Modular Retrieval for Generalization and Interpretation
arxiv(2023)
摘要
New retrieval tasks have always been emerging, thus urging the development of new retrieval models. However, instantiating a retrieval model for each new retrieval task is resource-intensive and time-consuming, especially for a retrieval model that employs a large-scale pre-trained language model. To address this issue, we shift to a novel retrieval paradigm called modular retrieval, which aims to solve new retrieval tasks by instead composing multiple existing retrieval modules. Built upon the paradigm, we propose a retrieval model with modular prompt tuning named REMOP. It constructs retrieval modules subject to task attributes with deep prompt tuning, and yields retrieval models subject to tasks with module composition. We validate that, REMOP inherently with modularity not only has appealing generalizability and interpretability in preliminary explorations, but also achieves comparable performance to state-of-the-art retrieval models on a zero-shot retrieval benchmark.\footnote{Our code is available at \url{https://github.com/FreedomIntelligence/REMOP}}
更多查看译文
关键词
retrieval,generalization,interpretation
AI 理解论文
溯源树
样例
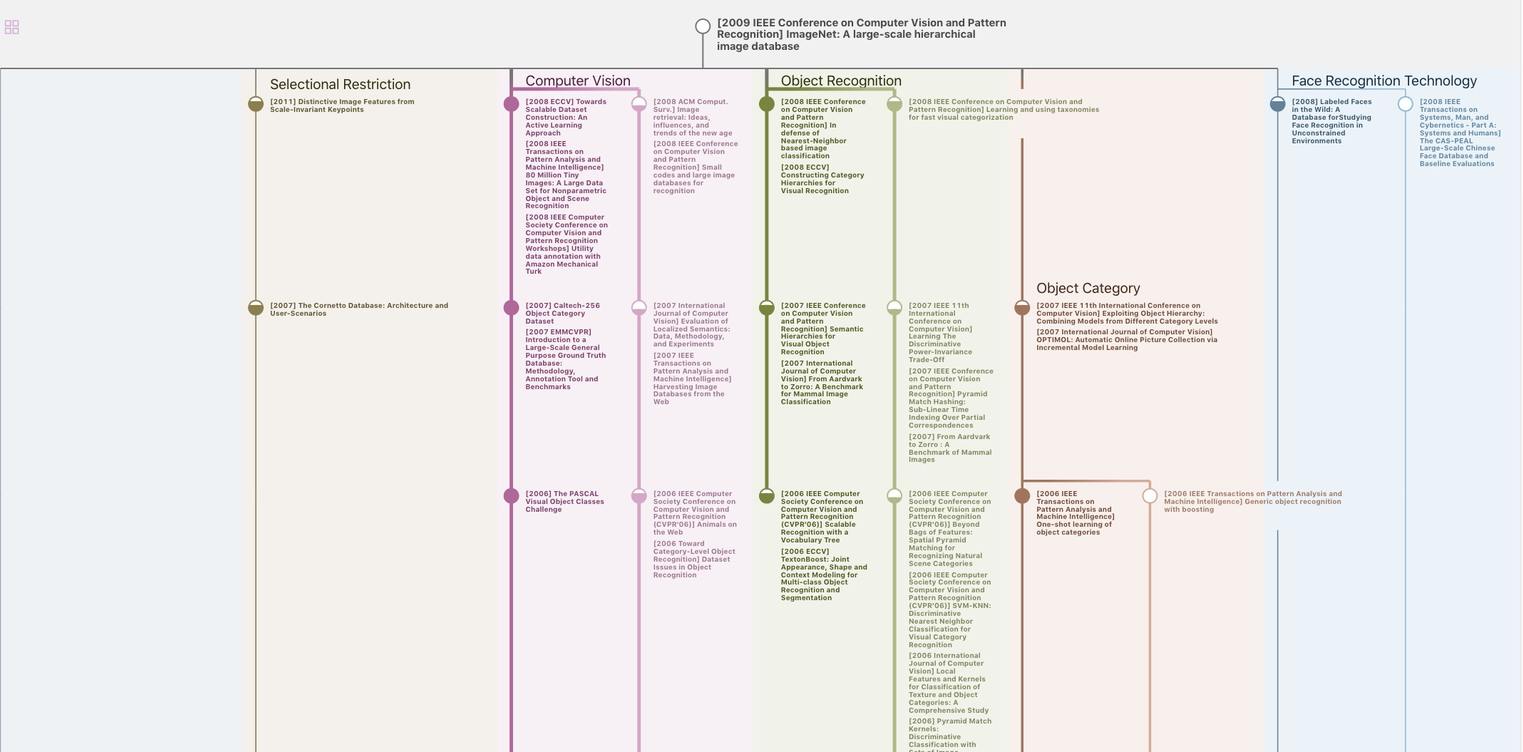
生成溯源树,研究论文发展脉络
Chat Paper
正在生成论文摘要