Predicting GPCR--G-protein coupling using hidden Markov models
BIOINFORMATICS(2004)
摘要
Motivation: Determining the coupling specificity of G-protein coupled receptors (GPCRs) is important for understanding the biology of this class of pharmacologically important proteins. Currently available in silico methods for predicting GPCR--G-protein coupling specificity have high error rate. Method: We introduce a new approach for creating hidden Markov models (HMMs) based on a first guess about the importance of various residues. We call these knowledge restricted HMMs to emphasize the fact that the state space of the HMM is restricted by the application of a priori knowledge. Specifically, we use only those amino acid residues of GPCRs which are likely to interact with G-proteins, namely those that are predicted to be in the intra-cellular loops. Furthermore, we concatenate these predicted loops into one sequence rather than considering them as four disparate units. This reduces the HMM state space by drastically decreasing the sequence length. Results: Our knowledge restricted HMM based method to predict GPCR--G-protein coupling specificity has an error rate of Availability: Academic users can get the data set mentioned herein and HMMs from the authors.
更多查看译文
关键词
state space,hidden markov model,amino acid,g protein coupled receptor,error rate,a priori knowledge,g protein
AI 理解论文
溯源树
样例
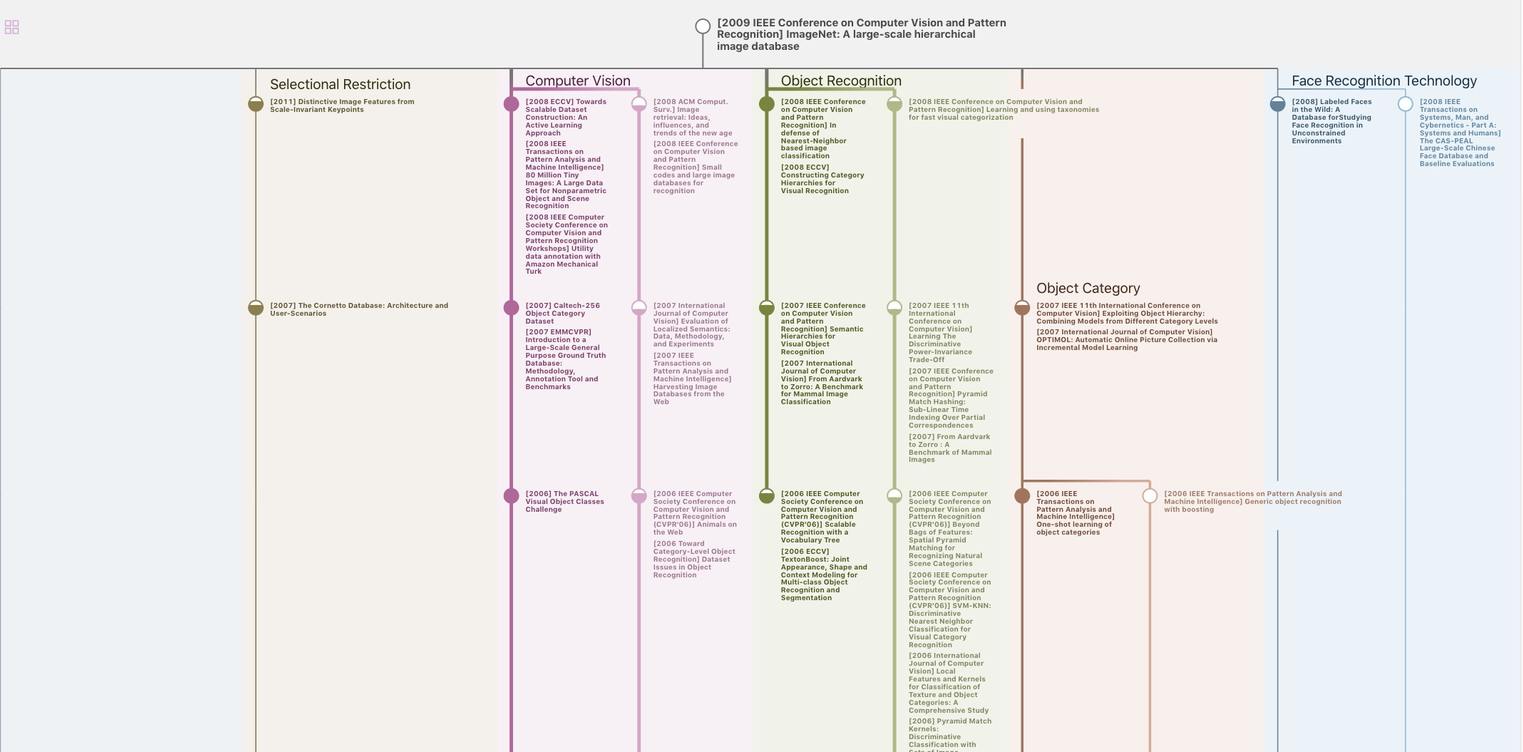
生成溯源树,研究论文发展脉络
Chat Paper
正在生成论文摘要