Explanation-Augmented SVM: an approach to incorporating domain knowledge into SVM learning
ICML '05 Proceedings of the 22nd international conference on Machine learning(2005)
摘要
We introduce a novel approach to incorporating domain knowledge into Support Vector Machines to improve their example efficiency. Domain knowledge is used in an Explanation Based Learning fashion to build justifications or explanations for why the training examples are assigned their given class labels. Explanations bias the large margin classifier through the interaction of training examples and domain knowledge. We develop a new learning algorithm for this Explanation-Augmented SVM (EA-SVM). It naturally extends to imperfect knowledge, a stumbling block to conventional EBL. Experimental results confirm desirable properties predicted by the analysis and demonstrate the approach on three domains.
更多查看译文
关键词
training example,support vector machines,novel approach,svm learning,conventional ebl,explanations bias,imperfect knowledge,desirable property,class label,domain knowledge,explanation-augmented svm,support vector machine
AI 理解论文
溯源树
样例
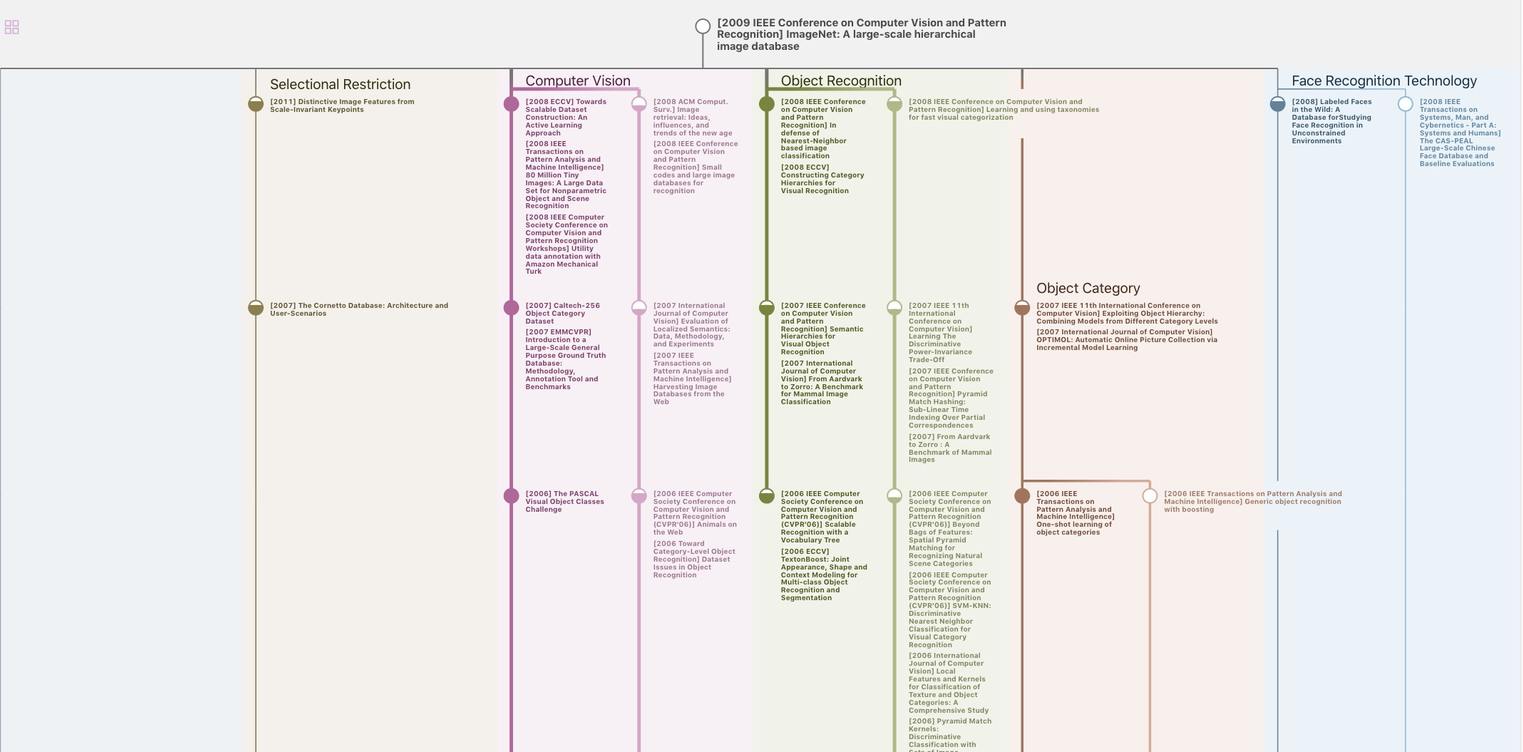
生成溯源树,研究论文发展脉络
Chat Paper
正在生成论文摘要