TriSampler: A Better Negative Sampling Principle for Dense Retrieval
AAAI 2024(2024)
摘要
Negative sampling stands as a pivotal technique in dense retrieval, essential for training effective retrieval models and significantly impacting retrieval performance. While existing negative sampling methods have made commendable progress by leveraging hard negatives, a comprehensive guiding principle for constructing negative candidates and designing negative sampling distributions is still lacking. To bridge this gap, we embark on a theoretical analysis of negative sampling in dense retrieval. This exploration culminates in the unveiling of the quasi-triangular principle, a novel framework that elucidates the triangular-like interplay between query, positive document, and negative document. Fueled by this guiding principle, we introduce TriSampler, a straightforward yet highly effective negative sampling method. The keypoint of TriSampler lies in its ability to selectively sample more informative negatives within a prescribed constrained region. Experimental evaluation show that TriSampler consistently attains superior retrieval performance across a diverse of representative retrieval models.
更多查看译文
关键词
DMKM: Conversational Systems for Recommendation & Retrieval
AI 理解论文
溯源树
样例
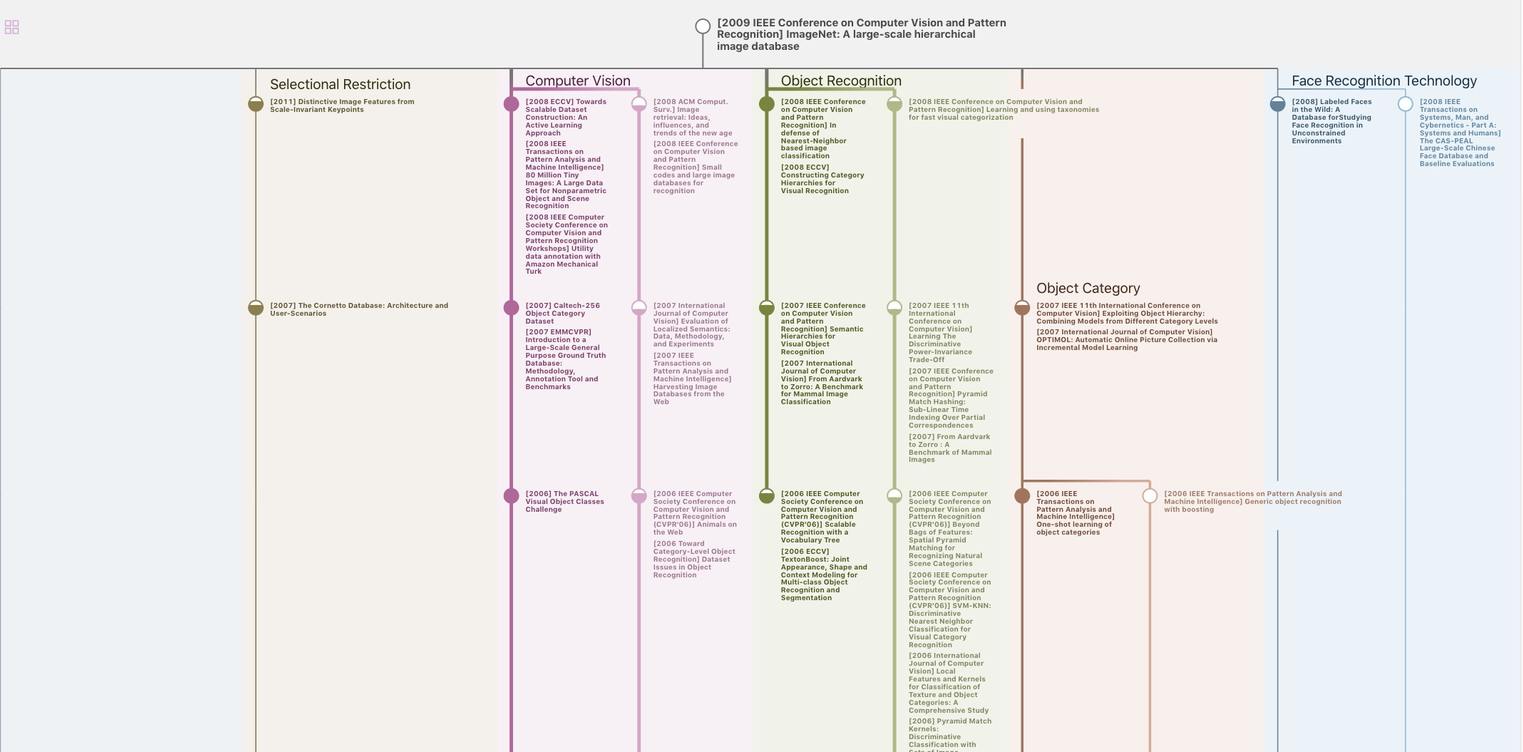
生成溯源树,研究论文发展脉络
Chat Paper
正在生成论文摘要