Breaking the curse of dimensional collapse in graph contrastive learning: A whitening perspective
INFORMATION SCIENCES(2024)
摘要
Dimensional collapse in graph contrastive learning (GCL) confines node embeddings to their lower-dimensional subspace, diminishing their distinguishability. However, the causes and solutions of this curse remain relatively underexplored. In statistics, whitening presents a powerful tool to eliminate correlations among multiple variables. This motivates us to relieve the dimensional collapse of GCL from a whitening perspective. In this paper, we propose an intuitive analysis suggesting that high similarity scores of node embeddings may cause dimensional collapse, providing more evidence for its presence. Considering the success of whitening in statistics, we introduce a new plug-and-play module called the Whitening Graph Contrastive Learning (WGCL) to address the dimensional collapse issue in existing GCL methods. WGCL plugin standardises the covariance matrices of dimensions, eliminating correlations among node embeddings' dimensions. Additionally, we enhance the conventional GCL training objective by introducing a mutual information maximisation loss between input features and node embeddings to maintain information capacity. Our experiments demonstrate that WGCL effectively addresses dimensional collapse, leading to an average improvement of 0.93% (up to 2.0%) in classification accuracy across three GCL backbones on nine widely-used datasets. The code to reproduce the experiments is available at https://github .com /acboyty /WGCL.
更多查看译文
关键词
Graph contrastive learning,Dimensional collapse,Whitening
AI 理解论文
溯源树
样例
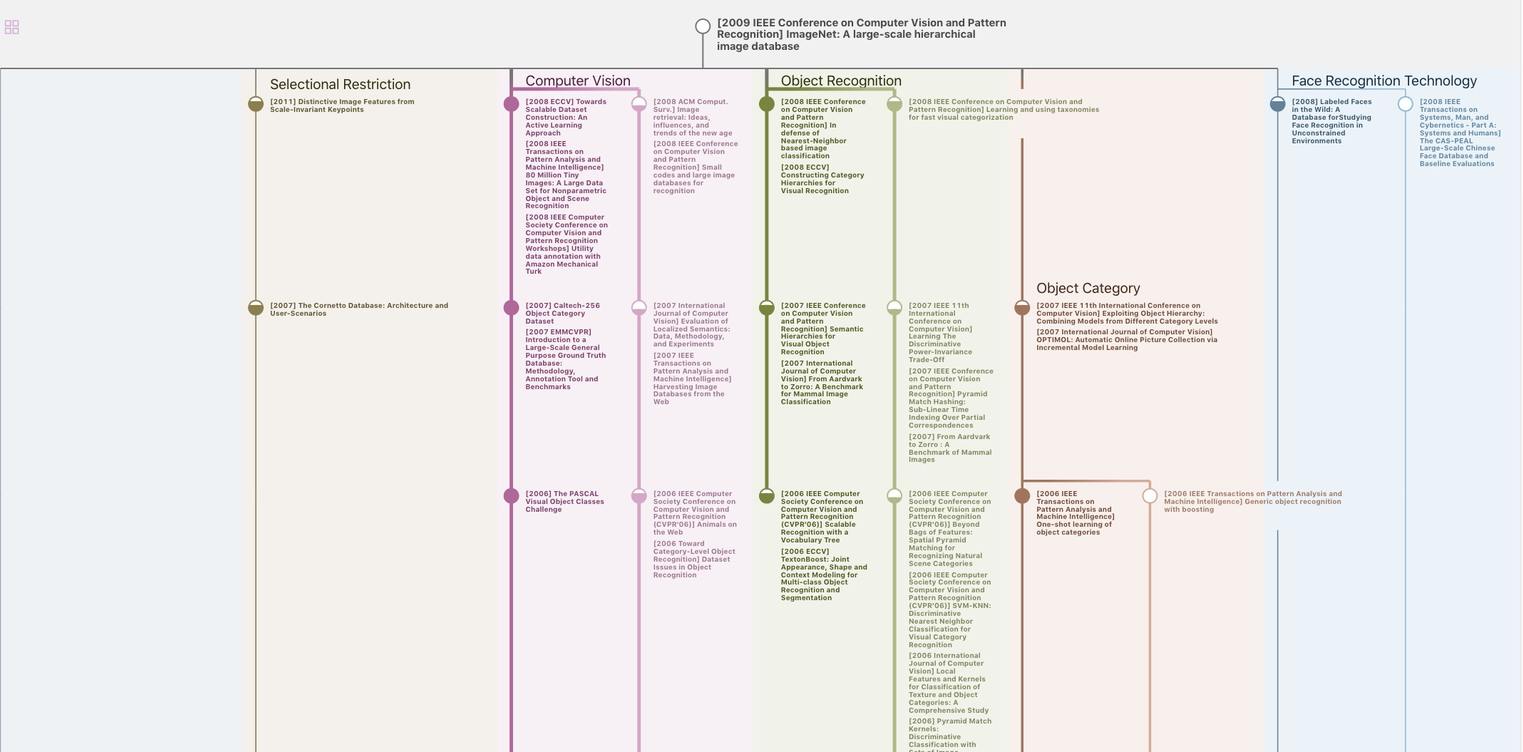
生成溯源树,研究论文发展脉络
Chat Paper
正在生成论文摘要