An Empirical Study of Selection Bias in Pinterest Ads Retrieval.
KDD(2023)
摘要
Data selection bias has been a long-lasting challenge in the machine learning domain, especially in multi-stage recommendation systems, where the distribution of labeled items for model training is very different from that of the actual candidates during inference time. This distribution shift is even more prominent in the context of online advertising where the user base is diverse and the platform contains a wide range of contents. In this paper, we first investigate the data selection bias in the upper funnel (Ads Retrieval) of Pinterest's multi-cascade ads ranking system. We then conduct comprehensive experiments to assess the performance of various state-of-the-art methods, including transfer learning, adversarial learning, and unsupervised domain adaptation. Moreover, we further introduce some modifications into the unsupervised domain adaptation and evaluate the performance of different variants of this modified method. Our online A/B experiments show that the modified version of unsupervised domain adaptation (MUDA) could provide the largest improvements to the performance of Pinterest's advertisement ranking system compared with other methods and the one used in current production.
更多查看译文
关键词
Selection Bias,Pre-Ranking System,Online Advertising
AI 理解论文
溯源树
样例
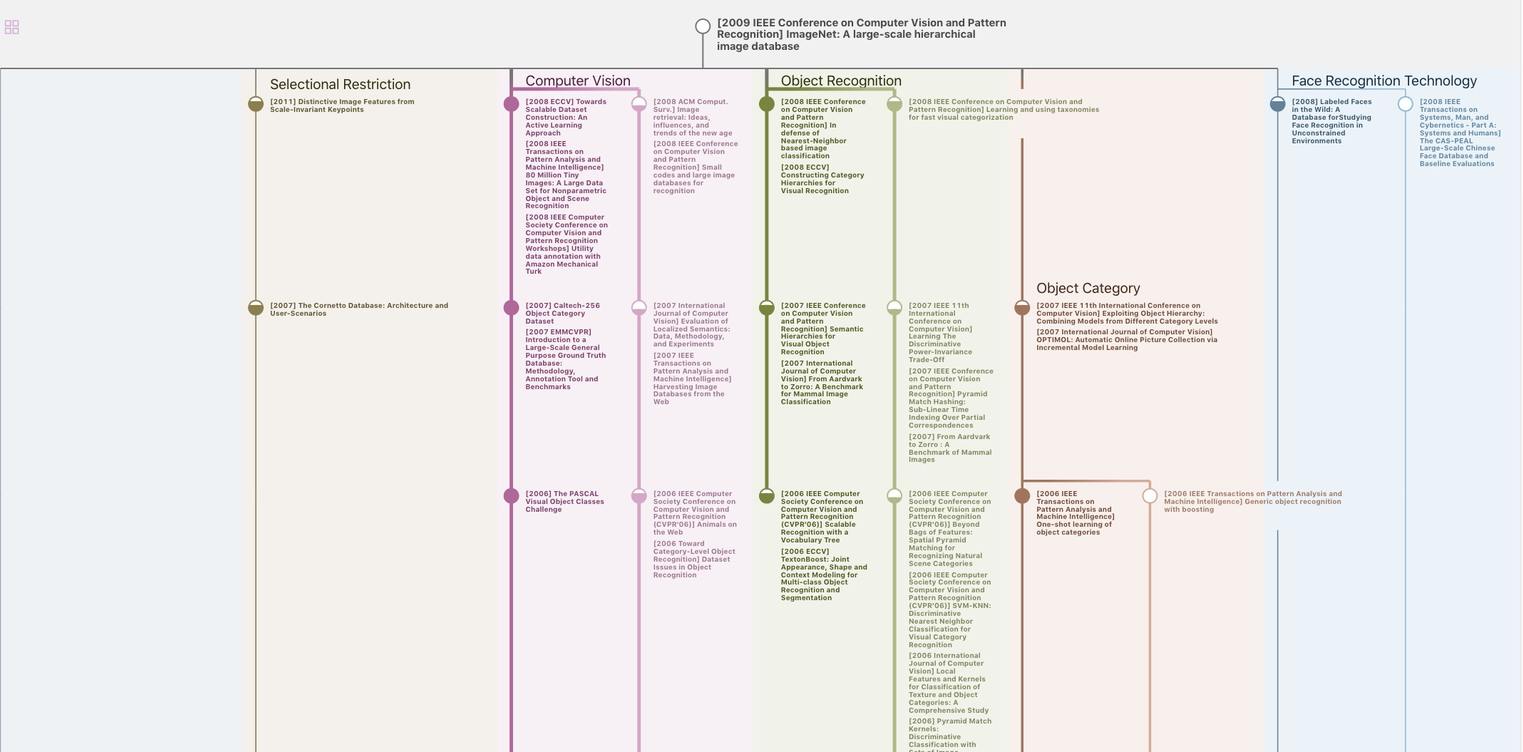
生成溯源树,研究论文发展脉络
Chat Paper
正在生成论文摘要