Skipped Nonsynaptic Backpropagation for Interval-valued Long-term Cognitive Networks.
Mexican International Conference on Artificial Intelligence (MICAI)(2022)
摘要
The recently published Interval-valued Long-term Cognitive Networks have shown promising results when reasoning under uncertainty conditions. In these recurrent neural networks, the interval weights are learned using a nonsynaptic backpropagation learning algorithm. Similar to traditional propagation-based algorithms, this variant might suffer from vanishing/exploding gradient issues. This paper proposes three skipped learning variants that do not use the backpropagation process to deliver the error signal to intermediate abstract layers (iterations in the recurrent neural network). The numerical simulations using 35 synthetic datasets confirm that the skipped variants work as well as the nonsynaptic backpropagation algorithm.
更多查看译文
关键词
nonsynaptic backpropagation,networks,interval-valued,long-term
AI 理解论文
溯源树
样例
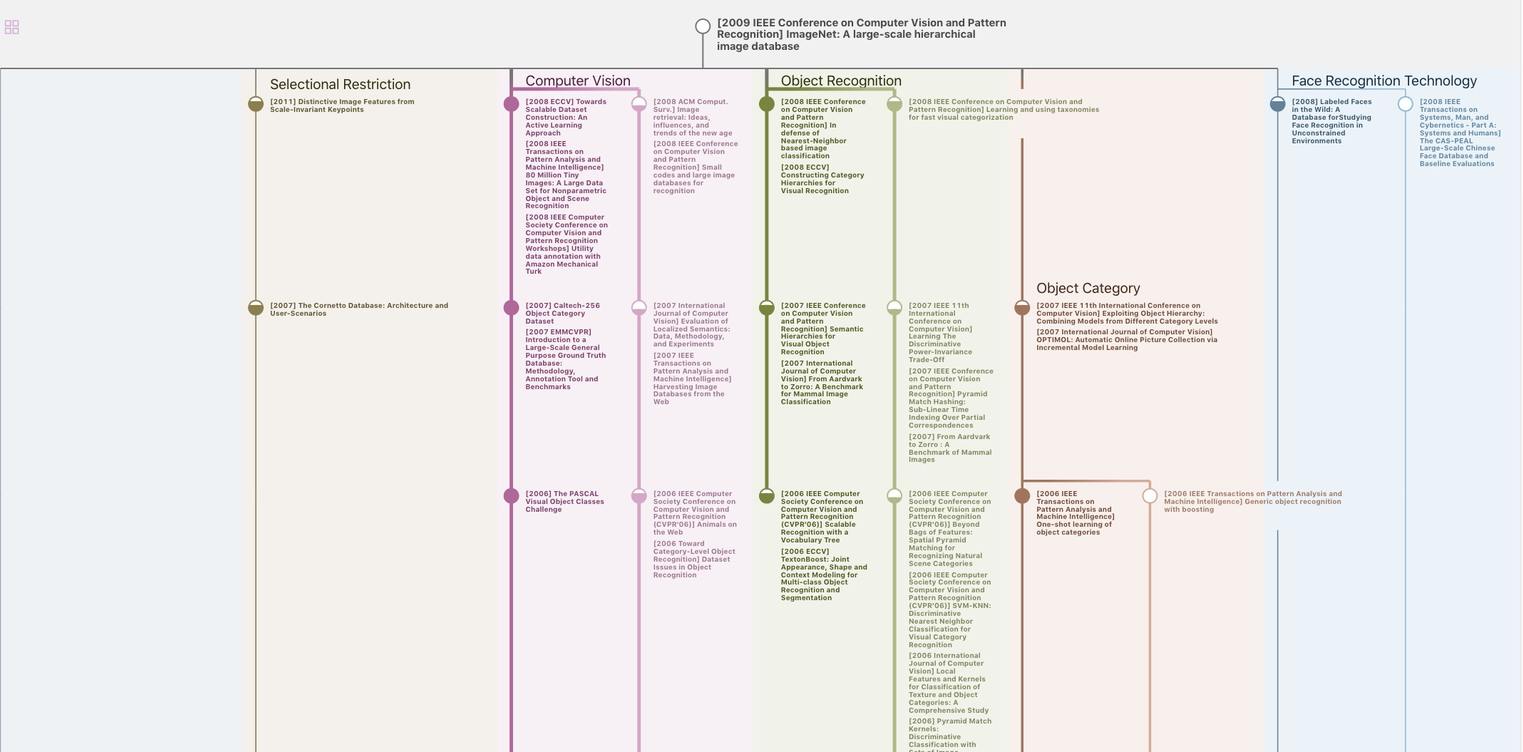
生成溯源树,研究论文发展脉络
Chat Paper
正在生成论文摘要