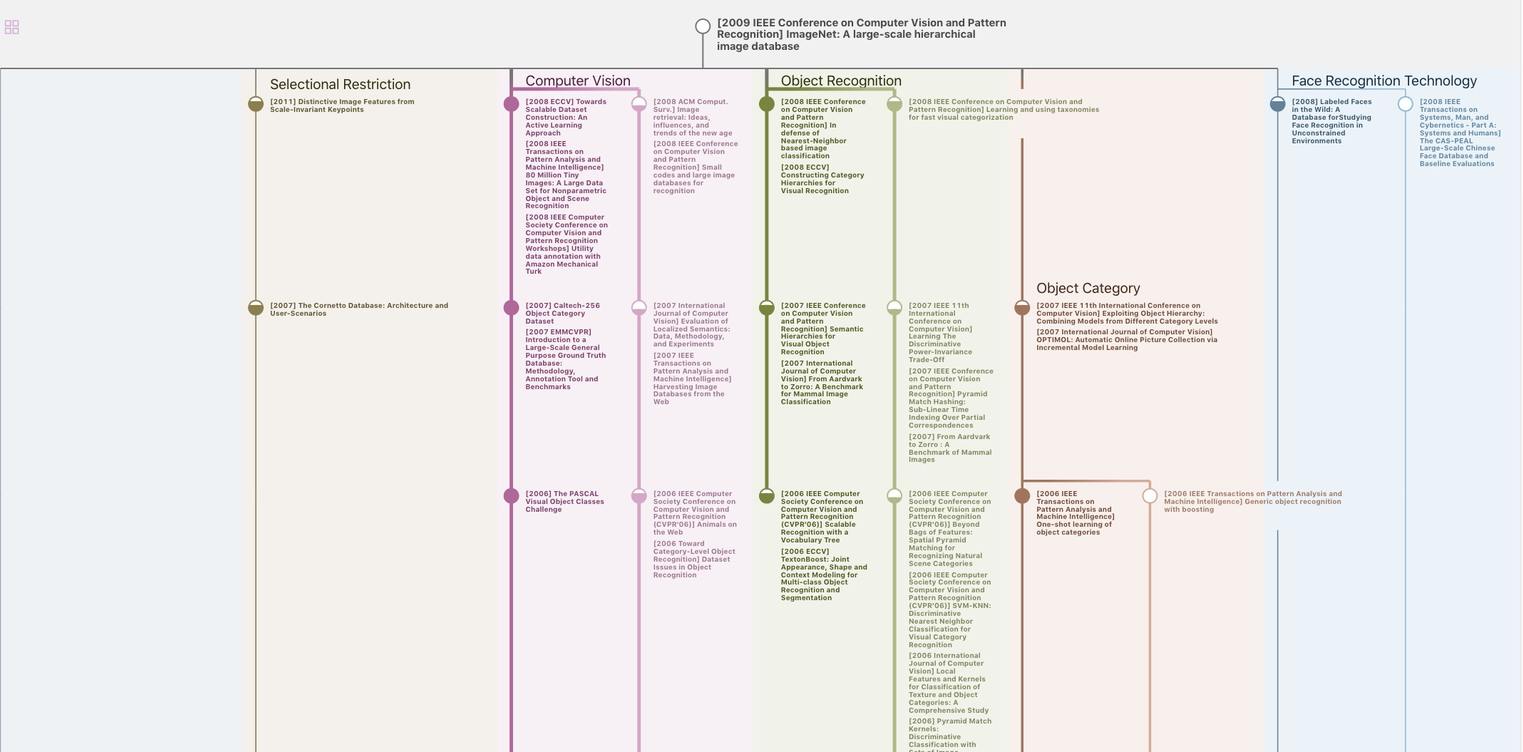
生成溯源树,研究论文发展脉络
Chat Paper
正在生成论文摘要