Superior Polymeric Gas Separation Membrane Designed by Explainable Graph Machine Learning
arxiv(2024)
摘要
Gas separation using polymer membranes promises to dramatically drive down
the energy, carbon, and water intensity of traditional thermally driven
separation, but developing the membrane materials is challenging. Here, we
demonstrate a novel graph machine learning (ML) strategy to guide the
experimental discovery of synthesizable polymer membranes with performances
simultaneously exceeding the empirical upper bounds in multiple industrially
important gas separation tasks. Two predicted candidates are synthesized and
experimentally validated to perform beyond the upper bounds for multiple gas
pairs (O2/N2, H2/CH4, and H2/N2). Notably, the O2/N2 separation selectivity is
1.6-6.7 times higher than existing polymer membranes. The molecular origin of
the high performance is revealed by combining the inherent interpretability of
our ML model, experimental characterization, and molecule-level simulation. Our
study presents a unique explainable ML-experiment combination to tackle
challenging energy material design problems in general, and the discovered
polymers are beneficial for industrial gas separation.
更多查看译文
AI 理解论文
溯源树
样例
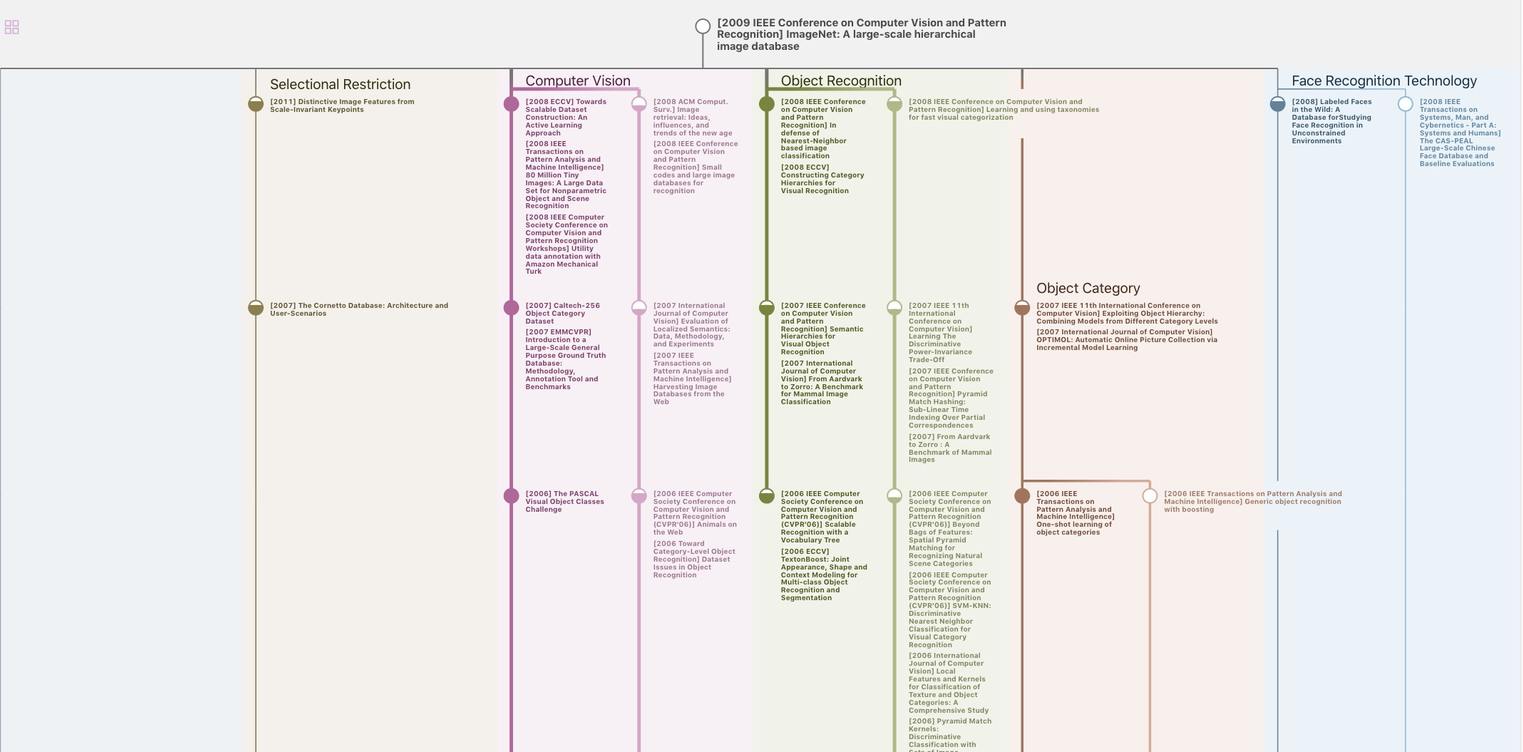
生成溯源树,研究论文发展脉络
Chat Paper
正在生成论文摘要