Multi-objective Hyperparameter Optimization with Performance Uncertainty.
OLA(2022)
摘要
The performance of any Machine Learning (ML) algorithm is impacted by the choice of its hyperparameters. As training and evaluating a ML algorithm is usually expensive, the hyperparameter optimization (HPO) method needs to be computationally efficient to be useful in practice. Most of the existing approaches on multi-objective HPO use evolutionary strategies and metamodel-based optimization. However, few methods have been developed to account for uncertainty in the performance measurements. This paper presents results on multi-objective hyperparameter optimization with uncertainty on the evaluation of ML algorithms. We combine the sampling strategy of Tree-structured Parzen Estimators (TPE) with the metamodel obtained after training a Gaussian Process Regression (GPR) with heterogeneous noise. Experimental results on three analytical test functions and three ML problems show the improvement over multi-objective TPE and GPR, achieved with respect to the hypervolume indicator.
更多查看译文
关键词
optimization,uncertainty,multi-objective
AI 理解论文
溯源树
样例
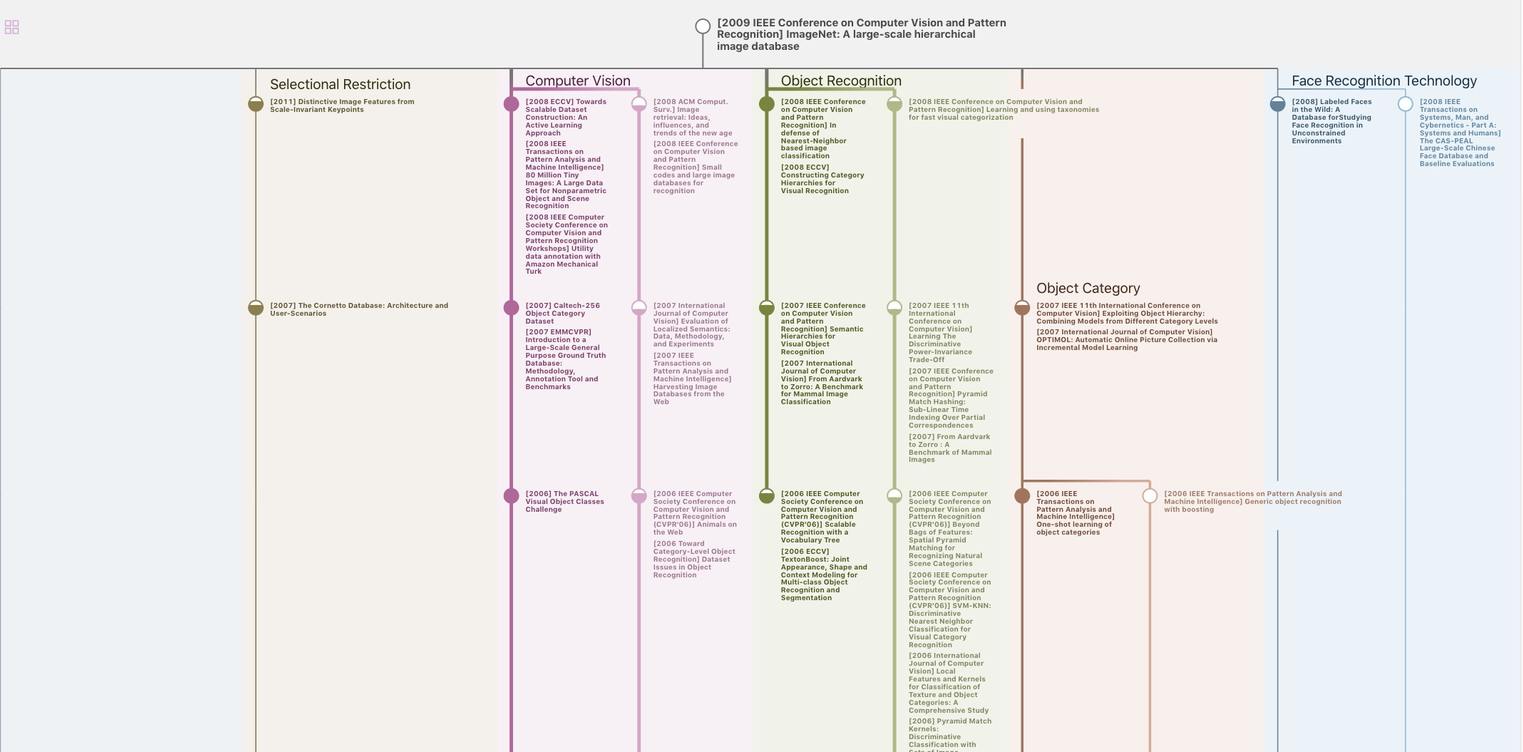
生成溯源树,研究论文发展脉络
Chat Paper
正在生成论文摘要