An online support vector machine for abnormal events detection
Signal Processing(2006)
摘要
The ability to detect online abnormal events in signals is essential in many real-world signal processing applications. Previous algorithms require an explicit signal statistical model, and interpret abnormal events as statistical model abrupt changes. Corresponding implementation relies on maximum likelihood or on Bayes estimation theory with generally excellent performance. However, there are numerous cases where a robust and tractable model cannot be obtained, and model-free approaches need to be considered. In this paper, we investigate a machine learning, descriptor-based approach that does not require an explicit descriptors statistical model, based on support vector novelty detection. A sequential optimization algorithm is introduced. Theoretical considerations as well as simulations on real signals demonstrate its practical efficiency.
更多查看译文
关键词
real signal,abnormality detection,abnormal events detection,bayes estimation theory,explicit signal,abnormal event,sequential optimization,real-world signal processing application,online support vector machine,audio thump detection.,support vector machines,online abnormal event,tractable model,gearbox fault detection,statistical model,abrupt change,explicit descriptors,fault detection,support vector,machine learning,support vector machine,signal processing,maximum likelihood
AI 理解论文
溯源树
样例
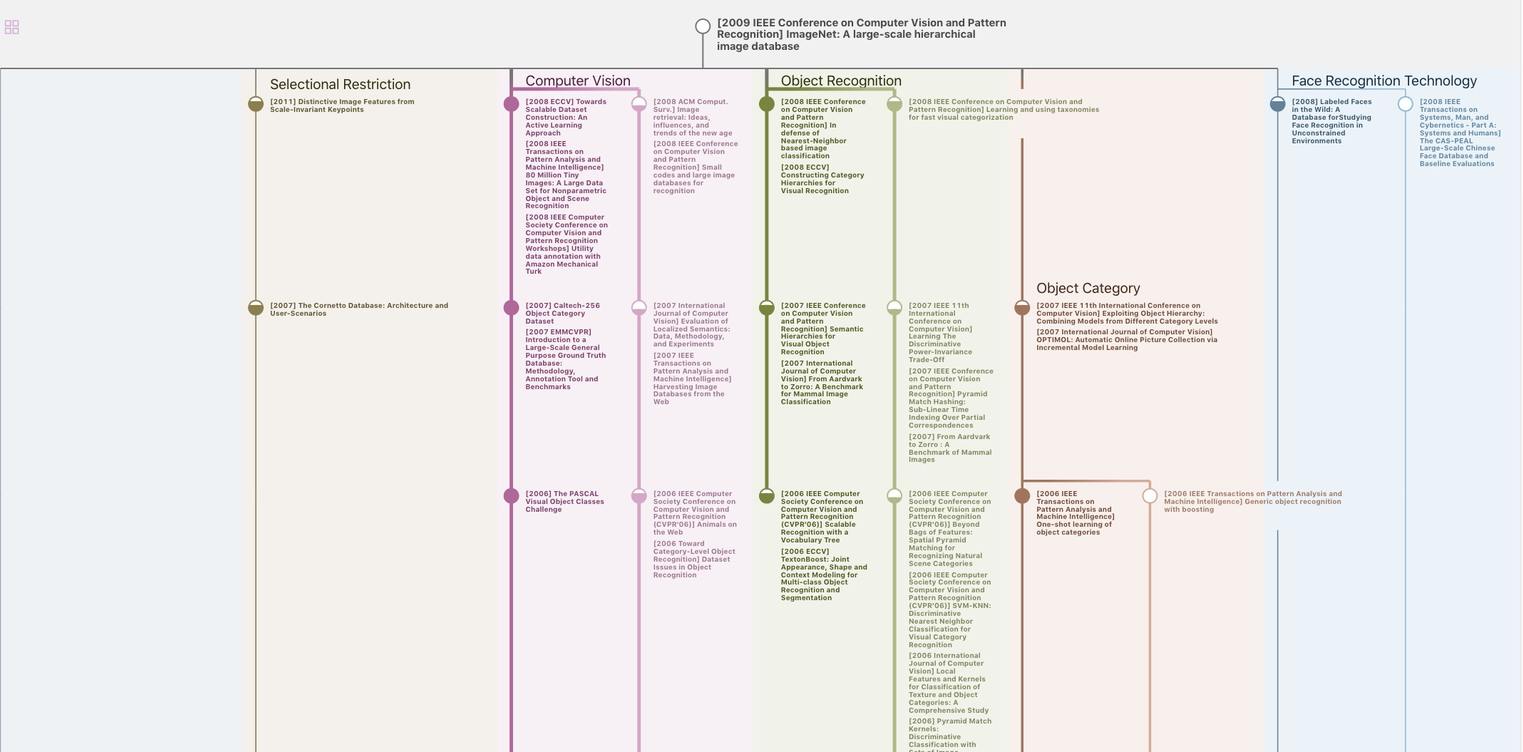
生成溯源树,研究论文发展脉络
Chat Paper
正在生成论文摘要