A Unified Meta-learning Framework for Fair Ranking with Curriculum Learning
IEEE Transactions on Knowledge and Data Engineering(2024)
摘要
In recent information retrieval systems, it is observed that the datasets used to train machine learning models can be biased, leading to systematic discrimination against certain demographic groups, which means the ranking utility of specific groups is often lower than others in a biased dataset. Training models on these datasets will further decrease the exposure of the minority groups. To address this problem, we propose a Meta Curriculum-based Fair Ranking framework (MCFR) which could alleviate the data bias issue through the weighted loss using gradient-based learning to learn. Specifically, we optimize a meta learner from a sampled dataset (
meta-dataset
), and meanwhile train a ranking model on the whole (
biased
) dataset. The meta-dataset is sampled with a curriculum learning scheduler to guide the meta learner's training to gradually mitigate the skewness towards biased attributes. The meta learner serves as a weighting function to make the ranking loss focus more on the minority group. We formulate the proposed MCFR as a bilevel optimization problem and solve it using gradients through gradients. Extensive experiments on real-world datasets demonstrate that our approach can be used as a generic framework to work with various ranking losses and fairness metrics.
更多查看译文
关键词
Fairness-aware Search,Meta-learning,Learning-to-rank,Curriculum Learning
AI 理解论文
溯源树
样例
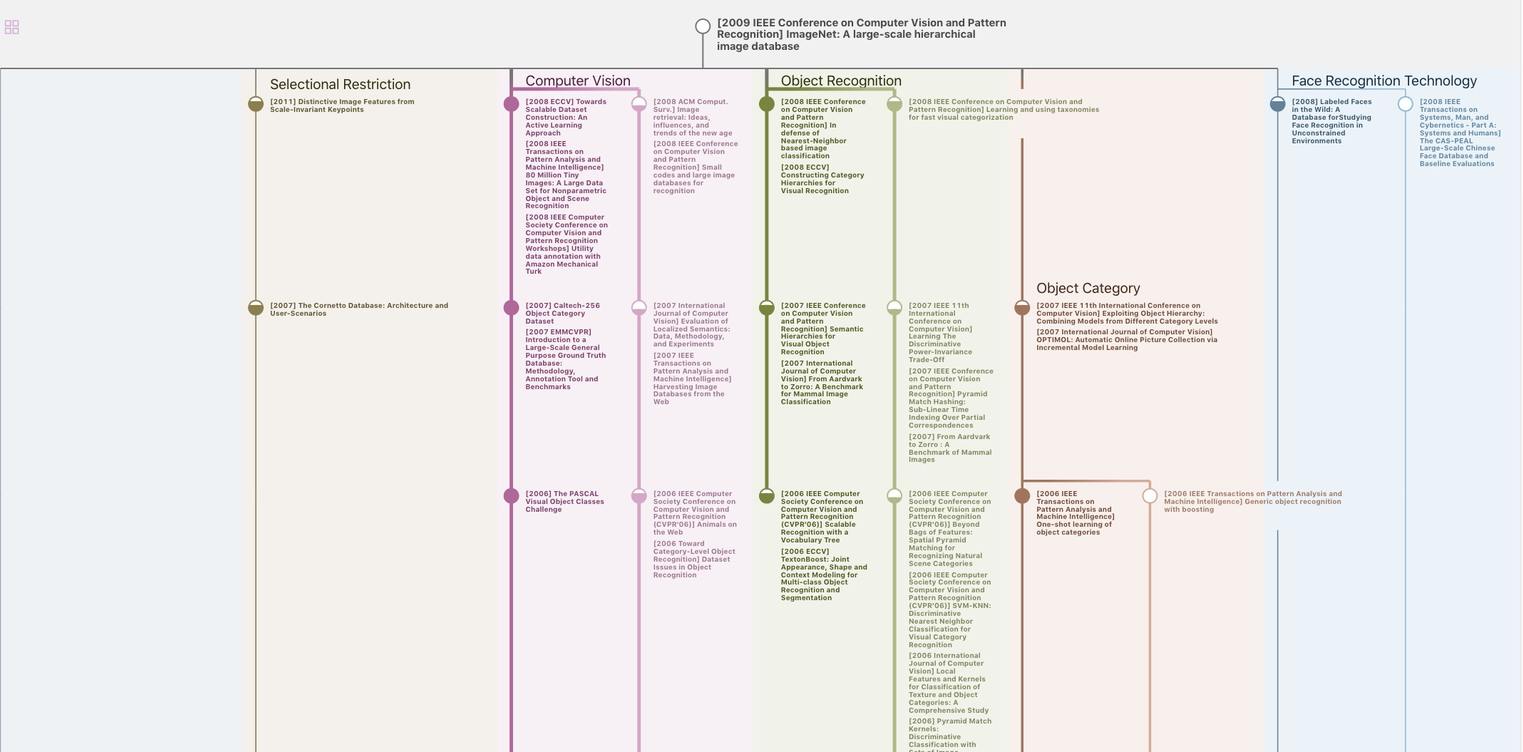
生成溯源树,研究论文发展脉络
Chat Paper
正在生成论文摘要