OAG-Bench: A Human-Curated Benchmark for Academic Graph Mining
CoRR(2024)
摘要
With the rapid proliferation of scientific literature, versatile academic
knowledge services increasingly rely on comprehensive academic graph mining.
Despite the availability of public academic graphs, benchmarks, and datasets,
these resources often fall short in multi-aspect and fine-grained annotations,
are constrained to specific task types and domains, or lack underlying real
academic graphs. In this paper, we present OAG-Bench, a comprehensive,
multi-aspect, and fine-grained human-curated benchmark based on the Open
Academic Graph (OAG). OAG-Bench covers 10 tasks, 20 datasets, 70+ baselines,
and 120+ experimental results to date. We propose new data annotation
strategies for certain tasks and offer a suite of data pre-processing codes,
algorithm implementations, and standardized evaluation protocols to facilitate
academic graph mining. Extensive experiments reveal that even advanced
algorithms like large language models (LLMs) encounter difficulties in
addressing key challenges in certain tasks, such as paper source tracing and
scholar profiling. We also introduce the Open Academic Graph Challenge
(OAG-Challenge) to encourage community input and sharing. We envisage that
OAG-Bench can serve as a common ground for the community to evaluate and
compare algorithms in academic graph mining, thereby accelerating algorithm
development and advancement in this field. OAG-Bench is accessible at
https://www.aminer.cn/data/.
更多查看译文
AI 理解论文
溯源树
样例
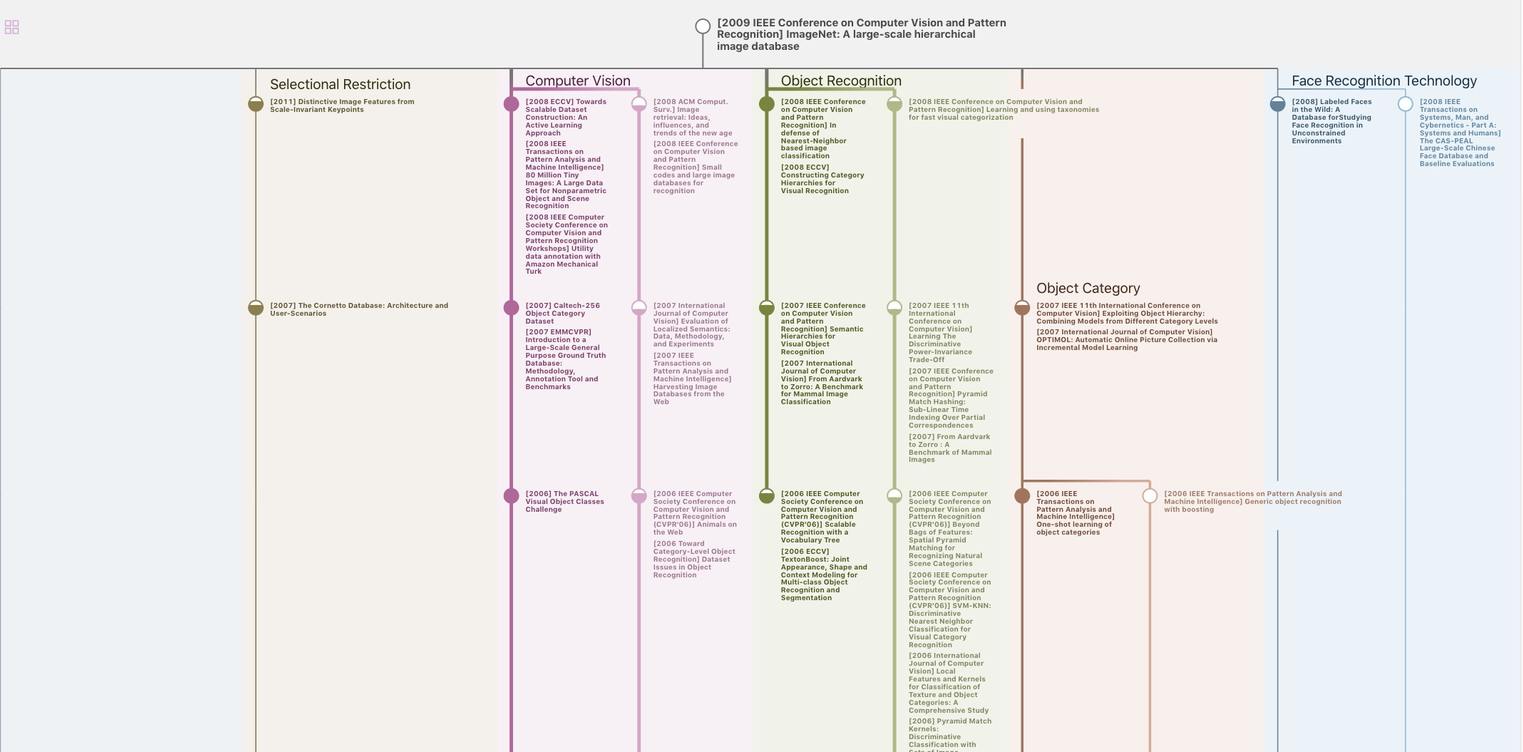
生成溯源树,研究论文发展脉络
Chat Paper
正在生成论文摘要