ApeGNN: Node-Wise Adaptive Aggregation in GNNs for Recommendation
WWW 2023(2023)
摘要
In recent years, graph neural networks (GNNs) have made great progress in recommendation. The core mechanism of GNNs-based recommender system is to iteratively aggregate neighboring information on the user-item interaction graph. However, existing GNNs treat users and items equally and cannot distinguish diverse local patterns of each node, which makes them suboptimal in the recommendation scenario. To resolve this challenge, we present a node-wise adaptive graph neural network framework ApeGNN. ApeGNN develops a node-wise adaptive diffusion mechanism for information aggregation, in which each node is enabled to adaptively decide its diffusion weights based on the local structure (e.g., degree). We perform experiments on six widely-used recommendation datasets. The experimental results show that the proposed ApeGNN is superior to the most advanced GNN-based recommender methods (up to 48.94%), demonstrating the effectiveness of node-wise adaptive aggregation.
更多查看译文
AI 理解论文
溯源树
样例
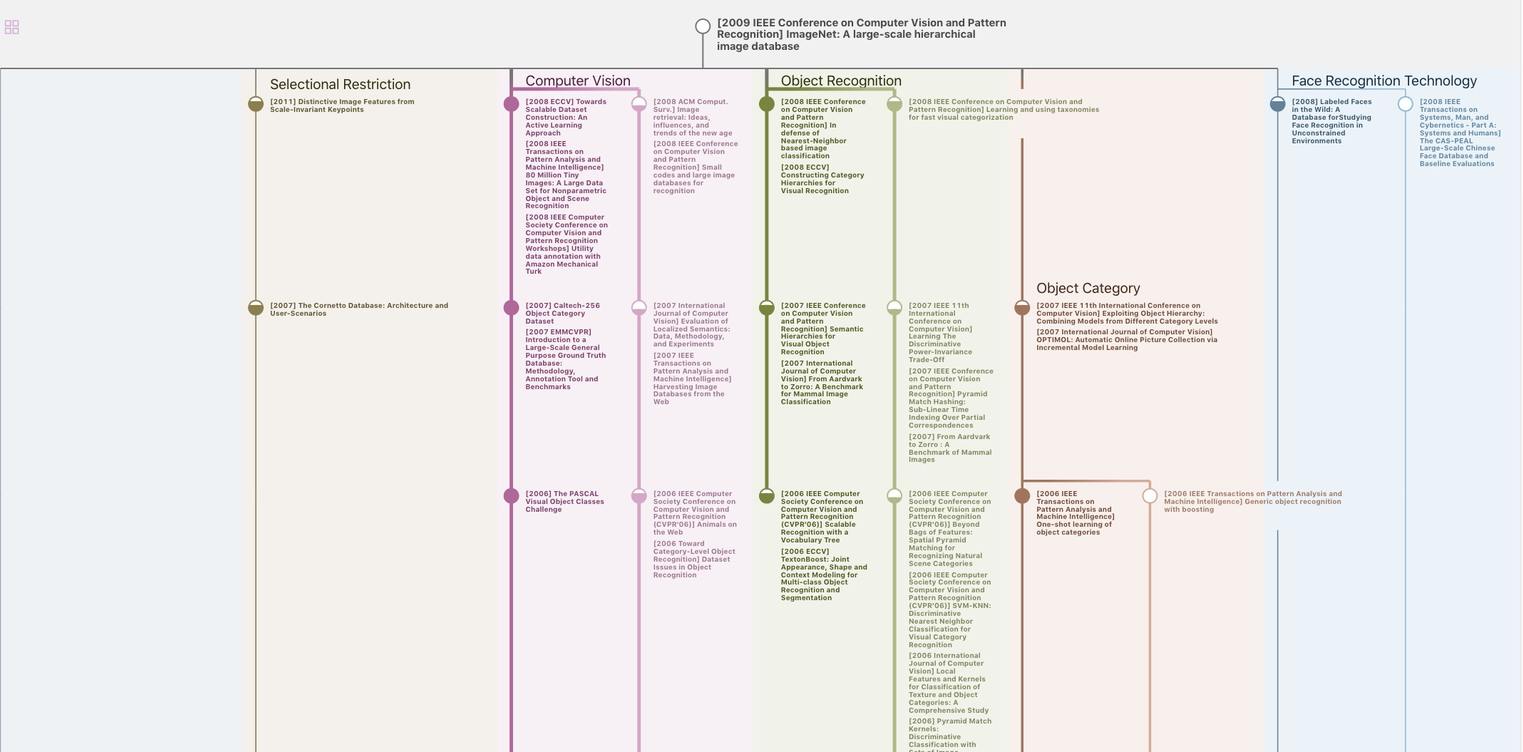
生成溯源树,研究论文发展脉络
Chat Paper
正在生成论文摘要