What Matters In The Structured Pruning of Generative Language Models?
ICLR 2023(2023)
摘要
Auto-regressive large language models such as GPT-3 require enormous computational resources to use, leading to huge financial cost and environmental impact. Structured pruning methods traditionally reduce resource usage, however, their application to and efficacy for generative language models is heavily under-explored. We analyze the effects of magnitude, random, and movement (Lagunas et al., 2021) pruning on MLP layers in GPT-like models. We find that movement can under-perform for these models while random pruning nearly matches the best methods. By examining neuron-level redundancy measures, we discover that movement does not select neurons based on how unique they are compared to other neurons, leaving behind excess redundancy. In view of this, we introduce Globally Unique Movement (GUM) to select neurons based on both uniqueness and sensitivity. We then discuss the roles of our techniques on different redundancy metrics through careful comparisons and ablations.
更多查看译文
关键词
Neural Network Pruning,Natural Language Generation
AI 理解论文
溯源树
样例
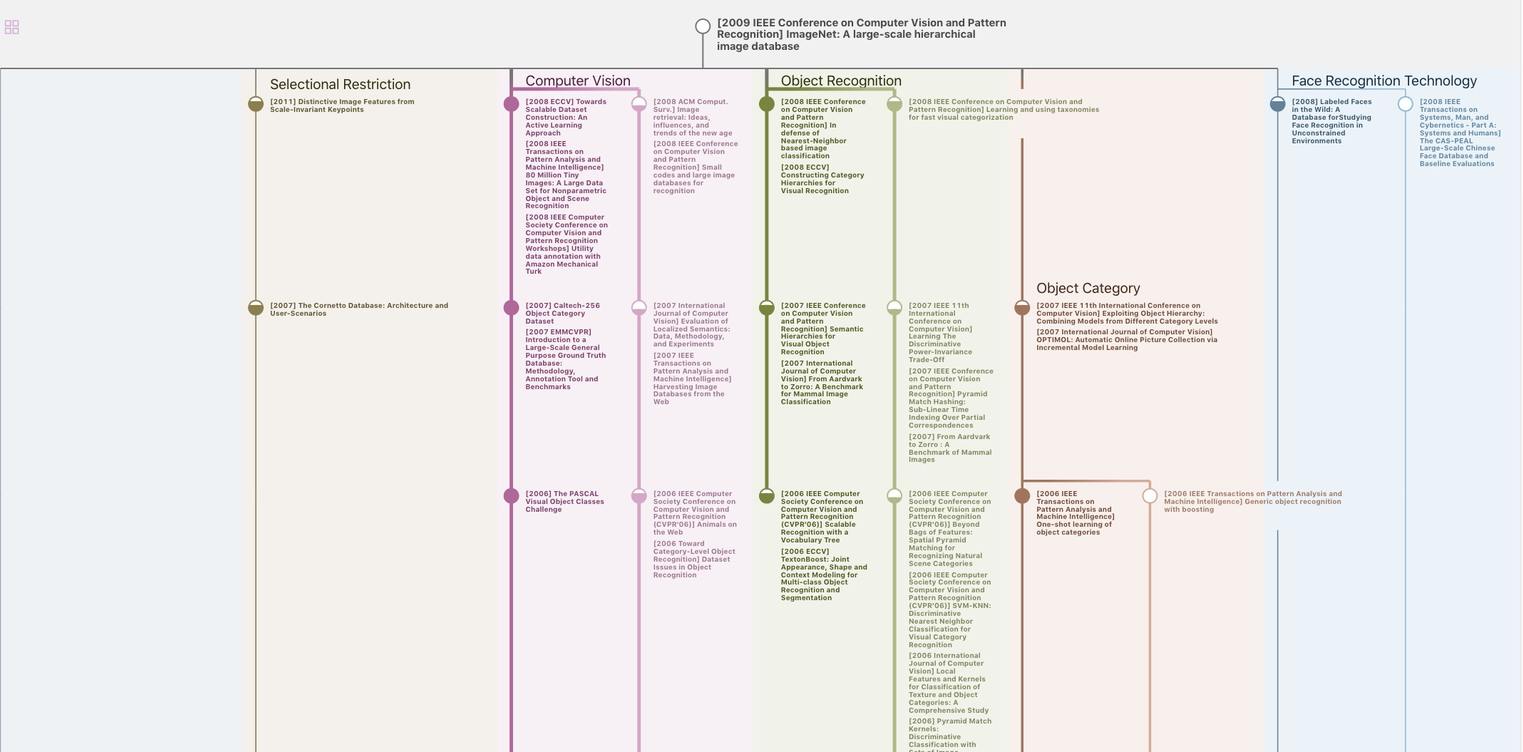
生成溯源树,研究论文发展脉络
Chat Paper
正在生成论文摘要