Towards Open-Domain Topic Classification
NAACL 2022: THE 2022 CONFERENCE OF THE NORTH AMERICAN CHAPTER OF THE ASSOCIATION FOR COMPUTATIONAL LINGUISTICS: HUMAN LANGUAGE TECHNOLOGIES: PROCEEDINGS OF THE DEMONSTRATIONS SESSION(2022)
摘要
We introduce an open-domain topic classification system that accepts user-defined taxonomy in real time. Users will be able to classify a text snippet with respect to any candidate labels they want, and get instant response from our web interface. To obtain such flexibility, we build the backend model in a zero-shot way. By training on a new dataset constructed from Wikipedia, our label-aware text classifier can effectively utilize implicit knowledge in the pretrained language model to handle labels it has never seen before. We evaluate our model across four datasets from various domains with different label sets. Experiments show that the model significantly improves over existing zero-shot baselines in open-domain scenarios, and performs competitively with weakly-supervised models trained on in-domain data.(12)
更多查看译文
关键词
classification,topic,open-domain
AI 理解论文
溯源树
样例
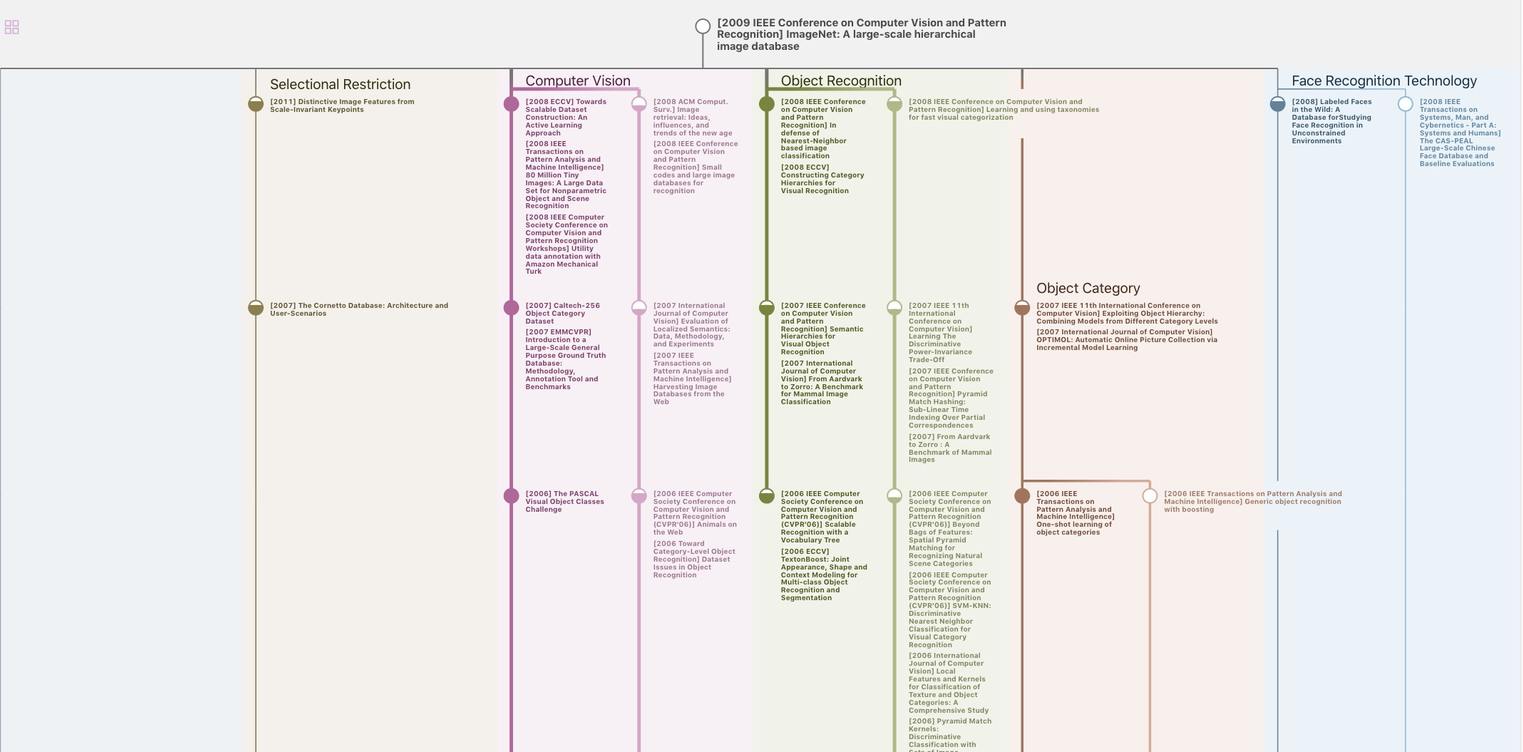
生成溯源树,研究论文发展脉络
Chat Paper
正在生成论文摘要